Generative Models for Data Science
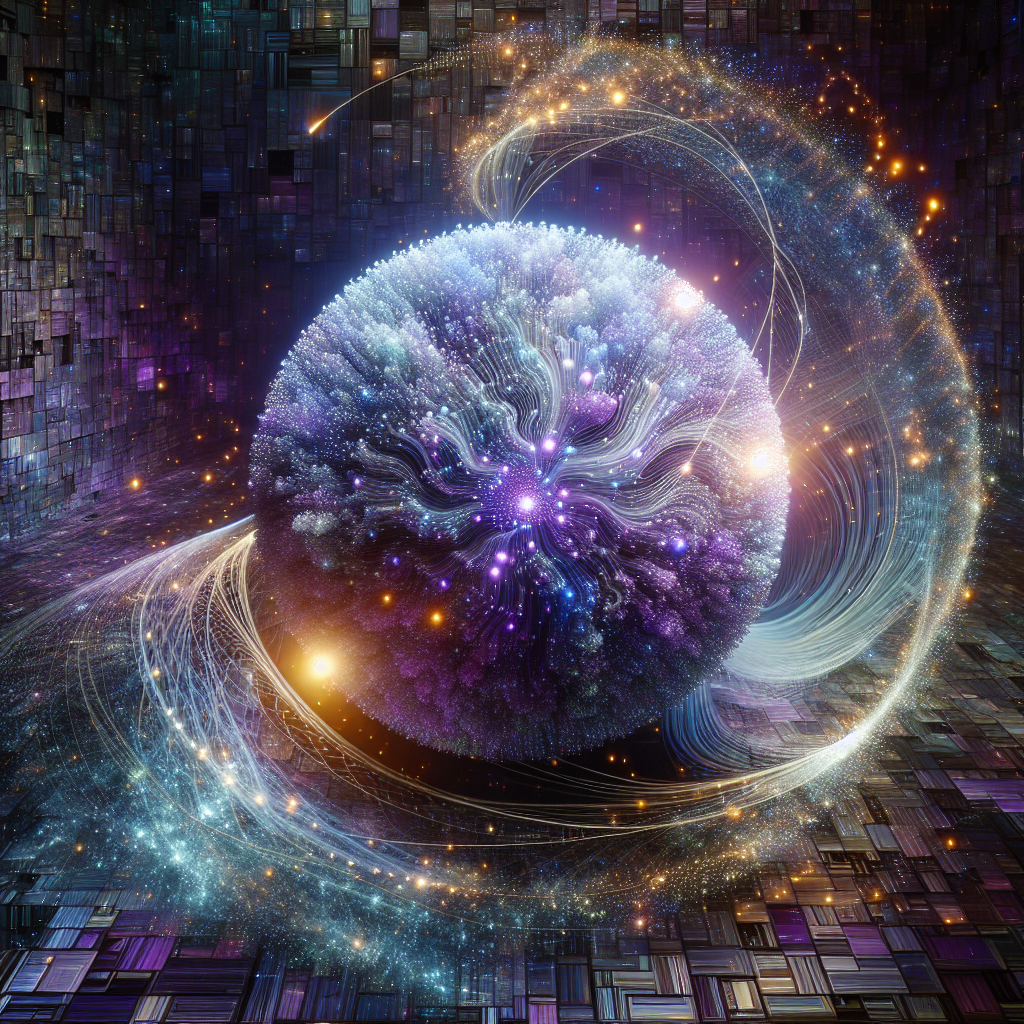
In today’s data-driven world, organizations are constantly seeking innovative ways to enhance their data capabilities. Among these advancements, deep learning generative models stand out for their transformative potential in creating synthetic data that mirrors real-world datasets. These technologies not only offer solutions to pressing challenges like data privacy and scarcity but also open new avenues for innovation and growth.
Understanding Deep Learning Generative Models
At the heart of this revolution are deep learning techniques that significantly advance how generative models create synthetic data. Two key players in this arena are Variational Autoencoders (VAEs) and Generative Adversarial Networks (GANs). These models leverage complex algorithms to learn from existing datasets, enabling them to generate new, high-quality data points indistinguishable from the real ones.
The Role of VAEs and GANs
Variational Autoencoders (VAEs) function through a probabilistic approach. They encode input data into a latent space representation—a compressed form that captures essential features—and then decode it back to produce new data points. This process allows for smooth interpolation between data samples, providing an intuitive way to generate diverse datasets. VAEs are particularly effective in applications requiring consistent quality and variation, such as creating realistic simulations of environments or enhancing image datasets.
Generative Adversarial Networks (GANs) consist of two neural networks—a generator and a discriminator—that compete against each other. The generator creates synthetic data, while the discriminator evaluates its authenticity compared to real data. Through this adversarial process, GANs can produce highly realistic outputs, making them invaluable for applications requiring high-fidelity data, such as photorealistic image synthesis or video generation.
Case Study: GANs in Fashion Design
A notable application of GANs is in the fashion industry, where companies like NVIDIA and Zalando have used these models to generate new clothing designs. By training on vast collections of existing designs, GANs can create innovative and diverse fashion items, significantly speeding up the design process and reducing costs associated with traditional methods.
Applications Across Industries
Generative models have found their place across various sectors due to their versatility and power:
- Healthcare: In medical imaging, VAEs and GANs help create synthetic datasets that maintain patient privacy while allowing researchers to develop advanced diagnostic tools. For instance, researchers at Stanford University have used these models to generate high-resolution images of rare conditions, facilitating better training for AI diagnostic systems without compromising patient confidentiality.
- Urban Planning: Cities like New York are utilizing GANs to simulate traffic patterns and optimize infrastructure development. By generating realistic scenarios based on historical data, planners can make informed decisions that enhance urban living conditions. This approach has led to more efficient public transportation systems and better emergency response strategies.
- AI Research: Institutions such as OpenAI and Stanford University lead the way in exploring the boundaries of generative models. Their work not only contributes to academic knowledge but also drives practical applications in technology solutions, from natural language processing to autonomous vehicles.
Future Predictions: The Expansion of Generative Models
As deep learning techniques continue to evolve, we can anticipate further expansion of generative models into new domains. For example, the entertainment industry is already exploring GANs for creating realistic virtual environments and characters, enhancing user experiences in video games and movies.
Moreover, as computational power increases and algorithms become more sophisticated, the potential applications of VAEs and GANs will likely grow exponentially, offering even more innovative solutions to complex problems across industries.
Overcoming Challenges
While the potential of deep learning generative models is immense, they come with their own set of challenges:
- Data Quality and Bias: Generative models are only as good as the data they are trained on. If the training dataset contains biases or inaccuracies, these can be reflected in the generated outputs. Ensuring high-quality, diverse datasets is crucial for minimizing bias and improving model performance.
- Ethical Concerns: The ability to create realistic synthetic content raises ethical questions, particularly regarding misinformation and deepfakes. Organizations must establish guidelines and safeguards to prevent misuse of generative technologies.
- Computational Resources: Training complex models like GANs requires significant computational power and time. As these models become more advanced, the demand for resources will continue to grow, necessitating investment in infrastructure and optimization techniques.
Actionable Insights: Implementing Generative Models
For organizations looking to implement generative models, consider the following actionable insights:
- Start with a Clear Objective: Define what you aim to achieve with generative modeling—whether it’s enhancing data privacy, generating new product designs, or improving AI training datasets.
- Invest in Quality Data: Ensure your datasets are comprehensive and representative of the diversity needed for accurate model outputs. Collaborate with experts to curate and preprocess data effectively.
- Stay Informed on Ethical Practices: Develop a framework for ethical use of generative technologies, considering potential risks and establishing protocols to mitigate them.
- Leverage Expertise: Partner with institutions like OpenAI or Stanford University to access cutting-edge research and expertise in generative modeling.
- Plan for Scalability: As your needs grow, ensure that your infrastructure can support larger models and datasets without compromising performance.
Conclusion
Deep learning generative models, particularly Variational Autoencoders (VAEs) and Generative Adversarial Networks (GANs), are revolutionizing data science by creating synthetic data that mirrors real-world complexities. These models offer innovative solutions across industries, from healthcare to urban planning, while also presenting new challenges related to ethics and resource management.
As technology continues to advance, the applications of generative models will only expand, offering unprecedented opportunities for innovation and growth. By understanding their capabilities and addressing associated challenges, organizations can harness the power of deep learning to drive transformational change in their industries.
Embark on Your Generative Modeling Journey
Let’s embark on this transformative journey together! If you’re ready to explore the potential of Variational Autoencoders (VAEs) and Generative Adversarial Networks (GANs), reach out for expert guidance. By leveraging these powerful technologies, we can unlock new possibilities and lead the charge in data-driven innovation.