The Impact of Generative AI on Data Science Research and Development
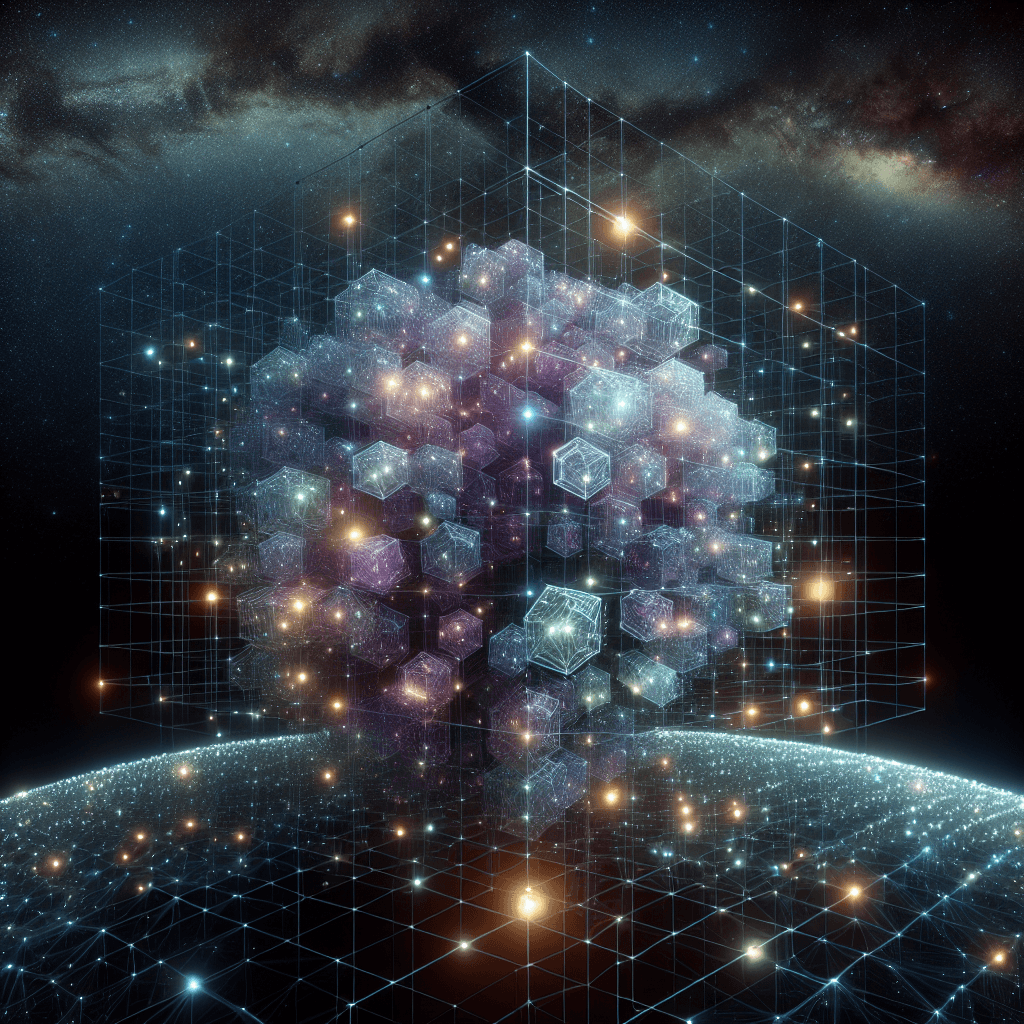
The Impact of Generative AI on Data Science Research and Development
Introduction
The advent of Generative AI (Generative Artificial Intelligence) has revolutionized the field of data science research and development. This technology has the potential to significantly impact various aspects of data science, including data analysis, machine learning, and data visualization. In this blog post, we will explore the main applications of Generative AI in data science research and development, its benefits, and challenges.
Understanding the Impact of Generative AI on Data Science Research Advancements
Generative AI has numerous applications in data science research, including:
- Data Generation: Generative AI can generate new, synthetic data that is similar to existing data. This can be useful for testing models on large datasets or generating new data for training machine learning models.
- Image and Video Generation: Generative AI can generate high-quality images and videos from text descriptions or other inputs. This has applications in fields such as computer vision, robotics, and entertainment.
- Natural Language Processing (NLP): Generative AI can be used to generate human-like text or responses to questions. This has applications in chatbots, virtual assistants, and content generation.
R&D Impact of Generative AI
The impact of Generative AI on R&D in data science is significant:
- Increased Efficiency: Generative AI can automate many tasks involved in data science research, such as data preprocessing and feature engineering.
- New Research Opportunities: Generative AI can enable new types of research, such as generating synthetic data for testing machine learning models or creating new datasets for training models.
- Improved Model Performance: Generative AI can be used to generate high-quality data that is more representative of the real world, leading to improved model performance.
Benefits of Generative AI
The benefits of Generative AI in data science research and development are numerous:
- Increased Productivity: Generative AI can automate many tasks involved in data science research, allowing researchers to focus on higher-level tasks.
- Improved Accuracy: Generative AI can generate high-quality data that is more representative of the real world, leading to improved model performance.
- New Research Opportunities: Generative AI can enable new types of research, such as generating synthetic data for testing machine learning models or creating new datasets for training models.
Challenges and Limitations
While Generative AI has many benefits, there are also several challenges and limitations:
- Data Quality Issues: The generated data may not be representative of the real world, leading to bias in machine learning models.
- Computational Resource Requirements: Training generative AI models can require significant computational resources, including high-performance hardware and large amounts of data.
- Explainability and Transparency: Generative AI models can be difficult to explain and interpret, making it challenging to understand how they arrive at their predictions.
Addressing Data Quality Issues
To address data quality issues associated with generative AI, researchers are exploring various techniques such as:
- Data augmentation: This involves artificially increasing the size of existing datasets by applying transformations or perturbations to the data.
- Data curation: This involves carefully selecting and annotating a subset of the generated data to ensure it is representative of the real world.
- Evaluation metrics: Researchers are developing new evaluation metrics that can accurately assess the quality of generated data.
Unlocking New Insights through Collaborative Research
Generative AI models are designed to work collaboratively with humans to unlock new insights and improve the accuracy of machine learning models. By integrating Generative AI into research workflows, scientists can focus on higher-level tasks while leveraging the power of automation for data analysis and feature engineering.
To achieve this collaboration, researchers are exploring various approaches such as:
- Human-AI hybrid models: These models combine human expertise with the power of Generative AI to generate high-quality data.
- Explainable AI: Researchers are developing techniques to explain and interpret the decisions made by Generative AI models, ensuring transparency and trust in the output.
Future Directions for Research
As Generative AI continues to evolve, future research directions will focus on addressing challenges such as data quality issues, computational resource requirements, and explainability and transparency. Some potential areas of exploration include:
- Developing more robust evaluation metrics: Researchers will continue to develop new evaluation metrics that can accurately assess the quality of generated data.
- Improving data augmentation techniques: Scientists will explore new techniques for augmenting existing datasets, such as active learning and transfer learning.
- Investigating the role of human-AI collaboration: Researchers will investigate the benefits and challenges of integrating Generative AI with human expertise to generate high-quality data.
Conclusion
Generative AI has the potential to revolutionize the field of data science research and development. By understanding its applications, benefits, and challenges, researchers can unlock new insights and improve the accuracy of machine learning models. As Generative AI continues to evolve, future research directions will focus on addressing key challenges such as data quality issues, computational resource requirements, and explainability and transparency.
Generative AI has numerous applications in data science research, including data generation, image and video generation, and natural language processing. It can automate tasks such as data preprocessing and feature engineering, enable new types of research, and improve model performance.
The impact of Generative AI on R&D in data science is significant, with potential benefits including increased efficiency, new research opportunities, and improved model performance. However, there are also challenges to be addressed, such as data quality issues, computational resource requirements, and explainability and transparency.
To address these challenges, researchers are exploring various techniques such as data augmentation, data curation, and evaluation metrics. Generative AI models can work collaboratively with humans to unlock new insights and improve the accuracy of machine learning models.
Future research directions will focus on developing more robust evaluation metrics, improving data augmentation techniques, and investigating the role of human-AI collaboration. By understanding the impact of Generative AI on data science research advancements, researchers can unlock new opportunities for R&D through generative AI models and unlock new insights through collaborative research.