Building Resilient AI Networks Now
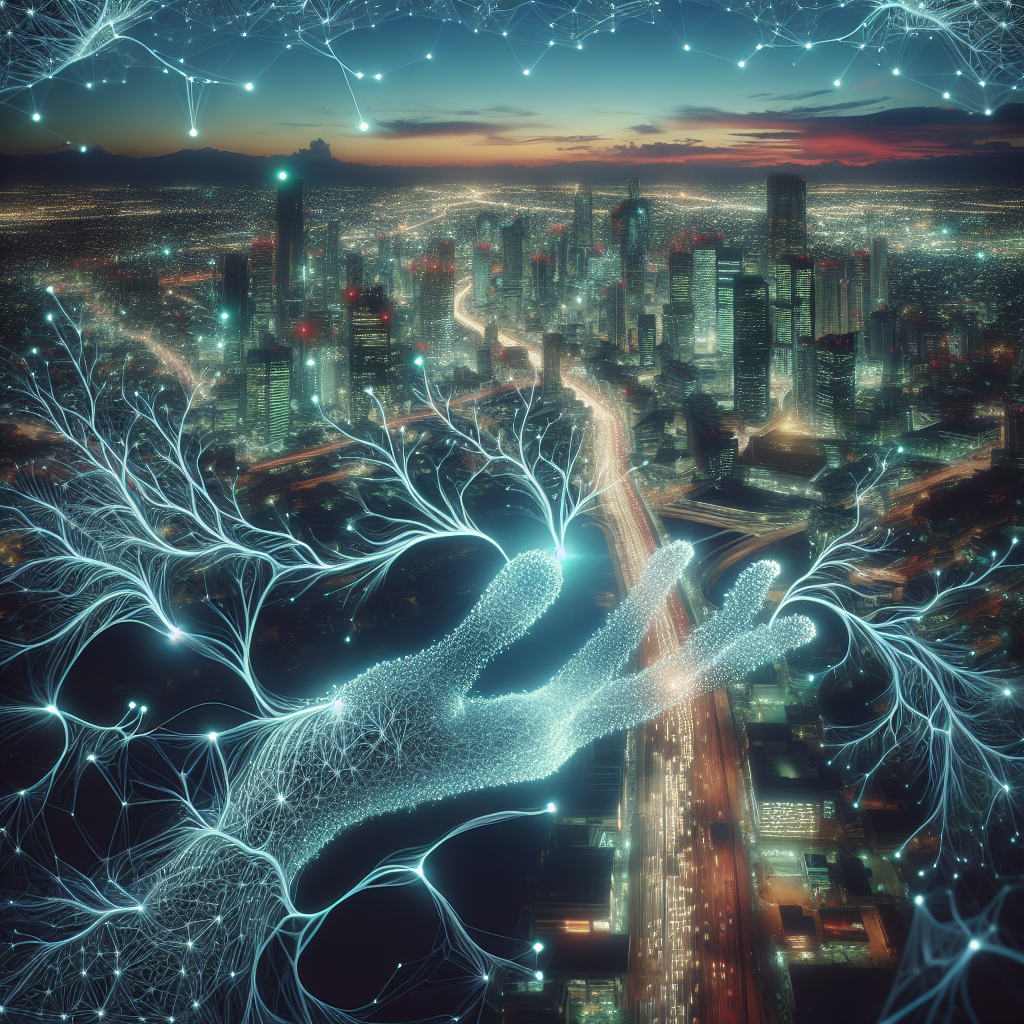
In today’s fast-paced digital landscape, artificial intelligence (AI) networks have become crucial to business operations. However, this increased reliance brings with it a critical need for robustness and resilience. According to OpenAI, resilient AI systems could save businesses millions in downtime costs annually by preventing disruptions.
This blog post explores strategies for building resilient AI networks, focusing on network robustness strategies, implementing redundancy measures, and techniques for mitigating risks within AI-driven networks. By understanding these strategies, decision-makers can enhance their AI infrastructure resilience, ensuring long-term success and reliability.
Introduction
As businesses increasingly rely on AI to drive innovation and efficiency, the importance of resilient AI systems cannot be overstated. Network robustness is crucial in maintaining continuous operations and protecting against unforeseen disruptions. Drawing from cutting-edge research by institutions like MIT Media Lab and insights from industry leaders in Silicon Valley, this article provides actionable insights for building resilient AI networks.
Understanding Resilient AI Systems
What are Resilient AI Systems?
Resilient AI systems are designed to withstand and quickly recover from disruptions, maintaining functionality even when faced with failures or attacks. This ensures minimal impact on business operations. Key components include redundancy, fault tolerance, and rapid recovery mechanisms.
Redundancy: By incorporating multiple instances of critical components, businesses can ensure continuity of service even if one part fails. For example, a dual-server setup where each server functions as a backup for the other allows seamless operation during maintenance or unexpected failures.
Fault Tolerance: This involves designing AI systems that can continue operating properly in the event of a failure within some of its components. Techniques such as error detection and correction help maintain system integrity even when parts are compromised.
Rapid Recovery Mechanisms: These include automated processes to restore normal operations quickly after a disruption. Implementing regular testing of backup and recovery protocols ensures that businesses can bounce back from failures swiftly.
Importance of Network Robustness
Network robustness refers to the ability of an AI network to handle unexpected challenges without significant degradation in performance. It involves strategic planning and implementation of measures that enhance resilience against both internal and external threats.
A robust network is not only about preventing outages but also about minimizing their impact when they occur. This can involve real-time monitoring tools that alert teams to anomalies, allowing for proactive rather than reactive management of issues.
Strategies for Building Resilient AI Networks
1. Implementing Redundancy Measures
Redundancy is a critical strategy for enhancing AI network reliability, ensuring continuous operation even if one part of the system fails. This approach minimizes downtime and maintains service quality.
- Servers and Data Centers: Incorporate duplicate components like servers or data centers. For instance, using geographically dispersed data centers can prevent localized disasters from disrupting operations entirely.
- Data Backup Systems: Ensure multiple backups to prevent data loss during failures. Regularly test these systems to ensure they are functioning as intended.
- Cloud-Based Solutions: Utilize cloud services that inherently provide redundancy through distributed architecture, ensuring that even if one server or location experiences issues, others can take over seamlessly.
2. Techniques for Mitigating Risks within AI-driven Networks
Risk mitigation is essential for safeguarding against potential threats in AI networks. Implement the following techniques:
- Regular Security Audits: Conduct thorough assessments to identify vulnerabilities and rectify them promptly. Engage third-party experts periodically to gain an unbiased perspective on your security posture.
- Threat Modeling: Anticipate potential threats by mapping out possible attack vectors and developing strategies to counteract them. This proactive approach allows for more effective risk management.
- Cybersecurity Best Practices: Implement industry-standard practices such as encryption, multi-factor authentication, and access controls to protect data integrity and confidentiality.
Additional Strategies
Adopting AI Monitoring Tools: Use advanced monitoring tools that leverage machine learning algorithms to detect anomalies in real-time. These tools can provide insights into system performance, helping teams identify and address issues before they escalate.
Regular Training for Staff: Ensure that all staff members are trained on the latest cybersecurity threats and best practices. This includes regular updates on potential vulnerabilities and how to respond effectively to incidents.
Data Integrity Checks: Implement automated processes to verify data integrity continuously. These checks can help detect and rectify errors or corruption in datasets, ensuring consistent and accurate AI outputs.
Case Studies and Examples
OpenAI’s Approach to Network Resilience
OpenAI has set a benchmark for resilient AI networks through its robust architecture and rigorous testing protocols. The organization employs a combination of redundancy, fault tolerance, and rapid recovery mechanisms that allow it to maintain high availability and performance standards. By regularly conducting failure simulations and stress tests, OpenAI ensures its systems can handle unexpected challenges without significant disruptions.
MIT Media Lab’s Innovations
MIT Media Lab has been at the forefront of developing resilient AI networks by exploring novel approaches such as decentralized architectures and adaptive learning algorithms. Their research focuses on creating systems that can autonomously adapt to changing environments and recover from failures efficiently, providing valuable insights for businesses looking to enhance their network resilience.
Industry Trends and Future Predictions
As AI continues to evolve, the demand for resilient AI networks is expected to grow. Key trends include:
- Increased Use of Edge Computing: By processing data closer to its source, edge computing reduces latency and enhances reliability, making it an attractive option for businesses seeking more robust AI solutions.
- AI in Cybersecurity: The integration of AI into cybersecurity measures is becoming increasingly common. AI-driven tools can detect threats faster and with greater accuracy than traditional methods, providing enhanced protection against cyberattacks.
- Adoption of 5G Technology: With the rollout of 5G networks, businesses will have access to faster and more reliable connectivity. This advancement will support the development of resilient AI networks capable of handling larger volumes of data in real-time.
Actionable Insights for Business Leaders
To build a resilient AI network, business leaders should:
- Conduct Regular Risk Assessments: Identify potential vulnerabilities and develop strategies to mitigate them proactively.
- Invest in Redundancy: Ensure critical components have backups in place to maintain continuity during failures.
- Stay Informed on Emerging Threats: Keep abreast of the latest cybersecurity developments and adjust your strategies accordingly.
- Leverage Advanced Technologies: Utilize AI monitoring tools, cloud services, and edge computing to enhance network resilience.
- Foster a Culture of Security Awareness: Train employees regularly and encourage a security-first mindset across all levels of the organization.
Conclusion
Building resilient AI networks is essential for businesses looking to thrive in today’s digital landscape. By implementing redundancy measures, mitigating risks effectively, and staying informed on industry trends, organizations can enhance their network resilience and ensure continuous operation even in the face of disruptions. Drawing inspiration from leaders like OpenAI and MIT Media Lab, businesses can develop robust AI systems that not only withstand challenges but also adapt and grow over time.
By prioritizing resilient AI networks, business leaders can safeguard their operations, protect valuable data, and maintain a competitive edge in an increasingly interconnected world.