Using Generative AI to Enhance Cybersecurity through Predictive Threat Analysis
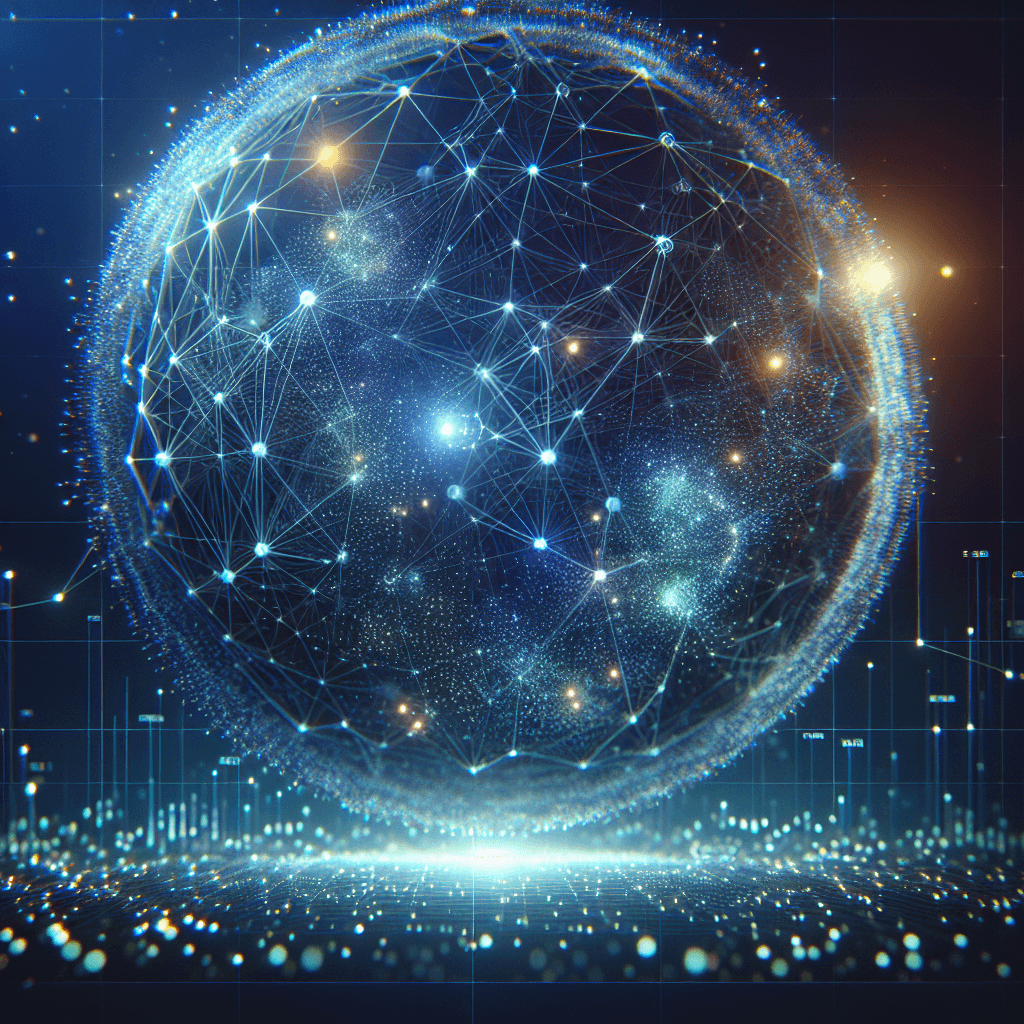
Using Generative AI to Enhance Cybersecurity through Predictive Threat Analysis
The rapidly evolving threat landscape of the digital world demands that cybersecurity measures are constantly updated and improved. One innovative approach gaining traction is the integration of Generative AI applications in predictive threat analysis. By leveraging machine learning algorithms and large datasets, Generative AI enables organizations to stay one step ahead of malicious actors. In this blog post, we will delve into the world of Generative AI-powered cybersecurity, exploring its potential benefits and limitations.
What is Predictive Threat Analysis?
Predictive threat analysis is a method used in cybersecurity that involves analyzing patterns and trends in threat intelligence data to forecast future attacks. This approach enables organizations to anticipate and prepare for potential security breaches before they occur. By identifying vulnerabilities and weaknesses in systems and networks, predictive threat analysis can help prevent successful attacks.
The Concept of Predictive Analytics
Predictive analytics is a statistical technique used to analyze historical data and make predictions about future events or outcomes. In the context of cybersecurity, predictive analytics involves analyzing patterns and trends in threat intelligence data to forecast potential security breaches. This approach allows organizations to identify vulnerabilities and weaknesses in their systems and networks before they can be exploited by malicious actors.
How Generative AI Enhances Predictive Threat Analysis
Generative AI applications play a crucial role in enhancing predictive threat analysis by:
- Analyzing vast amounts of data: Generative AI can process large datasets quickly and accurately, identifying patterns and anomalies that may indicate potential threats.
- Developing predictive models: By leveraging machine learning algorithms, Generative AI can develop predictive models that forecast the likelihood of future attacks based on historical data.
- Identifying unknown threats: Generative AI can recognize unknown threats by analyzing patterns in network traffic and system behavior.
Machine Learning Algorithms Used in Generative AI
Several machine learning algorithms are used in Generative AI applications, including:
- Supervised learning: Supervised learning involves training a model on labeled data to predict outcomes.
- Unsupervised learning: Unsupervised learning involves identifying patterns and anomalies in unlabeled data.
- Deep learning: Deep learning involves using neural networks to analyze complex data sets.
Benefits of Using Generative AI for Predictive Threat Analysis
The integration of Generative AI in predictive threat analysis offers several benefits, including:
- Improved threat detection accuracy: By leveraging machine learning algorithms and large datasets, Generative AI can detect threats more accurately than traditional methods.
- Enhanced predictive capabilities: Generative AI enables organizations to forecast potential attacks, allowing them to take proactive measures to prevent breaches.
- Increased efficiency: Generative AI automates the process of threat analysis, reducing manual effort and improving response times.
Case Study: Using Generative AI for Predictive Threat Analysis
One company used Generative AI to enhance its predictive threat analysis capabilities. The company’s cybersecurity team worked with a third-party provider to develop a custom Generative AI application that analyzed patterns in network traffic and system behavior. The application identified several potential threats, including a zero-day exploit that was not yet known by the company’s security team.
The company’s proactive response to these threats saved them from significant financial losses and reputational damage.
Challenges and Limitations
While Generative AI has shown significant promise in enhancing predictive threat analysis, there are several challenges and limitations to consider:
- Data quality issues: Poor-quality data can lead to inaccurate predictions and reduced effectiveness.
- Overfitting and bias: Generative AI models can be biased towards existing patterns and data, leading to overfitting and reduced accuracy.
- Explainability and transparency: It can be difficult to understand the decision-making process of Generative AI models.
Best Practices for Implementing Generative AI in Predictive Threat Analysis
To get the most out of Generative AI applications in predictive threat analysis, consider the following best practices:
- Use high-quality data: Ensure that the data used to train and validate Generative AI models is accurate and comprehensive.
- Regularly update and refine models: As new threats emerge, regularly update and refine Generative AI models to maintain their effectiveness.
- Monitor performance metrics: Track key performance indicators (KPIs) such as accuracy and recall to ensure that Generative AI applications are meeting expectations.
Conclusion
The integration of Generative AI in predictive threat analysis offers significant potential for enhancing cybersecurity. By leveraging machine learning algorithms and large datasets, organizations can stay ahead of malicious actors and improve their overall security posture. As this technology continues to evolve, it is essential to address the challenges and limitations associated with its use. With careful planning, implementation, and maintenance, Generative AI applications can play a critical role in protecting against cyber threats.
Frequently Asked Questions
1. What are the primary benefits of using Generative AI for predictive threat analysis?
Generative AI offers improved threat detection accuracy, enhanced predictive capabilities, and increased efficiency.
2. How does Generative AI work in predictive threat analysis?
Generative AI works by analyzing patterns and trends in threat intelligence data to forecast potential security breaches.
3. What are the challenges and limitations of using Generative AI for predictive threat analysis?
The challenges and limitations of using Generative AI include data quality issues, overfitting and bias, and explainability and transparency.
4. How can organizations get the most out of Generative AI applications in predictive threat analysis?
Organizations can get the most out of Generative AI applications by using high-quality data, regularly updating and refining models, and monitoring performance metrics.