Unlocking Vector Databases with AI Tools
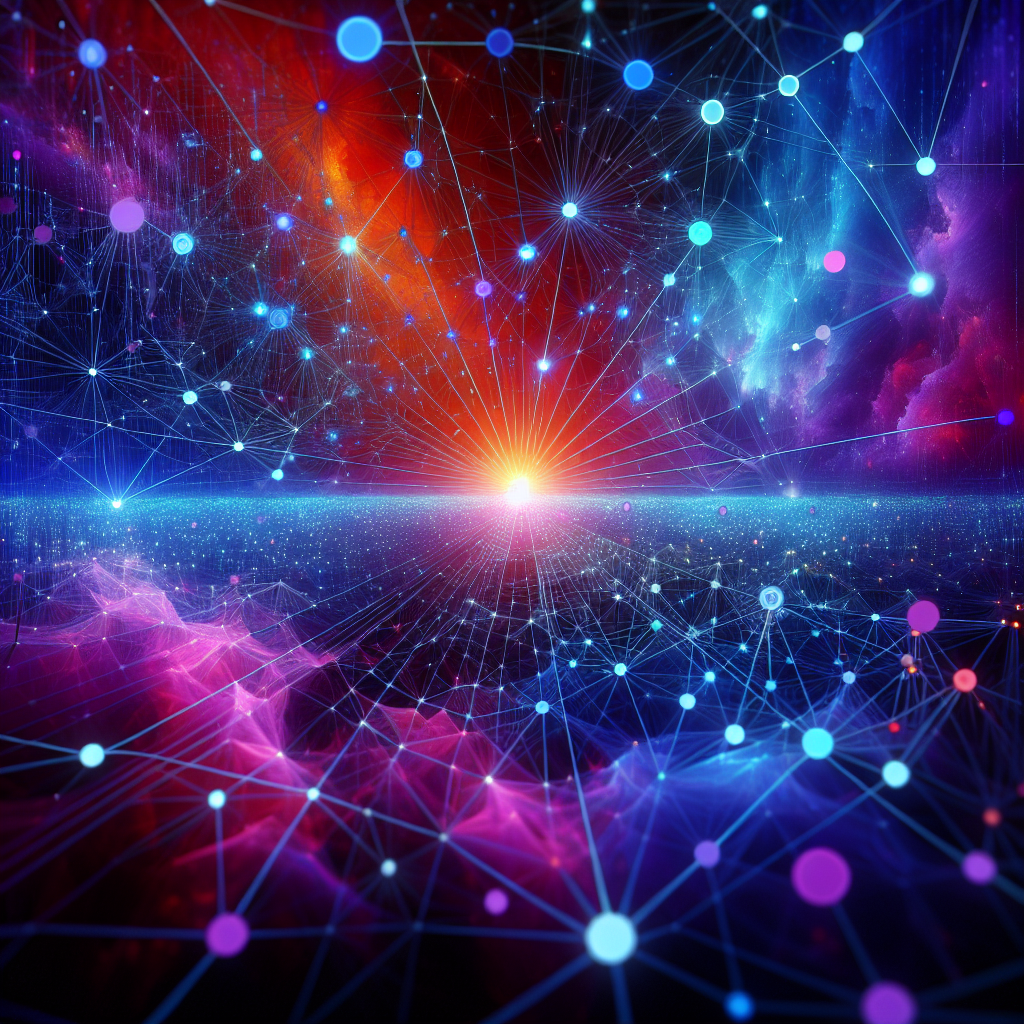
Unlocking Vector Databases with AI Tools
Introduction
In today’s fast-paced digital world, traditional databases are increasingly being enhanced—or sometimes replaced—by advanced systems designed to handle intricate queries and vast data sets efficiently. Vector databases have emerged as a transformative technology in this landscape, playing an essential role in applications such as recommendation engines, content search, and natural language processing. These powerful systems leverage artificial intelligence tools to significantly boost their capabilities, providing features like semantic search optimization and AI-powered data retrieval.
The marriage of machine learning with database technologies has revolutionized our interaction with data by introducing advanced algorithms and models that allow vector databases to grasp the semantics behind information rather than merely matching keywords. This shift enables a more intuitive approach to retrieving relevant data, enhancing user experiences across diverse applications.
In this blog post, we’ll delve into how AI tools are transforming vector databases, spotlighting the contributions of leading entities like OpenAI, Google Cloud, and Apache Milvus in advancing this technology. Whether you’re an IT professional, a business owner, or simply curious about the future of data management, understanding these developments is crucial.
The Role of AI in Vector Databases
Understanding Vector Databases
Vector databases store data as vectors—multi-dimensional arrays that represent complex data points like text, images, and audio. These databases are uniquely designed to manage unstructured data efficiently by leveraging machine learning models to capture the nuances of this information. Unlike traditional relational databases that focus on structured data, vector databases excel in handling diverse datasets, offering more flexibility and power in analyzing large-scale, varied data.
How Can AI Enhance the Efficiency of Managing Vector Databases?
Artificial intelligence tools play a pivotal role in enhancing vector database performance through various mechanisms:
Query Optimization: AI algorithms help optimize query processing by understanding user intent and context. This ensures faster and more accurate results compared to keyword-based searches, which often miss nuanced queries.
Efficient Data Indexing: Machine learning models assist in organizing data efficiently, improving retrieval speed. By analyzing patterns within the data, these models can pre-index likely queries, reducing latency during search operations.
Anomaly Detection: AI can identify irregularities or anomalies within the data, enhancing database security and reliability. This is especially critical in industries like finance and healthcare, where data integrity is paramount.
Scalability: With AI integration, vector databases can scale more effectively to accommodate growing datasets without a proportional increase in resource consumption. Machine learning algorithms optimize storage patterns and query paths, allowing for seamless scaling.
Understanding the Role of AI in Optimizing Vector Database Queries
AI transforms how queries are processed by embedding contextual understanding into search mechanisms. Traditional databases often rely on exact matches or basic syntactic parsing to execute queries, which can be limiting. In contrast, AI-driven vector databases use models like natural language processing (NLP) and deep learning to interpret the semantic meaning behind user inputs.
This capability allows for more nuanced data retrieval—imagine searching for “financial risk management techniques” and retrieving related but non-verbatim documents discussing derivative hedging strategies. The AI’s understanding of context ensures that users receive relevant results, enhancing both efficiency and satisfaction.
Case Studies: Leading Innovations
OpenAI: Pioneering Semantic Search Optimization
OpenAI has been at the forefront of semantic search optimization with its advanced language models like GPT-3. These tools understand complex queries by processing vast amounts of data to recognize patterns and relationships that might not be immediately obvious. For instance, a user searching for “sustainable agriculture practices” could receive insights into crop rotation techniques, organic farming methods, or precision agriculture technologies—all thanks to the semantic understanding embedded in OpenAI’s models.
Google Cloud: Facilitating AI-Powered Data Retrieval
Google Cloud supports businesses in integrating machine learning with vector databases through platforms like TensorFlow and Vertex AI. These tools enable efficient deployment of AI models that enhance data retrieval processes. For example, companies can use these technologies to develop recommendation engines tailored to user behavior, providing personalized content suggestions or product recommendations based on historical interaction data.
Apache Milvus: Advancing Machine Learning Integration
Apache Milvus is an open-source vector database designed for scalability and machine learning integration. It offers robust tools supporting high-performance search and analytics on vector data. By facilitating seamless integration with existing ML frameworks, Apache Milvus empowers organizations to deploy sophisticated models that enhance their data processing capabilities, whether it’s in image recognition or natural language understanding tasks.
Practical Applications Across Industries
E-commerce: Personalized Shopping Experiences
In the e-commerce sector, AI-enhanced vector databases enable highly personalized shopping experiences. By analyzing user behavior and preferences, businesses can offer tailored product recommendations that increase conversion rates and customer satisfaction.
Healthcare: Enhanced Diagnostics and Research
Healthcare providers leverage AI-driven vector databases for improved diagnostics and research capabilities. These systems can quickly sift through vast amounts of medical data to identify patterns or anomalies, aiding in early disease detection and personalized treatment plans.
Media and Entertainment: Content Discovery and Curation
Media companies utilize vector databases to enhance content discovery and curation. By understanding user preferences and viewing habits, these platforms can recommend movies, music, or articles that align with individual tastes, increasing engagement and retention.
Challenges and Future Directions
While the integration of AI with vector databases offers numerous advantages, it also presents challenges:
Data Privacy and Security: As more sensitive data is processed through these systems, ensuring robust security measures to protect user privacy becomes imperative.
Infrastructure Requirements: Implementing AI-enhanced vector databases can demand significant infrastructure investments, which might be challenging for smaller organizations.
Skill Gap: The complexity of managing AI-driven systems requires specialized skills and knowledge, necessitating ongoing education and training for IT professionals.
Despite these challenges, the future of AI in vector databases is promising. Continued advancements in machine learning models and computational power will likely lead to even more sophisticated capabilities, further revolutionizing data management across industries.
Conclusion
AI tools are unlocking new possibilities in the realm of vector databases by offering unparalleled efficiency and accuracy in data management. By leveraging technologies from leading entities like OpenAI, Google Cloud, and Apache Milvus, organizations can optimize their database queries, enhance user experiences, and gain deeper insights into their data.
As AI continues to evolve, its integration with vector databases will only deepen, driving innovation across industries. Embracing these advancements is crucial for staying competitive in a rapidly changing digital landscape.
Frequently Asked Questions
1. What are vector databases?
Vector databases store data as vectors—multi-dimensional arrays that represent complex data points like text, images, and audio. They efficiently handle unstructured data by leveraging machine learning models to capture the nuances of this information.
2. How do AI tools enhance vector database performance?
AI tools enhance vector database performance through query optimization, efficient data indexing, anomaly detection, and scalability. These capabilities improve the speed and accuracy of data retrieval, making databases more responsive and reliable.
3. What role does OpenAI play in semantic search optimization?
OpenAI utilizes models like GPT-3 to understand complex queries and retrieve information with high precision. Their tools improve the relevance of search results by comprehending natural language inputs, crucial for applications requiring accurate data retrieval.
4. How does Google Cloud support AI-powered data retrieval?
Google Cloud provides services that integrate machine learning with vector databases through platforms like TensorFlow and Vertex AI. These tools enable efficient deployment of AI models that enhance data retrieval processes, allowing businesses to develop sophisticated recommendation engines.
5. What is Apache Milvus’s contribution to machine learning integration?
Apache Milvus is an open-source vector database designed for scalability and machine learning integration. It offers robust tools supporting high-performance search and analytics on vector data, facilitating seamless integration with existing ML frameworks.