AI Agents for Data Science Workflows
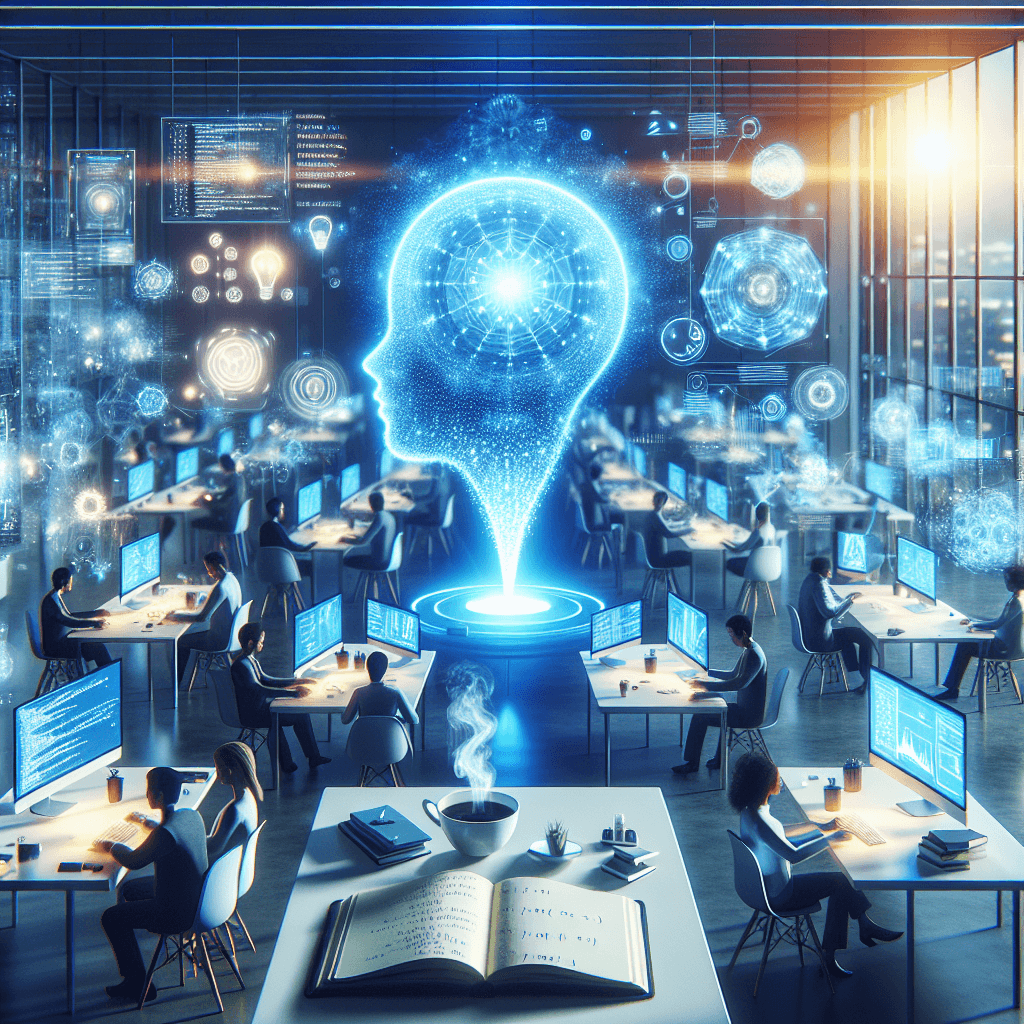
AI Agents for Data Science Workflows: Revolutionizing the Future of Data Analysis
The integration of AI Agents into Data Science has been a significant development in recent years, transforming the way data scientists approach their Workflows. In this blog post, we will delve into the world of AI Agents and explore how they are revolutionizing the field of Data Science. By applying AI agents to automate data science workflows, organizations can streamline their processes, improve efficiency, and uncover new insights.
Introduction to AI Agents
AI Agents are computer programs that use Artificial Intelligence (AI) and Machine Learning (ML) algorithms to perform tasks autonomously. These agents can learn from data, make decisions, and take actions without human intervention. In the context of Data Science, AI Agents can be used to automate repetitive tasks, improve data quality, and enhance decision-making. Understanding the role of AI agents in data science is crucial for organizations looking to leverage their capabilities.
Benefits of AI Agents in Data Science
The benefits of using AI Agents in Data Science Workflows are numerous. Some of the key advantages include:
- Automated Data Processing: AI Agents can quickly process large datasets, reducing the time and effort required for data preparation.
- Improved Data Quality: AI Agents can detect errors and inconsistencies in data, ensuring that only high-quality data is used for analysis.
- Enhanced Decision-Making: AI Agents can analyze data in real-time, providing insights and recommendations to support informed decision-making.
Companies like Databricks are already leveraging AI Agents to improve their data science workflows. By using AI Agents to automate tasks such as data cleaning and feature engineering, data scientists can focus on higher-level tasks that require human expertise.
How AI Agents Work
AI Agents use a combination of Natural Language Processing (NLP) and ML algorithms to understand and interact with data. They can be trained on specific datasets and tasks, allowing them to learn and improve over time. Some common techniques used by AI Agents include:
- Supervised Learning: AI Agents are trained on labeled datasets to learn patterns and relationships.
- Unsupervised Learning: AI Agents are trained on unlabeled datasets to discover hidden patterns and relationships.
- Reinforcement Learning: AI Agents learn through trial and error, receiving rewards or penalties for their actions.
Applications of AI Agents in Data Science
AI Agents have a wide range of applications in Data Science, including:
- Data Preparation: AI Agents can automate data cleaning, transformation, and feature engineering.
- Predictive Modeling: AI Agents can build and deploy predictive models using various ML algorithms.
- Data Visualization: AI Agents can create interactive and dynamic visualizations to communicate insights and findings.
Challenges and Limitations
While AI Agents have the potential to revolutionize Data Science Workflows, there are also challenges and limitations to consider. Some of the key challenges include:
- Data Quality: AI Agents require high-quality data to learn and make accurate predictions.
- Explainability: AI Agents can be difficult to interpret, making it challenging to understand their decision-making processes.
- Security: AI Agents can pose security risks if not properly designed and implemented.
Future of AI Agents in Data Science
The future of AI Agents in Data Science is exciting and rapidly evolving, and AI Agents are at the forefront of this evolution. Some potential areas of development include:
- Edge AI: The integration of AI Agents with edge computing to enable real-time processing and analysis of data.
- Explainable AI: The development of techniques to explain and interpret the decisions made by AI Agents.
Real-World Examples
Several companies are already leveraging AI Agents in their data science workflows. For example:
- Predictive Maintenance: Companies like Siemens are using AI Agents to predict equipment failures, reducing downtime and increasing overall efficiency.
- Personalized Recommendations: Companies like Netflix are using AI Agents to provide personalized recommendations to users, improving the user experience and driving engagement.
Getting Started with AI Agents
To get started with applying AI agents to automate data science workflows, consider the following steps:
- Identify areas for automation: Determine which tasks in your workflow can be automated using AI Agents.
- Choose an AI Agent platform: Select a platform that meets your needs and provides the necessary tools and support.
- Train and deploy AI Agents: Train AI Agents on your dataset and deploy them to automate tasks.
- Monitor and evaluate performance: Monitor the performance of AI Agents and evaluate their impact on your workflow.
Best Practices
When working with AI Agents, it’s essential to follow best practices to ensure success:
- Data Quality: Ensure that your data is high-quality and well-formatted to enable accurate predictions.
- Model Selection: Select the most suitable model for your task, taking into account factors like complexity and interpretability.
- Continuous Monitoring: Continuously monitor the performance of AI Agents and update them as needed.
Conclusion
By leveraging AI Agents and understanding their role in data science, organizations can streamline their workflows, improve efficiency, and drive business success. Whether you’re working with companies like Databricks or exploring new applications of AI Agents, the possibilities are endless. As the field continues to evolve, we can expect to see even more innovative applications of AI Agents in Data Science.
The future of Data Science is exciting and rapidly evolving, and AI Agents are at the forefront of this evolution. By applying AI agents to automate data science workflows and following best practices, you can unlock new insights and drive business success. Remember to continuously monitor the performance of AI Agents and update them as needed to ensure optimal results.
Keywords: AI Agents, Data Science, Workflows
LSI terms: Applying AI agents to automate data science workflows, Understanding the role of AI agents in data science
Entities: Databricks
In addition to the technical aspects, it’s essential to consider the business implications of using AI Agents in data science. This includes:
- Return on Investment (ROI): Evaluate the potential ROI of implementing AI Agents in your workflow.
- Resource Allocation: Determine the resources required to implement and maintain AI Agents.
- Change Management: Develop a plan to manage the changes that come with implementing AI Agents.
By considering these factors, you can ensure a successful implementation of AI Agents in your data science workflow. The potential benefits are significant, and by following best practices and understanding the role of AI agents in data science, you can drive business success and stay ahead of the competition.
As the field continues to evolve, we can expect to see new developments and innovations in AI Agents for data science. Some potential areas of research include:
- Multi-Agent Systems: The development of systems that enable multiple AI Agents to work together to solve complex tasks.
- Explainable AI: The development of techniques to explain and interpret the decisions made by AI Agents.
These advancements will further enhance the capabilities of AI Agents in data science, enabling organizations to drive even more significant business success. By staying up-to-date with the latest developments and advancements, you can ensure that your organization remains at the forefront of innovation and continues to drive business success.
In conclusion, AI Agents have the potential to revolutionize the field of data science, enabling organizations to streamline their workflows, improve efficiency, and drive business success. By understanding the role of AI agents in data science, following best practices, and considering the business implications, you can unlock new insights and drive business success. The future of Data Science is exciting and rapidly evolving, and AI Agents are at the forefront of this evolution.