Driving Innovation in AI Agent Architectures
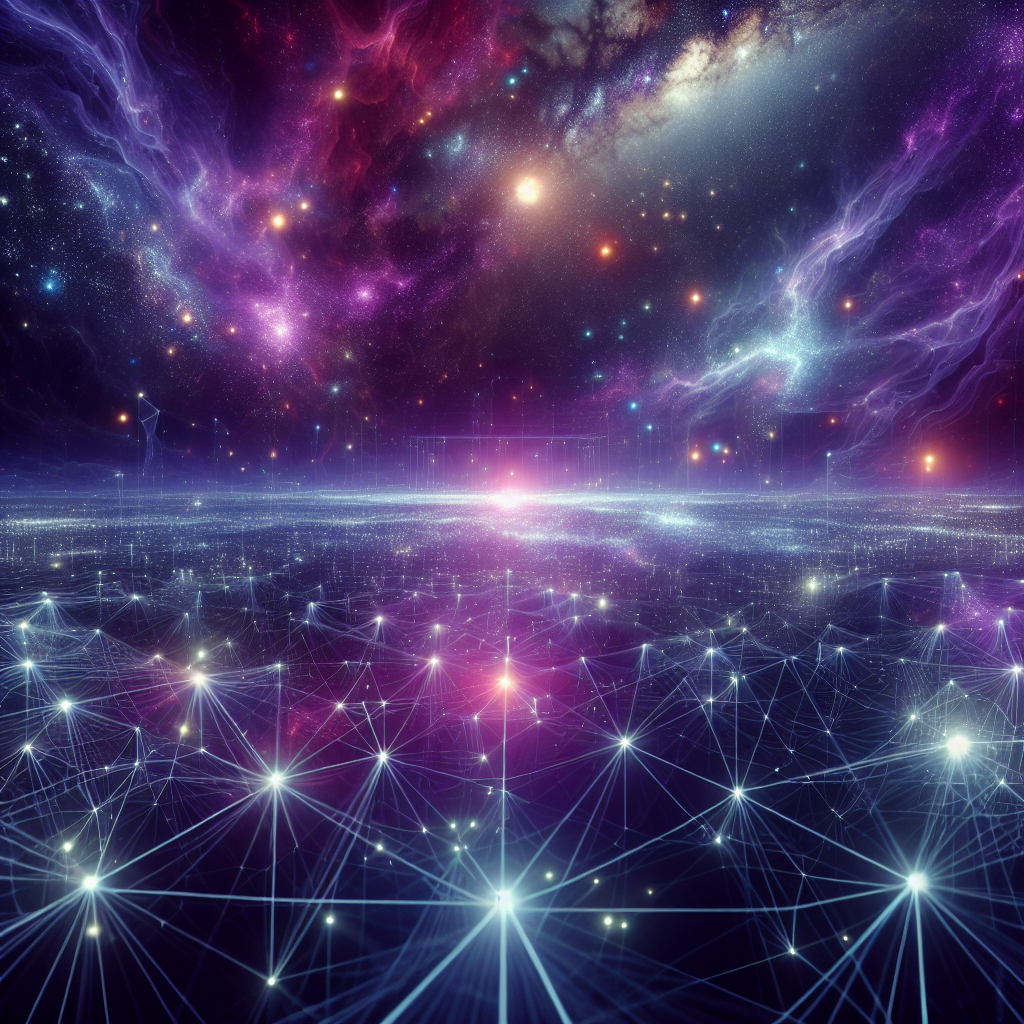
Introduction
Artificial Intelligence (AI) is revolutionizing industries worldwide by powering autonomous systems that enhance efficiency and drive innovation. At the core of this transformation are AI agent architectures, the foundational frameworks that underpin advancements in machine learning. Understanding these architectures is essential to unlocking their potential across various sectors. This blog post explores the latest trends in artificial intelligence architecture development, examines emerging technologies impacting AI-driven decision-making processes, and highlights contributions from industry leaders such as OpenAI and Google DeepMind. Silicon Valley stands at the forefront of this technological evolution, serving as a hub for groundbreaking advancements.
Latest Trends in Artificial Intelligence Architecture Development
The Evolution of AI Agent Architectures
In recent years, AI agent architectures have undergone significant evolution, driven by breakthroughs in machine learning and data processing capabilities. Traditional architectures were largely rule-based systems with limited adaptability. Today, the focus is on creating adaptive, scalable, and intelligent agents capable of autonomous decision-making.
- Hybrid Models: These models merge symbolic reasoning with neural networks, offering a balanced approach to problem-solving. This fusion allows AI agents to not only learn from data but also utilize structured knowledge representations that can guide their learning processes.
- Reinforcement Learning (RL): A subfield concentrating on training agents through rewards and penalties, enabling them to learn optimal actions over time. Companies like Google DeepMind have made remarkable progress in this area, with their AlphaGo program defeating human champions. RL has further evolved into more sophisticated techniques such as deep reinforcement learning (DRL), where neural networks are used to approximate the complex decision-making processes.
- Transfer Learning: This approach enables AI agents to apply knowledge gained from one task to different but related tasks. By leveraging pre-trained models, developers can save time and resources while achieving higher performance in new domains.
OpenAI’s Contributions
OpenAI has played a pivotal role in shaping modern AI agent architectures. Their research into deep reinforcement learning and neural networks has led to groundbreaking applications in autonomous systems. Notably, the development of advanced models by OpenAI showcases how these frameworks can be leveraged for machine learning advancements. The organization’s GPT series exemplifies innovations in natural language processing (NLP), demonstrating unprecedented abilities in text generation and comprehension.
OpenAI’s initiatives extend beyond academic contributions; they are actively engaged in creating accessible AI technologies through open-source platforms, encouraging widespread innovation across industries. Their work emphasizes the importance of safety and alignment in AI systems, ensuring that these powerful tools benefit humanity responsibly.
Exploring the Impact of Emerging Technologies on AI-Driven Decision-Making Processes
Emerging technologies are significantly altering the landscape of AI-driven decision-making processes. The integration of Internet of Things (IoT) devices with AI systems allows for distributed processing capabilities through edge computing, enabling real-time data analysis and enhancing the efficiency and responsiveness of autonomous systems across various industries.
Edge Computing and IoT Integration
The synergy between IoT and edge computing enhances AI agent architectures by minimizing latency and reducing bandwidth usage. Devices can process data locally, allowing for swift decision-making without needing to transmit information to centralized cloud servers. This capability is particularly valuable in sectors like healthcare, where real-time monitoring and response are critical.
Blockchain Technology
Blockchain technology offers a decentralized approach to data management that enhances the transparency and security of AI-driven processes. By providing immutable records, blockchain ensures the integrity of decisions made by autonomous systems, which is crucial for applications in finance and supply chain management.
Understanding the Latest Trends in Artificial Intelligence Architecture Development
The rapid advancements in artificial intelligence architecture development are fueled by a deeper understanding of machine learning algorithms and their applications. Innovations such as neural networks have become integral to developing sophisticated AI models that adapt and improve over time, driving significant progress in autonomous systems innovation.
Role of Neural Networks
Neural networks play a crucial role in enhancing AI-driven decision-making processes by enabling machines to recognize patterns and make predictions based on vast amounts of data. Their ability to learn from examples makes them indispensable for developing advanced AI models that continually adapt and improve. Convolutional neural networks (CNNs) and recurrent neural networks (RNNs), for example, are instrumental in processing image and sequential data, respectively.
Generative Adversarial Networks (GANs)
Generative Adversarial Networks (GANs) represent another leap forward in AI agent architectures. By using two competing networks – a generator and a discriminator – GANs can produce highly realistic synthetic data. This capability is transformative for fields such as computer vision, where it enables the creation of training datasets that can enhance model accuracy without compromising privacy.
Silicon Valley: The Epicenter of AI Innovation
Silicon Valley is globally recognized as the epicenter for technological advancement, particularly in artificial intelligence. Home to leading tech companies and research institutions, it offers a rich environment teeming with resources and talent that propel innovations in AI agent architectures and machine learning technologies. This vibrant ecosystem fosters collaboration and competition, driving rapid advancements and bringing cutting-edge solutions to market.
Collaboration Hubs
Silicon Valley’s unique culture of open innovation is epitomized by its numerous tech hubs and accelerators where entrepreneurs, researchers, and corporations converge to share ideas and resources. Institutions like Stanford University and the Singularity University provide platforms for interdisciplinary collaboration that fuel breakthroughs in AI technologies.
Ethical Considerations and Future Directions
As AI agent architectures continue to evolve, addressing ethical considerations becomes increasingly crucial. Ensuring fairness, accountability, and transparency in AI systems is vital to building trust among users and stakeholders. Techniques such as explainable AI (XAI) are being developed to make the decision-making processes of autonomous agents more understandable to humans.
Looking ahead, the future of AI agent architectures will likely involve even greater integration with other emerging technologies, like quantum computing, which promises to solve complex problems faster than classical computers. This convergence could unlock new possibilities for AI applications that we can only begin to imagine today.
Conclusion
The landscape of AI agent architectures is rapidly advancing, driven by innovations in machine learning and the integration of emerging technologies. Industry leaders like OpenAI and Google DeepMind are at the forefront of these developments, pushing the boundaries of what autonomous systems can achieve. As these architectures become more sophisticated, they hold the potential to transform industries and improve quality of life worldwide.
By understanding these trends and embracing ethical practices, we can ensure that AI agent architectures continue to drive positive change, unlocking new opportunities for innovation and collaboration across the globe.