Generative AI Applications Across Sectors
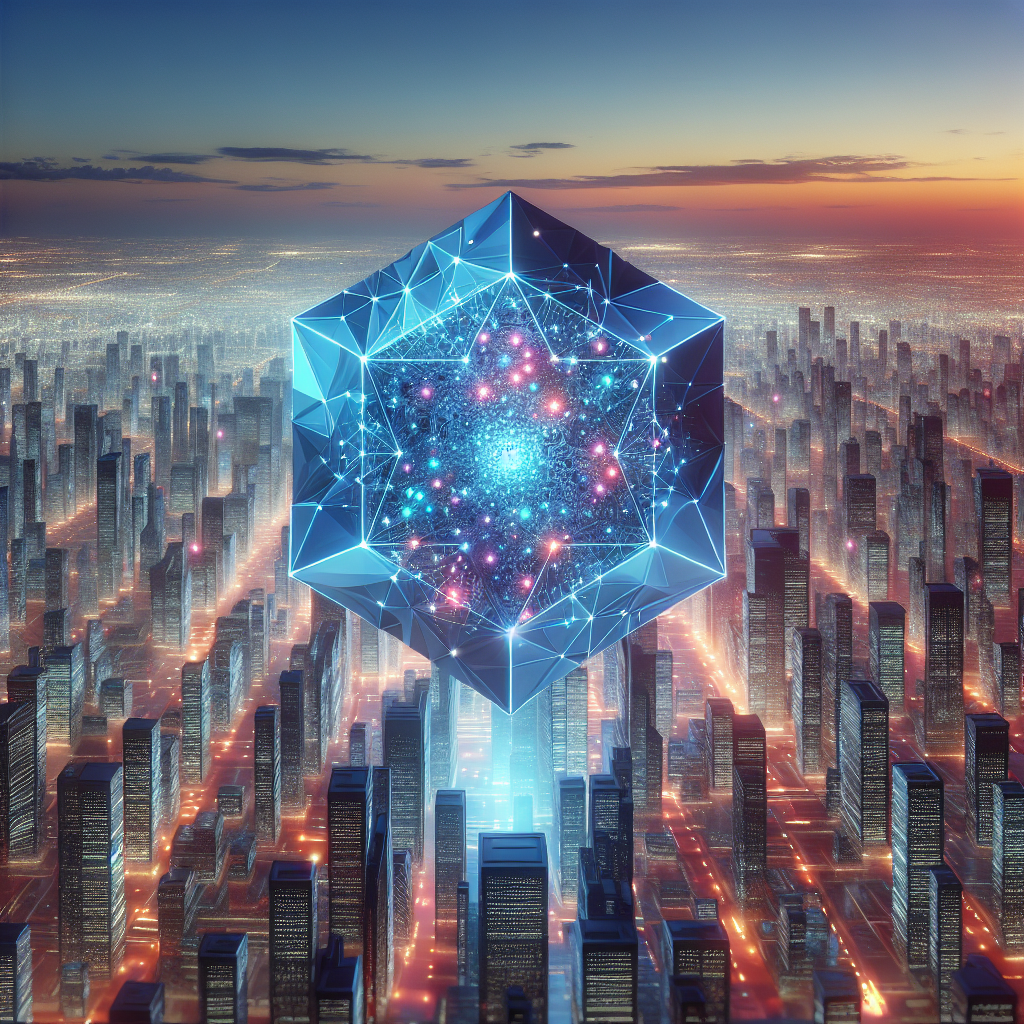
Are you intrigued by how generative Artificial Intelligence (AI) is reshaping industries far and wide? Imagine a world where technology not only enhances existing processes but creates entirely new possibilities—this is the transformative power of generative AI. From revolutionizing patient care in healthcare to advancing fraud detection in finance, and even sparking boundless creativity in the arts, generative AI is fast becoming indispensable. Let’s dive into how businesses can leverage this cutting-edge technology and explore actionable insights for decision-makers eager to integrate these innovations.
Introduction
We’re witnessing a pivotal moment in technological evolution with the rise of generative AI. Unlike traditional AI that analyzes data to predict patterns, generative AI models craft new content—whether it’s text, images, or even music—that mirrors human creativity and intelligence. This remarkable capability is reshaping industries by enabling personalized patient treatment plans in healthcare, sophisticated fraud detection mechanisms in finance, and innovative content creation across creative fields.
As a business leader, understanding the potential of generative AI—and how to integrate these tools effectively—is crucial. So, let’s talk about how you can harness this transformative power within your organization.
Prerequisites for Integrating Generative AI
Before diving into the world of generative AI, make sure your team is ready with the right resources:
- Understanding of AI Fundamentals: Start with a basic grasp of AI concepts and technologies. Familiarizing yourself with machine learning models, neural networks, and data processing techniques will be invaluable.
- Technical Infrastructure: Ensure you have robust computational power, often requiring cloud-based solutions like AWS or Google Cloud to handle the intensive processing needs.
- Data Availability: Access high-quality data for training your generative AI models. The more diverse and comprehensive your dataset, the better your model’s performance will be.
- Skilled Personnel: Have expertise in machine learning, data science, and domain-specific knowledge. Consider partnering with experts or investing in training to build a competent team.
Step-by-Step Guide to Implementing Generative AI
Ready to embark on this exciting journey? Here’s how you can integrate generative AI into your business:
1. Define Your Objectives
First things first—clarify what you aim to achieve with generative AI. Whether it’s personalizing patient treatment plans in healthcare or creating compelling marketing content, setting clear goals will steer your implementation strategy.
Example: A healthcare provider might focus on integrating generative AI into their Electronic Health Records (EHR) systems to predict individualized care pathways for enhanced patient outcomes. By analyzing vast datasets of patient histories and medical research, generative AI can suggest tailored treatment plans that improve recovery rates and reduce costs.
2. Conduct a Needs Assessment
Evaluate your current processes and pinpoint where generative AI can add value. Assess existing capabilities, limitations, and potential bottlenecks that AI could address.
Example: A financial institution might identify the need for advanced fraud detection by analyzing transaction patterns to flag anomalies more efficiently than traditional systems. Generative AI models like OpenAI’s GPT-4 can analyze complex datasets to detect suspicious activities, reducing false positives and saving time and resources.
3. Choose the Right Generative AI Tools
Selecting appropriate tools is crucial. OpenAI offers a range of generative models, like GPT-4, that can be tailored to various industry-specific applications.
Example: A marketing agency might choose DALL-E for generating unique visual content that captures and engages their audience’s imagination. This tool can create custom graphics from textual descriptions, saving time and resources while boosting creativity.
4. Train and Fine-Tune Models
Work with data scientists to train your chosen generative AI models using relevant datasets. Fine-tuning ensures the outputs align closely with business objectives and quality standards.
Example: A hospital could fine-tune a model on anonymized patient records to boost predictive accuracy in treatment planning. By continuously updating the dataset, the model can adapt to new medical insights and improve its recommendations over time.
5. Implement Integration Strategies
Develop strategies for seamlessly integrating generative AI into existing workflows. This might involve API integration, developing custom applications, or using pre-built solutions offered by platforms like OpenAI.
Example: An e-commerce company could integrate a generative AI tool that automatically crafts personalized product descriptions based on customer data. By analyzing purchase history and browsing behavior, the tool can create tailored content that resonates with individual customers, enhancing their shopping experience and boosting sales.
6. Monitor and Optimize Performance
After deployment, continuously monitor the performance of your generative AI systems. Gather feedback to refine models and address any emerging issues promptly.
Example: A financial firm might set up regular audits to evaluate the accuracy of its fraud detection system, making adjustments as needed for optimal performance. By analyzing false positives and negatives, they can fine-tune their model to improve reliability and trustworthiness.
Common Mistakes to Avoid
- Overlooking Ethical Considerations: Ensure compliance with data privacy regulations and ethical standards. Generative AI can inadvertently produce biased or inappropriate content if not properly managed.
- Neglecting Data Quality: Poor quality or insufficient data can lead to suboptimal AI performance. Investing in high-quality, diverse datasets is crucial for training effective models.
- Underestimating Change Management: Address the cultural shift required for successful AI adoption. Engage your team through education and involvement in the implementation process.
Advanced Tips for Experts
For seasoned professionals, consider exploring:
- Hybrid Models: Combine generative and discriminative models to leverage strengths from both approaches. This can enhance accuracy and efficiency in various applications.
- AI Governance Frameworks: Establish guidelines ensuring accountability and transparency in AI decision-making processes. A robust framework helps mitigate risks and build trust among stakeholders.
- Continuous Learning Ecosystems: Foster an environment where team members stay updated on the latest advancements in AI. Encourage participation in workshops, conferences, and online courses to keep skills sharp and relevant.
Industry-Specific Insights
Healthcare: Personalizing Patient Treatment Plans
Generative AI is transforming healthcare by personalizing patient treatment plans. By analyzing vast amounts of medical data, these models can recommend tailored therapies that consider individual genetic profiles, lifestyle factors, and health histories. This approach not only improves patient outcomes but also optimizes resource allocation in hospitals.
Case Study: Mayo Clinic has been exploring the use of generative AI to enhance diagnostic accuracy and treatment recommendations. By integrating AI-driven insights into their clinical workflows, they aim to provide more precise care and reduce unnecessary procedures.
Finance: Enhancing Fraud Detection Systems
The financial sector leverages generative AI to enhance fraud detection systems. These models can analyze transaction patterns in real-time, identifying anomalies that may indicate fraudulent activity. This proactive approach helps banks and credit institutions protect their customers and minimize financial losses.
Statistic: According to a report by Juniper Research, AI-driven fraud detection tools could save the banking industry $25 billion annually by 2023.
Creative Industries: Content Creation and Design
In the creative industry, generative AI tools are being used for content creation and design. From generating unique artwork to writing engaging copy, these models offer new avenues for creativity and innovation. Businesses can leverage generative AI to streamline their creative processes and deliver personalized experiences to their audiences.
Example: Adobe’s Sensei, powered by AI, assists designers in creating stunning visuals by suggesting layout options, color schemes, and design elements based on the project’s requirements.
Future Predictions and Trends
As generative AI continues to evolve, we can expect even more groundbreaking applications across various sectors. Here are some trends to watch:
- Increased Automation: More industries will adopt generative AI for automating routine tasks, allowing human workers to focus on strategic initiatives.
- Enhanced Collaboration: AI tools will facilitate better collaboration between humans and machines, leading to more innovative solutions and improved productivity.
- Ethical AI Development: As awareness of ethical considerations grows, we’ll see a stronger emphasis on developing responsible AI systems that prioritize fairness, transparency, and accountability.
Frequently Asked Questions
How does generative AI differ from traditional AI?
Generative AI focuses on creating new content based on learned patterns, while traditional AI analyzes data and makes predictions. This distinction allows generative AI to be used creatively across various sectors.
What industries benefit most from generative AI?
Industries such as healthcare, finance, and creative fields like marketing and design see significant benefits. These sectors leverage the technology for tasks ranging from personalized treatment plans to innovative content creation.
Is it necessary to have a large dataset for training generative AI models?
While extensive datasets can enhance model performance, techniques like transfer learning allow effective use of smaller datasets by leveraging pre-trained models.
How long does it take to implement generative AI in an organization?
Implementation time varies based on factors like data availability and the complexity of integration. It could range from a few months to over a year.
What are the risks associated with generative AI?
Potential risks include ethical concerns, data privacy issues, and challenges related to model bias. Organizations must proactively address these through robust governance frameworks.
Ready to Transform Your Business with AI?
Are you ready to leverage the transformative power of generative AI within your organization? Our team specializes in AI Agentic software development and AI Cloud Agents services, having successfully implemented similar solutions across diverse industries. From healthcare providers crafting personalized treatment plans to financial institutions enhancing fraud detection systems, our expertise is your pathway to innovation.
Reach out for a consultation to explore how we can help integrate generative AI into your workflows. Visit our contact page or use the forms available on our website to get in touch with us. We’re more than happy to field any questions and provide tailored assistance that aligns with your specific needs.
Transforming your business landscape with generative AI isn’t just a step forward—it’s an evolution toward unprecedented efficiency, creativity, and insight. Let’s embark on this journey together!