Data Scientists – Navigating the Generative AI Landscape
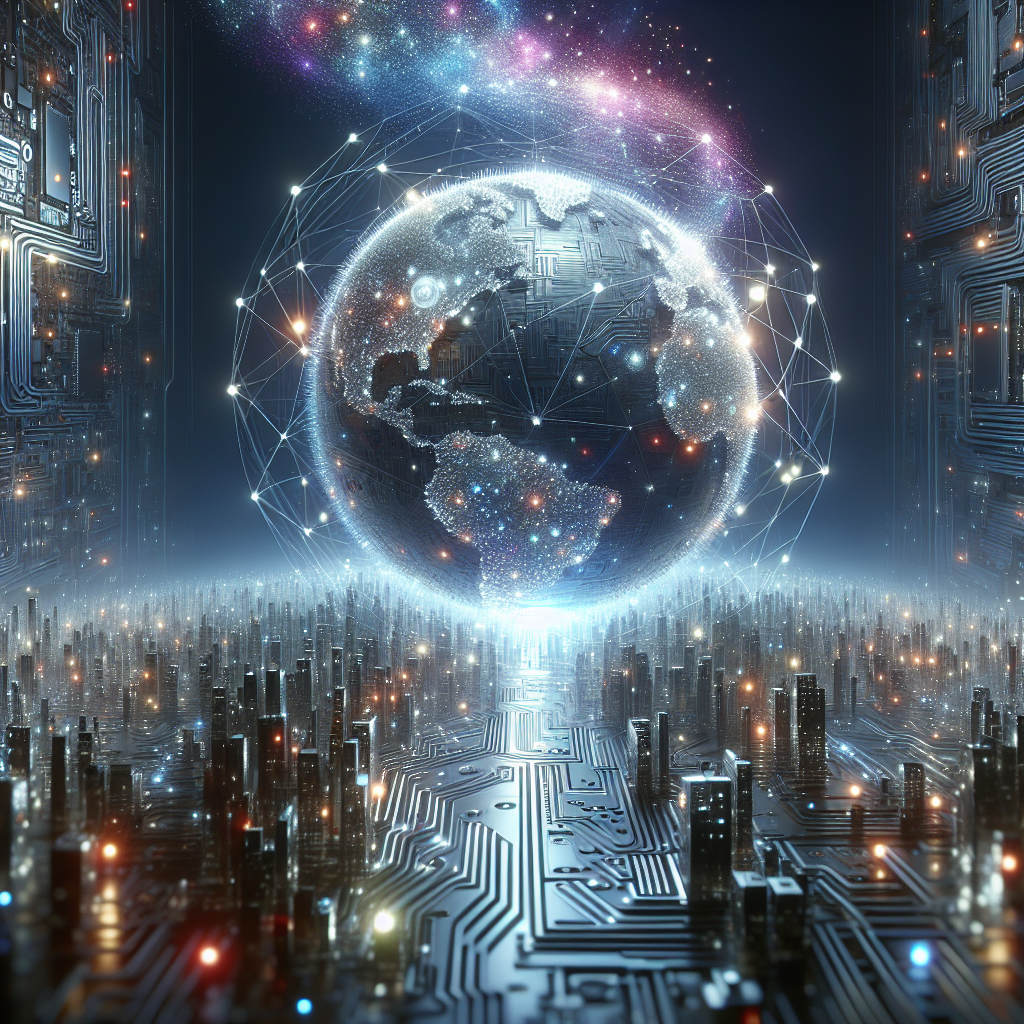
Navigating the Generative AI Landscape – A Guide for Data Scientists
In recent years, generative Artificial Intelligence (AI) has emerged as a transformative force across numerous industries, reshaping how we approach data analysis, predictive modeling, and content creation. San Francisco, long recognized as a hub for tech innovation, continues to lead in exploring these advancements. For data scientists, understanding the landscape of generative AI is crucial not only for leveraging its capabilities but also for addressing inherent challenges such as bias and ethical considerations.
Introduction
Generative AI, powered by groundbreaking technologies from companies like OpenAI, has redefined the boundaries of creativity and efficiency across various fields. Data scientists find themselves at the forefront of this revolution, tasked with integrating these new tools into existing workflows while ensuring accuracy, transparency, and fairness. This blog post delves into generative AI trends, the evolving roles of data scientists in AI, challenges they face, best practices for integration, and the broader impact on the field of data science.
Understanding Generative AI Trends
The Rise of Generative Models
Generative AI includes models like GPT-3 from OpenAI that can generate human-like text, images, and even code. These models are revolutionizing tasks ranging from content creation to complex problem-solving in industries such as finance, healthcare, and marketing.
Key Trends
- Increased Adoption Across Industries: Businesses across sectors are increasingly adopting generative AI for various applications. For example, in the healthcare sector, generative models assist in creating personalized treatment plans by analyzing patient data and predicting outcomes.
- Improved Model Sophistication: Models are becoming more sophisticated, capable of generating higher quality outputs with fewer inputs. This is particularly evident in creative industries where AI-generated art and music are gaining popularity.
- Focus on Personalization: Generative models are being used to create personalized experiences in marketing and customer service. By analyzing consumer behavior data, these models can tailor recommendations and interactions to individual preferences, enhancing user engagement.
- Integration with Other Technologies: The integration of generative AI with other emerging technologies like blockchain and IoT is opening new avenues for innovation. For instance, smart cities are leveraging AI to optimize energy consumption and traffic management.
- Ethical AI Development: There is a growing emphasis on developing ethical AI systems that prioritize fairness, accountability, and transparency. This trend is driven by increased awareness of the societal impacts of AI technologies.
Roles of Data Scientists in the Era of Generative AI
Enhancing Predictive Modeling
Data scientists can leverage generative AI to enhance predictive modeling by using it for data augmentation, which helps improve model robustness. By generating synthetic datasets that mirror real-world complexities, these models aid in overcoming data scarcity and improving prediction accuracy.
For instance, in financial forecasting, generative AI can create diverse economic scenarios to test the resilience of investment strategies. This approach allows data scientists to better understand potential risks and make more informed decisions.
Driving Innovation
As part of multidisciplinary teams, data scientists are pivotal in exploring innovative applications of generative AI, from automating report generation to creating dynamic content for social media strategies. They play a key role in identifying opportunities where AI can streamline processes and enhance productivity.
In marketing, data scientists use generative AI to craft personalized advertising campaigns that resonate with target audiences. By analyzing consumer data, they can generate engaging content that drives higher conversion rates.
Collaborating Across Domains
Data scientists are increasingly collaborating with professionals from other fields to harness the full potential of generative AI. For example, in healthcare, they work alongside medical experts to develop AI-driven diagnostic tools that improve patient outcomes.
In education, data scientists collaborate with educators to create personalized learning experiences using AI-generated content tailored to individual student needs and learning styles.
Challenges Data Scientists Face with Generative AI
Ethical Considerations and Bias Mitigation
One significant challenge is addressing ethical concerns and bias. Generative AI models can inadvertently perpetuate or even amplify existing biases present in training data. This can lead to unfair outcomes, particularly in sensitive areas like hiring practices or criminal justice.
Data scientists must implement rigorous testing and validation processes to identify and mitigate biases. Techniques such as fairness-aware machine learning and diverse dataset curation are essential for developing equitable AI systems.
Ensuring Transparency and Accountability
Another challenge is ensuring transparency and accountability in AI-generated outputs. Data scientists need to develop methods for explaining how AI models arrive at their conclusions, which is crucial for building trust with stakeholders.
Explainable AI (XAI) techniques are being developed to provide insights into model decision-making processes. These tools help data scientists communicate the rationale behind AI-driven decisions, fostering greater acceptance and understanding.
Data Privacy and Security
With generative AI relying heavily on large datasets, ensuring data privacy and security is paramount. Data scientists must implement robust encryption and access control measures to protect sensitive information from unauthorized use or breaches.
Compliance with data protection regulations like GDPR is essential for maintaining user trust and avoiding legal repercussions. Data scientists play a critical role in designing systems that prioritize data confidentiality while enabling AI innovation.
Best Practices in Generative AI
Continuous Learning and Adaptation
To stay ahead in the rapidly evolving field of generative AI, data scientists must commit to continuous learning and adaptation. Engaging with professional networks like the Data Science Association provides access to resources, workshops, and conferences that keep practitioners informed about the latest advancements.
Collaborative Development
Collaborative development is key to successful generative AI projects. By fostering interdisciplinary teams, data scientists can leverage diverse expertise to address complex challenges and drive innovation.
For example, in autonomous vehicle development, collaboration between data scientists, engineers, and regulatory experts ensures that AI systems are safe, reliable, and compliant with industry standards.
Ethical Frameworks
Implementing ethical frameworks is essential for guiding the responsible use of generative AI. Data scientists should adhere to principles such as fairness, accountability, and transparency when designing and deploying AI models.
Organizations can establish ethics committees to oversee AI projects, ensuring that they align with societal values and ethical standards. These committees provide guidance on addressing potential biases and mitigating risks associated with AI technologies.
Impact of Generative AI on Data Science
Transforming Industries
Generative AI is transforming industries by enabling new capabilities and enhancing existing processes. In finance, AI-driven models optimize investment strategies and risk management. In healthcare, AI tools assist in early disease detection and personalized treatment planning.
Data scientists play a crucial role in these transformations by developing and refining AI models that drive innovation and efficiency across sectors.
Empowering Decision-Making
Generative AI empowers decision-makers with actionable insights derived from complex data analyses. By automating routine tasks and generating predictive scenarios, AI allows organizations to focus on strategic planning and informed decision-making.
For instance, in supply chain management, AI-generated forecasts help companies anticipate demand fluctuations and optimize inventory levels, reducing costs and improving service delivery.
Fostering Creativity
Generative AI is also fostering creativity by providing tools for artists, writers, and designers to explore new forms of expression. AI-generated art and music are gaining recognition, challenging traditional notions of authorship and creativity.
Data scientists contribute to this creative renaissance by developing algorithms that enhance artistic capabilities and enable novel collaborations between humans and machines.
Conclusion
As generative AI continues to evolve, data scientists will remain at the forefront of its integration into various industries. By understanding generative AI trends, embracing new roles, addressing challenges, and adhering to best practices, they can harness the transformative potential of these technologies while ensuring ethical and responsible use.
Through continuous learning, collaboration, and commitment to transparency, data scientists will play a pivotal role in shaping the future impact of generative AI on our world. By staying informed and engaged, they will not only contribute to the advancement of AI but also ensure that it benefits society as a whole.
Joining networks like the Data Science Association can provide invaluable resources and support as data scientists navigate this exciting landscape. Together, we can drive innovation, foster creativity, and empower decision-making in an increasingly AI-driven world.