Behavioral Insights from Generative AI Models
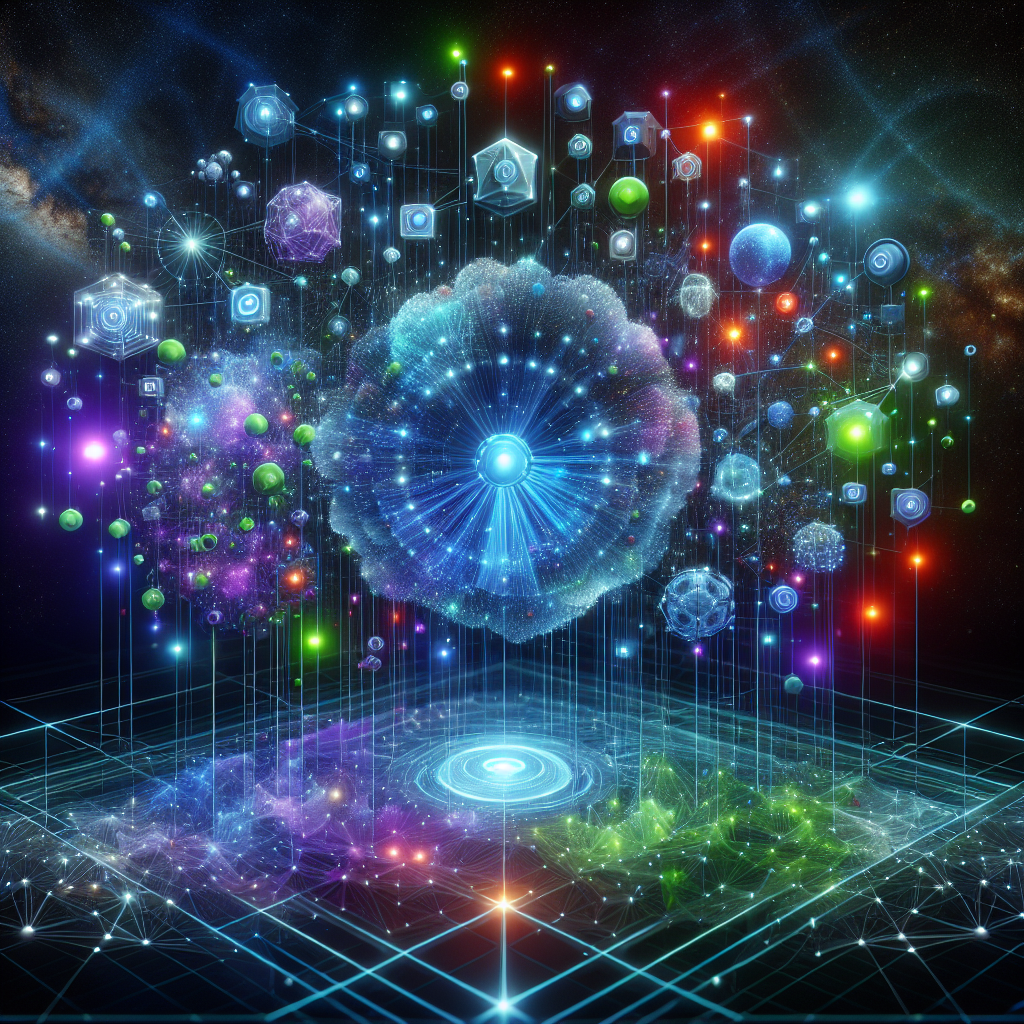
Picture a world where artificial intelligence seamlessly blends into the fabric of business operations and decision-making—a world akin to standing on the cusp of a technological revolution. Much like pioneers at the dawn of Silicon Valley’s golden age, today’s business leaders have an equally transformative opportunity. Generative AI models are not just futuristic tools; they’re here, reshaping how we understand human-like patterns in data, unlocking new efficiencies, and driving innovation.
In this narrative journey, we’ll explore behavioral insights gleaned from leading generative AI models like those developed by OpenAI. We’ll delve into their ethical implications, and provide actionable strategies for businesses keen on navigating this exciting frontier. Imagine your business as a modern-day tech pioneer, ready to harness the potential of these advanced systems.
The Dawn of Generative AI: A Historical Context
The story of generative AI is one of rapid evolution and boundless potential. Emerging from research labs in the early 2010s, models like OpenAI’s GPT series began with modest capabilities but quickly outpaced their predecessors. These advancements were fueled by a growing understanding of neural networks and deep learning techniques, allowing machines to not only process data but also generate content that mirrors human creativity.
Generative AI has since transcended academic circles, making its mark in industries ranging from marketing to healthcare. Consider how OpenAI’s GPT-3 transformed customer service interactions by providing instant, nuanced responses—revolutionizing the way businesses engage with their clientele. This shift underscores a broader trend: companies are increasingly relying on AI not just for operational efficiency but also as a creative partner.
Criteria for Evaluation
When evaluating generative AI models, imagine standing at the helm of an exploration vessel, assessing its capabilities with a discerning eye. Here are essential criteria:
- Human-Like Pattern Recognition: Consider how effectively these digital explorers mimic human behavior and thought processes.
- Ethical Decision-Making: Reflect on the ethical implications woven into their decision-making fabric.
- Business Application Readiness: Assess whether they’re ready to seamlessly integrate into your business workflows.
Understanding Human-Like Patterns
Generative AI models are designed to replicate and understand patterns in human behavior. This ability is crucial for applications like personalized marketing, where understanding consumer preferences can lead to more effective campaigns. For instance, OpenAI’s GPT-3 has been instrumental in crafting tailored content that resonates with diverse audiences, demonstrating an almost uncanny ability to predict user needs.
The concept of “understanding human-like patterns” extends beyond mere data analysis; it involves interpreting the subtleties and nuances of human communication. Google’s BERT excels here, particularly in sentiment analysis tasks where context is key. By dissecting the intricacies of language, these models offer businesses insights that were previously inaccessible.
Ethical Considerations in AI Development
Ethics form the backbone of responsible AI development. As generative models become more sophisticated, so too do the ethical challenges they present. OpenAI, for example, has implemented rigorous testing to minimize biases in its models, acknowledging that unchecked biases can perpetuate societal inequalities.
The journey towards ethical AI is ongoing and complex. It involves continuous monitoring and updating of algorithms to ensure fairness and transparency. Companies must adopt a proactive approach, integrating ethical guidelines into every stage of AI development. This commitment not only safeguards against potential misuse but also builds trust with consumers who are increasingly concerned about data privacy and algorithmic accountability.
Business Application Readiness: A Pragmatic Approach
For businesses, the readiness of an AI model to be integrated into existing workflows is paramount. OpenAI’s GPT series stands out for its adaptability across various industries, from finance to entertainment. Its ability to generate coherent text makes it a versatile tool for content creation and customer interaction.
However, not all models are created equal in terms of business readiness. Stanford University’s transformer models, while pioneering in research, often require additional development before they can be effectively deployed in commercial settings. Businesses must weigh the potential benefits against the time and resources needed to tailor these models to their specific needs.
Detailed Comparison: Leading Generative AI Models
1. OpenAI’s GPT Series
Human-Like Pattern Recognition
OpenAI’s GPT series, akin to master storytellers, excels in creating narratives and engaging dialogues that seem strikingly human. These models set benchmarks in generating coherent text patterns.
- Pros: Exceptional output quality across various industries, broad applicability.
- Cons: Potential for biased or inappropriate content if not carefully monitored.
Ethical Decision-Making
OpenAI is deeply invested in ethical AI development, striving to mitigate biases and prevent unintended language generation consequences. However, the journey towards a bias-free future is ongoing and requires vigilance.
2. Google’s BERT
Human-Like Pattern Recognition
BERT shines when understanding context within text, making it indispensable for sentiment analysis and information retrieval tasks. Its ability to decipher nuances in human communication is unmatched.
- Pros: Superior contextual comprehension.
- Cons: Less flexible than GPT models in generating novel content.
Ethical Decision-Making
Google prioritizes transparency and fairness in AI development, addressing ethical concerns proactively. However, issues like data privacy and bias remain complex challenges to overcome.
3. Stanford University’s Transformer Models
Human-Like Pattern Recognition
Stanford researchers have been at the forefront of transformer architecture development, focusing on efficiency and scalability. Their work underpins many cutting-edge generative models.
- Pros: Pioneering research leading to innovative applications.
- Cons: Often in early stages of development, making them less ready for immediate business application.
Ethical Decision-Making
Stanford’s collaborative projects emphasize ethical AI practices. The open-source nature invites community scrutiny and continuous improvement, reinforcing their commitment to responsible AI use.
Recommendations for Different Use Cases
Customer Engagement Platforms
- Recommendation: Deploy OpenAI’s GPT models to craft engaging, personalized customer interactions.
- Rationale: Their advanced language generation capabilities create realistic dialogue simulations that enhance user experience.
Sentiment Analysis in Marketing
- Recommendation: Utilize Google’s BERT model for deep insights into consumer sentiment from social media and reviews.
- Rationale: Its contextual understanding excels in analyzing nuanced human emotions expressed through text.
Academic and Research Applications
- Recommendation: Explore Stanford’s transformer models for academic research requiring innovative AI solutions.
- Rationale: Their pioneering contributions provide a robust foundation for experimental applications and collaborative studies.
Case Studies: Generative AI in Action
OpenAI’s GPT Series Transforming Content Creation
A leading e-commerce platform leveraged OpenAI’s GPT-3 to enhance its content creation process. By automating product descriptions, the company not only increased efficiency but also improved customer engagement through personalized narratives. This case study highlights how generative AI can drive both operational excellence and consumer satisfaction.
Google’s BERT Enhancing Customer Support
A global telecommunications provider implemented Google’s BERT to refine its customer support systems. By analyzing customer inquiries with greater accuracy, the company reduced response times and increased resolution rates. This success story underscores the power of contextual understanding in improving service quality.
Industry Trends and Future Predictions
The future of generative AI is bright, with advancements expected to revolutionize numerous sectors. As these models become more sophisticated, we can anticipate:
- Increased Personalization: Businesses will harness AI to deliver hyper-personalized experiences, from marketing campaigns to product recommendations.
- Ethical AI Governance: The establishment of global standards for ethical AI use will likely accelerate, driven by both regulatory bodies and industry leaders.
- Cross-Disciplinary Applications: Generative AI will find new applications in fields like healthcare, where it can assist in diagnosing diseases or developing personalized treatment plans.
Frequently Asked Questions
What are the ethical implications of using generative AI?
Generative AI can inadvertently perpetuate biases present in training data. It’s crucial to implement robust monitoring systems, ensuring outputs remain fair and unbiased.
How do businesses benefit from understanding human-like patterns in AI?
Analyzing how AI mimics human behavior allows businesses to optimize customer interactions, enhance user engagement, and develop intuitive AI-driven products.
Are generative AI models ready for immediate integration into business operations?
While some models like OpenAI’s GPT series are well-suited for immediate deployment, others may require further development or customization to meet specific business needs effectively.
How can companies ensure ethical use of generative AI?
Establishing clear guidelines prioritizing transparency, fairness, and accountability is crucial. Collaborating with academic institutions like Stanford University can also offer valuable insights into best practices.
What role does the San Francisco Bay Area play in advancing generative AI research?
The region remains a hub for innovation, home to leading tech companies and research institutions that drive advancements in AI technology and ethical considerations.
Ready to Transform Your Business with AI?
As pioneers at the forefront of AI-driven business solutions, we’re committed to empowering organizations by providing cutting-edge AI Agentic software development and AI Cloud Agents services. Leveraging insights discussed here, we’ve helped numerous companies across industries integrate generative AI solutions tailored to their unique needs.
Whether your goal is enhancing customer engagement or optimizing operational efficiency, our expertise can guide you through this transformative journey. Contact us today for a consultation, and let us demonstrate how these concepts can be practically applied within your organization. Use the contact form on this page to easily communicate with our team—we are more than happy to field any questions and provide assistance throughout your AI transformation process.
Together, we can unlock new possibilities for innovation and growth in your business landscape, ensuring you remain a pioneer in an ever-evolving digital world.