Machine Learning for Policy Analysis
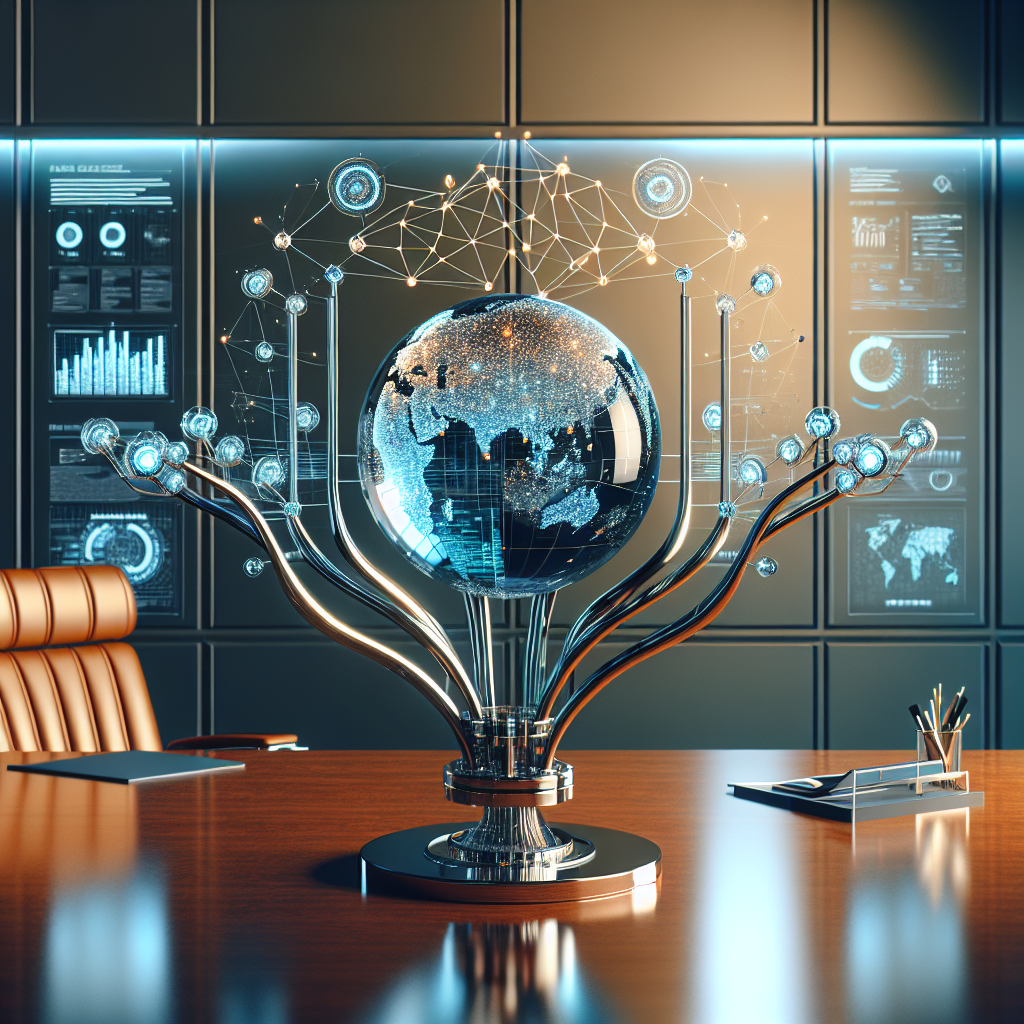
Machine Learning for Policy Analysis: A Comprehensive Guide
Introduction
In recent years, Machine Learning has emerged as a powerful tool in various fields, including policy analysis. The integration of Machine Learning with Policy Analysis has the potential to revolutionize the way policies are developed, implemented, and evaluated. By using machine learning to analyze and inform policy decisions, policymakers can make more informed decisions, leading to better outcomes for citizens. This blog post aims to provide an overview of the applications, benefits, and challenges of using Machine Learning for Policy Analysis, highlighting the role of machine learning in policy analysis and development.
What is Machine Learning for Policy Analysis?
Machine Learning for Policy Analysis involves the use of algorithms and statistical models to analyze large datasets and identify patterns that can inform policy decisions. This approach enables policymakers to make data-driven decisions, rather than relying on intuition or anecdotal evidence. The role of machine learning in policy analysis is to provide insights into complex social phenomena, predict outcomes, and evaluate the effectiveness of policies. By leveraging machine learning, policymakers can gain a deeper understanding of the issues they are trying to address and develop more effective solutions.
Applications of Machine Learning in Policy Analysis
Machine learning has a wide range of applications in policy analysis, including:
- Predictive modeling: Machine learning algorithms can be used to build predictive models that forecast the outcomes of different policy interventions. For example, policymakers can use machine learning to predict the impact of a new tax policy on economic growth.
- Data analysis: Machine learning can be used to analyze large datasets and identify patterns and trends that inform policy decisions. For instance, policymakers can use machine learning to analyze data on crime rates and develop more effective strategies for reducing crime.
- Policy evaluation: Machine learning can be used to evaluate the effectiveness of policies and identify areas for improvement. By analyzing data on policy outcomes, policymakers can determine which policies are working and which need to be revised or replaced.
Benefits of Machine Learning in Policy Analysis
The benefits of using machine learning in policy analysis include:
- Improved decision-making: Machine learning provides policymakers with accurate and reliable data, enabling them to make more informed decisions.
- Increased efficiency: Machine learning automates many tasks, freeing up policymakers to focus on higher-level strategic work.
- Enhanced transparency and accountability: Machine learning can be used to develop transparent and accountable models that provide clear explanations for policy decisions.
Challenges of Machine Learning in Policy Analysis
While machine learning has the potential to revolutionize policy analysis, there are also challenges to consider:
- Data quality: Machine learning requires high-quality data to produce accurate results. Poor data quality can lead to biased or inaccurate models.
- Model interpretability: Machine learning models can be complex and difficult to interpret, making it challenging for policymakers to understand the reasoning behind policy recommendations.
- Ethical considerations: Machine learning raises ethical concerns, such as bias and fairness. Policymakers must ensure that machine learning models are fair and unbiased.
Best Practices for Using Machine Learning in Policy Analysis
To get the most out of machine learning in policy analysis, policymakers should follow best practices, including:
- Using high-quality data: Machine learning algorithms require high-quality data to produce accurate results. Policymakers should ensure that their data is accurate, complete, and relevant.
- Selecting the right algorithm: Different machine learning algorithms are suited to different tasks. Policymakers should select the algorithm that best fits their needs and goals.
- Evaluating and refining models: Machine learning models should be continuously evaluated and refined to ensure that they are producing accurate results.
Case Studies of Machine Learning in Policy Analysis
There are many examples of machine learning being used effectively in policy analysis. For instance:
- The city of New York used machine learning to develop a predictive model that forecasted the likelihood of fires in different buildings. The model was used to target inspections and reduce the risk of fires.
- The state of California used machine learning to develop a model that predicted the likelihood of recidivism among prisoners. The model was used to identify high-risk individuals and provide them with targeted interventions.
Future Directions for Machine Learning in Policy Analysis
As machine learning continues to evolve, we can expect to see new and innovative applications in policy analysis. Some potential future directions include:
- Increased use of natural language processing: Natural language processing can be used to analyze large amounts of text data, such as policy documents and citizen feedback.
- Greater emphasis on transparency and accountability: As machine learning becomes more widespread, there will be a greater need for transparency and accountability in its use. This includes developing explainable models and providing clear information about how decisions are made.
- More focus on human-centered design: Machine learning should be designed with the needs of citizens in mind. This includes developing systems that are intuitive, user-friendly, and accessible to all.
The Role of Human Judgment in Machine Learning
While machine learning has the potential to revolutionize policy analysis, it is also important to recognize the role of human judgment. Machine learning models should be seen as tools that support decision-making, rather than replacing human judgment altogether. Policymakers should use machine learning models to inform their decisions, but also consider other factors, such as ethical and moral implications.
The Potential Risks of Machine Learning in Policy Analysis
There are also potential risks associated with using machine learning in policy analysis, including:
- Bias and discrimination: Machine learning models can perpetuate existing biases and discriminate against certain groups.
- Lack of transparency: Machine learning models can be complex and difficult to interpret, making it challenging for policymakers to understand the reasoning behind policy recommendations.
- Dependence on data quality: Machine learning models are only as good as the data they are trained on. Poor data quality can lead to biased or inaccurate models.
Mitigating the Risks of Machine Learning in Policy Analysis
To mitigate these risks, policymakers should:
- Ensure that machine learning models are fair and unbiased: This includes regularly auditing models for bias and taking steps to address any issues that arise.
- Develop transparent and explainable models: Policymakers should prioritize transparency and explainability when developing machine learning models.
- Invest in high-quality data: Policymakers should invest in high-quality data and ensure that it is accurate, complete, and relevant.
Conclusion
Machine learning has the potential to revolutionize policy analysis by providing policymakers with accurate and reliable data, increasing efficiency, and enhancing transparency and accountability. However, there are also challenges and risks associated with using machine learning in policy analysis, including data quality, model interpretability, and ethical considerations. By following best practices and mitigating these risks, policymakers can unlock the full potential of machine learning and develop more effective policies.