Using AI in Agriculture Forecasting
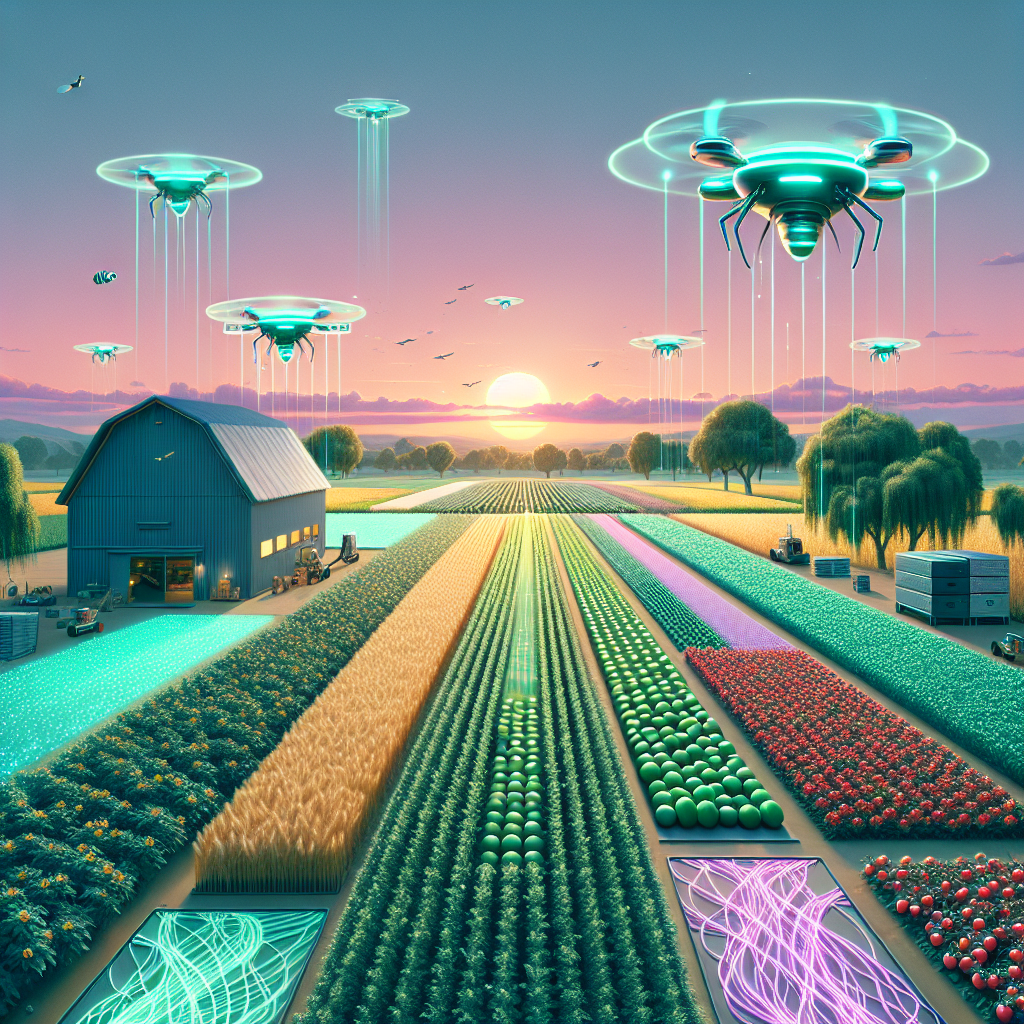
Using AI in Agriculture Forecasting: Enhancing Efficiency and Yield
In the ever-evolving landscape of agriculture, technology is driving sustainable food production while adapting to climate change. One area seeing significant advancements is agriculture forecasting, particularly through the integration of Artificial Intelligence (AI). These AI-driven systems are revolutionizing how farmers manage their operations by optimizing resource allocation and increasing crop yields. By leveraging AI in areas like weather prediction, farmers can make informed decisions that enhance productivity and resilience against adverse weather conditions.
Understanding Agriculture Forecasting
What is Agriculture Forecasting?
Agriculture forecasting involves predicting future trends related to agricultural activities, including crop growth cycles, livestock production, and market demands. These predictions are crucial for optimizing operations, managing resources efficiently, and making strategic business decisions. Accurate forecasts can help farmers anticipate changes in weather patterns, market fluctuations, and consumer behavior, allowing them to adapt their strategies proactively.
The Role of AI in Agriculture Forecasting
AI in agriculture forecasting utilizes advanced data analytics, machine learning algorithms, and predictive modeling techniques to provide accurate forecasts based on historical and real-time data. This includes integrating vast amounts of information from various sources such as satellite imagery, soil sensors, market trends, economic indicators, and consumer behavior patterns. By processing this data, AI can identify complex relationships and hidden insights that traditional methods might overlook.
Weather Prediction with AI
How AI Enhances Weather Prediction
AI in weather prediction is transforming the accuracy and reliability of forecasts. Traditional methods often rely on limited data points and human interpretation, which can be prone to errors. In contrast, AI-powered systems process vast amounts of data from multiple sources at an unprecedented scale and speed, identifying subtle patterns that may be missed by human analysts. Machine learning algorithms can analyze complex datasets, including historical weather records, satellite imagery, and atmospheric models, to generate highly accurate predictions.
Benefits of Accurate Weather Prediction
Accurate weather prediction is essential for farmers to make informed decisions about planting, irrigation, and harvesting. With AI in agriculture forecasting, farmers can anticipate adverse weather conditions, reduce crop losses, and optimize resource use. For example, by predicting drought periods, farmers can plan their irrigation schedules more efficiently, ensuring that crops receive the necessary water without wasting resources.
Predictive Modeling for Crop Yields
How Predictive Modeling Works
Predictive modeling for crop yields uses historical data and machine learning algorithms to forecast future crop performance. This approach considers various factors, including weather patterns, soil health, water availability, and farming practices. By analyzing these variables, predictive models can estimate the potential yield of different crops under specific conditions, helping farmers make informed decisions about which crops to plant and how to manage them.
Benefits of Predictive Modeling
By leveraging predictive modeling, farmers can make informed decisions about resource allocation, such as water and fertilizer usage. This not only enhances crop yields but also reduces waste and environmental impact. For instance, predictive models can suggest optimal planting dates based on weather forecasts, ensuring that crops are planted at the most favorable time to maximize their growth potential.
Irrigation Management with AI
Optimizing Water Usage
Irrigation management is a critical aspect of farming that can significantly impact crop yields and profitability. Traditional irrigation methods often result in water waste due to inefficient scheduling or inadequate monitoring. AI-powered irrigation systems, however, use data from weather forecasts, soil sensors, and other sources to optimize water usage. These systems can adjust irrigation schedules in real-time based on current conditions, ensuring that crops receive the right amount of water at the right time.
Benefits of AI-Driven Irrigation Management
The benefits of AI-driven irrigation management are numerous. By reducing water waste, these systems help conserve a valuable resource while also lowering operational costs for farmers. Additionally, precise water application can improve crop quality and yield, contributing to increased profitability. For example, AI-powered irrigation systems can detect signs of stress in plants and adjust watering schedules accordingly, ensuring that crops remain healthy and productive.
Case Studies: Successful Implementation of AI in Agriculture Forecasting
John Deere’s Precision Ag Solutions
John Deere is a leading provider of agricultural equipment and technology solutions. Their precision ag platform integrates various technologies, including AI, to help farmers optimize their operations. One key feature is the ability to predict weather patterns and soil moisture levels, enabling farmers to make informed decisions about planting and irrigation. By leveraging data from satellite imagery, weather forecasts, and in-field sensors, John Deere’s systems can provide accurate predictions that help farmers improve crop yields while reducing resource waste.
Syngenta’s Digital Farming Solutions
Syngenta is a global leader in agriculture with a focus on innovation and sustainability. Their digital farming solutions utilize AI to optimize crop management practices. One example is their FieldScripts platform, which uses data from various sources, including weather forecasts, soil health assessments, and market trends, to generate personalized recommendations for farmers. By providing actionable insights based on real-time data, Syngenta’s systems help farmers make informed decisions about crop selection, planting dates, and resource management.
Climate Corporation by Bayer
Climate Corporation, now part of Bayer, provides advanced analytics and decision support tools for farmers. Their AI-powered solutions leverage historical weather data, soil health assessments, and other sources to generate accurate forecasts and recommendations. For example, their FieldView platform can predict crop yields based on various factors, helping farmers optimize resource allocation and improve profitability. By providing farmers with access to timely and accurate information, Climate Corporation’s systems help them make informed decisions that enhance productivity and sustainability.
Challenges and Considerations
Data Quality and Availability
One of the key challenges in implementing AI in agriculture forecasting is ensuring data quality and availability. Accurate predictions depend on high-quality data from reliable sources, which can be difficult to obtain in some regions. Farmers may need to invest in sensors and other monitoring tools to collect data, and they should work with agricultural experts to ensure that the data being used is accurate and relevant.
Technical Expertise
Another challenge is the technical expertise required to implement and manage AI systems effectively. While many platforms offer user-friendly interfaces, farmers may still need to have a basic understanding of how these systems work and how to interpret the information they provide. Collaborating with agricultural experts or data analysts can help farmers overcome this challenge and make the most of their AI investments.
Cost Considerations
Cost is another important consideration when it comes to implementing AI in agriculture forecasting. While there are many platforms available, some may require significant upfront investments or ongoing subscription fees. Farmers should carefully evaluate the costs and benefits of different options and choose solutions that fit within their budget and meet their specific needs.
Conclusion
The integration of Artificial Intelligence (AI) in agriculture forecasting is transforming how farmers manage their operations, optimize resource allocation, and enhance productivity. By leveraging advanced data analytics and predictive modeling techniques, AI systems can provide accurate forecasts that help farmers make informed decisions about planting, irrigation, and other critical activities. While there are challenges to overcome, the benefits of AI-powered agriculture forecasting are significant, including increased crop yields, reduced resource waste, and improved profitability. As technology continues to evolve, we can expect to see even more innovative applications of AI in the agricultural sector, contributing to a more sustainable and efficient food production system.