Utilizing AI Agents for Financial Data Analysis
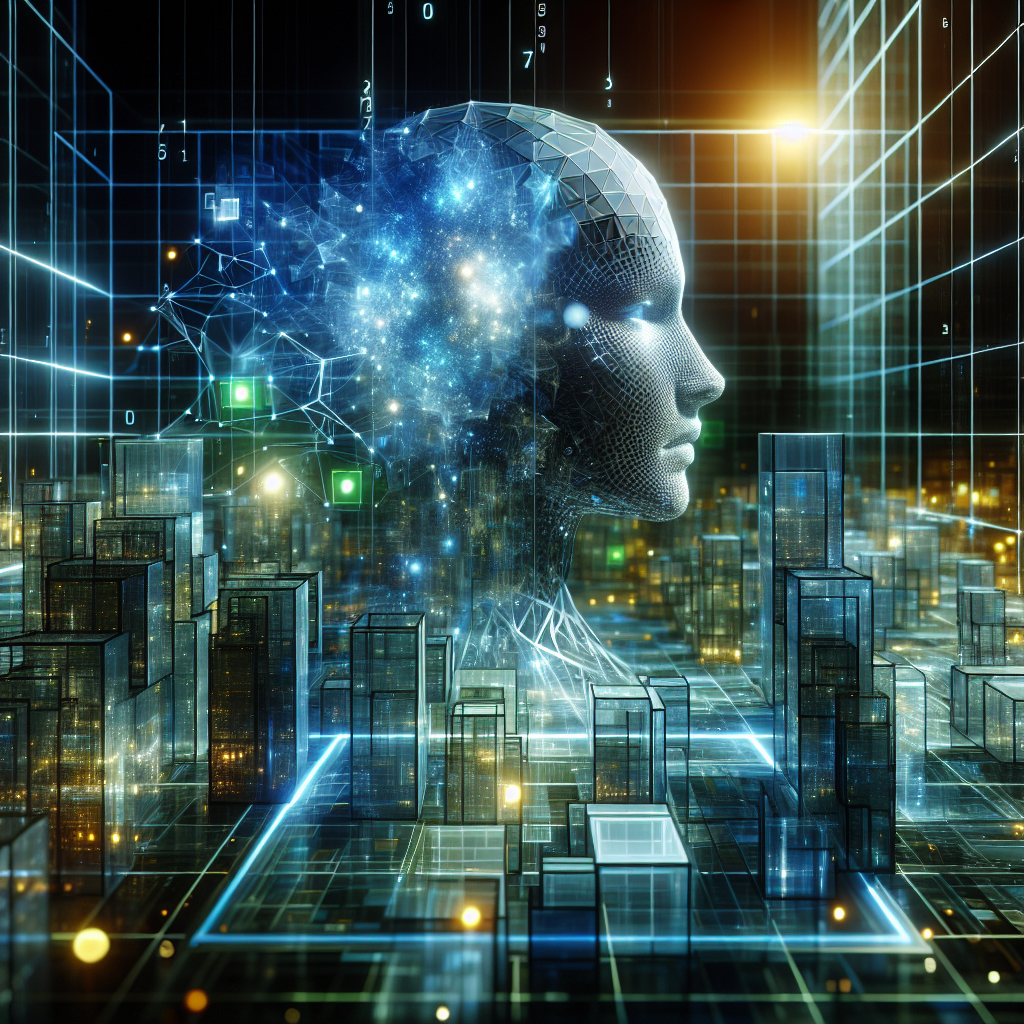
Utilizing AI Agents for Financial Data Analysis
In today’s fast-paced financial world, leveraging advanced technology to gain a competitive edge is crucial. AI agents for financial analysis are transforming how investors and analysts approach market data by providing real-time insights and sophisticated forecasting techniques. This blog post explores how these intelligent systems optimize investment strategies, enhance economic forecasting, and deliver valuable investment insights.
Introduction
The integration of artificial intelligence (AI) in finance has been revolutionary. From algorithmic trading to risk management, AI agents are reshaping the industry. By analyzing vast amounts of data at unprecedented speeds, these AI systems offer detailed investment insights and accurate economic forecasting, enabling better decision-making processes.
As financial markets grow increasingly complex, the demand for precise and timely information rises. AI agents step in, offering a nuanced understanding of market dynamics that traditional methods often miss. Whether you’re an investor seeking to maximize returns or a financial analyst aiming to predict economic trends, utilizing AI can significantly enhance your capabilities.
The Power of AI Agents
1. Understanding AI Agents for Financial Analysis
AI agents are sophisticated algorithms designed to simulate human-like reasoning and decision-making in finance. These systems utilize machine learning techniques to interpret vast datasets, identifying patterns and correlations that might be invisible to the naked eye. By automating data analysis tasks, AI agents allow financial professionals to focus on strategic decisions rather than manual data crunching.
2. Key Benefits
- Efficiency: AI agents can process enormous volumes of data far quicker than human analysts, providing timely insights.
- Accuracy: Through advanced algorithms and continuous learning, AI systems enhance the precision of financial forecasts.
- Scalability: These tools are capable of handling large datasets without compromising performance, making them ideal for global markets.
Applications in Investment Insights
1. Predictive Analytics
Predictive analytics involves using historical data to forecast future events. AI agents excel at this by applying machine learning models to predict stock price movements or market trends. This capability is invaluable for investors looking to make informed decisions based on potential future scenarios. As Bloomberg reports, machine learning models provide predictive analytics that guide portfolio management.
Example: Consider a hedge fund using an AI model to analyze sentiment from social media and news articles. By detecting shifts in public opinion about a particular stock, the AI can predict short-term price movements, allowing the fund to adjust its positions proactively.
2. Risk Assessment
AI systems can evaluate the risk associated with various investment opportunities by analyzing past performance and current market conditions. This helps in constructing diversified portfolios that align with an investor’s risk tolerance, ultimately optimizing returns while minimizing potential losses.
Example: An AI-powered tool could assess geopolitical risks by monitoring international news feeds and social media discussions. By quantifying these factors’ potential impact on asset prices, the tool provides investors with a clear picture of associated risks, supporting better-informed investment choices.
3. Portfolio Optimization
By continuously monitoring market changes, AI agents suggest real-time adjustments to investment portfolios. They analyze diverse data points, offering insights into which assets to hold or divest, thereby optimizing portfolio performance—a strategy embraced by leading financial institutions like J.P. Morgan and Goldman Sachs.
Example: A robo-advisor platform uses an AI model to dynamically rebalance a client’s portfolio based on market volatility indices and macroeconomic indicators. This ensures that the investment strategy remains aligned with the investor’s goals, even as market conditions change.
Enhancing Economic Forecasting with AI Agents
AI agents are revolutionizing economic forecasting by integrating multiple datasets to create comprehensive models. This integration allows for a detailed analysis of global economic trends, assisting policymakers and businesses in making informed decisions.
1. Scenario Analysis
AI systems perform scenario analysis by simulating various economic conditions. This enables analysts to anticipate potential market shifts and prepare accordingly. By modeling different scenarios—such as changes in interest rates or inflation—a company can strategize effectively for future challenges.
Example: A multinational corporation might use AI-driven scenario planning to understand how tariffs could impact its supply chain. The AI models simulate various tariff regimes, allowing the company to devise contingency plans that minimize disruptions and costs.
2. Demand Forecasting
AI agents analyze historical sales data alongside economic indicators to forecast demand for products or services. This insight helps businesses manage inventory levels efficiently, ensuring they meet customer needs without overstocking.
Example: An e-commerce giant leverages AI tools to predict seasonal spikes in product demand based on past purchasing trends and current market conditions. By optimizing stock levels, the company reduces storage costs while enhancing customer satisfaction through timely deliveries.
Case Studies of AI Integration
1. Bloomberg’s AI-Driven Platforms
Bloomberg has integrated AI into its data analytics platforms, providing clients with enhanced tools for market analysis. Their AI models process real-time news and financial data to deliver actionable insights swiftly, empowering investors to make informed decisions rapidly.
Outcome: Clients report improved trading outcomes due to the rapid adaptation of their strategies based on Bloomberg’s AI-generated forecasts.
2. J.P. Morgan’s COIN Program
J.P. Morgan developed COIN (Contract Intelligence), an AI tool that reviews legal documents in seconds—a task that previously took lawyers thousands of hours. This integration significantly enhances efficiency and accuracy in transaction processing.
Outcome: The program has saved the firm millions by reducing time spent on document review, allowing teams to focus on more strategic tasks.
3. Goldman Sachs’ Marcus Platform
Goldman Sachs launched Marcus, an AI-driven online lending platform that uses machine learning algorithms to assess creditworthiness quickly. This innovation provides personalized loan offers based on a comprehensive analysis of applicant data.
Outcome: The platform has attracted millions of users by offering competitive rates and simplifying the borrowing process through streamlined assessments.
Challenges and Future Prospects
Despite the advantages, integrating AI into financial analysis presents challenges. Data privacy concerns and regulatory compliance issues require careful management. Additionally, there’s a need for ongoing education to ensure that financial professionals can effectively leverage these technologies.
Future Prospects: The future of AI in finance is promising, with potential advancements such as enhanced predictive accuracy and deeper integration across financial services. As machine learning models become more sophisticated, they will offer even greater insights, driving innovation and efficiency in the industry.
Conclusion
AI agents for financial analysis are transforming the landscape of finance by providing unparalleled investment insights and economic forecasting capabilities. As these technologies continue to evolve, they will play an increasingly critical role in shaping the future of the industry. Embracing AI not only enhances decision-making but also drives innovation, ensuring that businesses and investors remain competitive in a rapidly changing financial world.
By integrating advanced algorithms and machine learning models, AI agents enable more informed investment decisions, optimizing portfolio management and risk assessment. As leading financial entities like Bloomberg, J.P. Morgan, and Goldman Sachs continue to leverage these technologies, the potential for growth and innovation in finance is limitless.