Boosting Agent Intelligence through Effective Learning
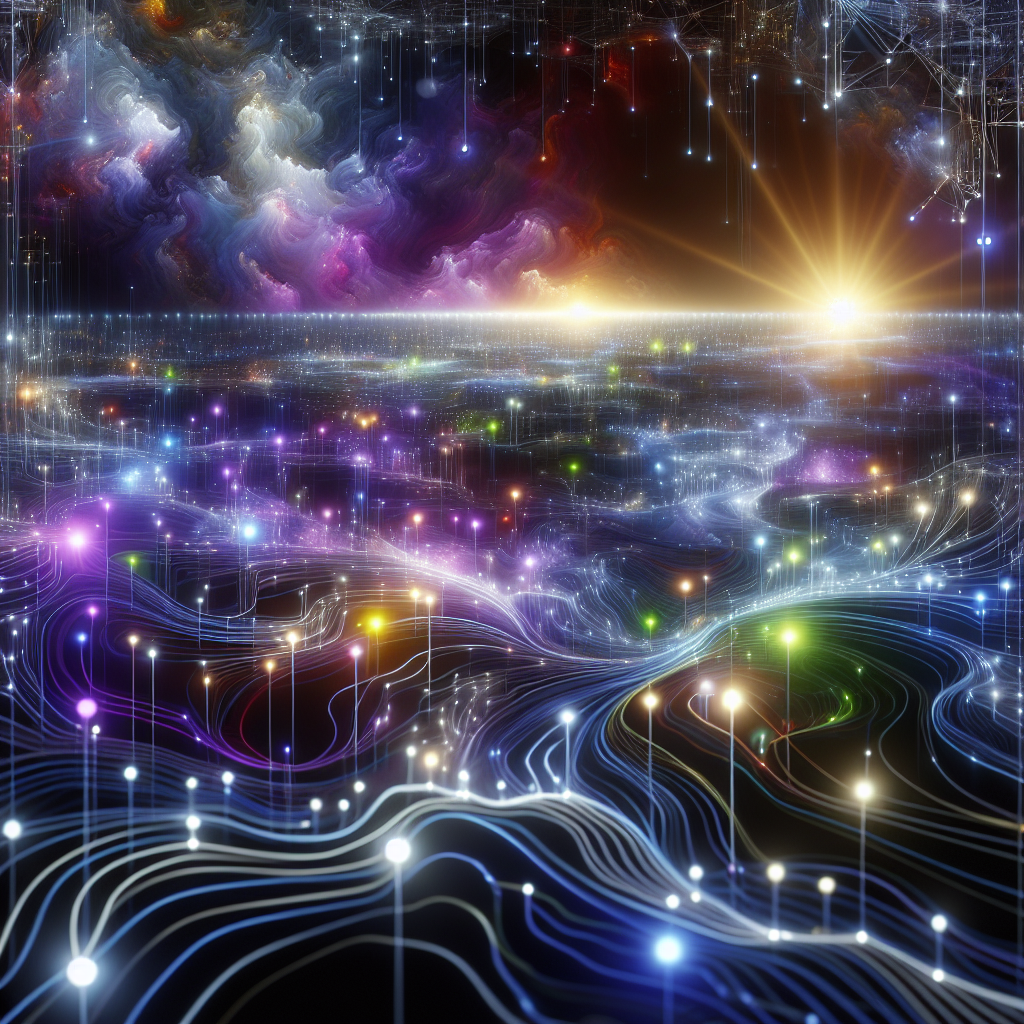
In today’s rapidly evolving technological landscape, artificial intelligence (AI) agents have become integral components across various industries. From automating mundane tasks to making complex decisions, AI-driven systems are reshaping how businesses operate. Enhancing agent intelligence is a continuous journey that requires effective learning strategies and advanced algorithms. This blog post explores how machine learning techniques can be harnessed to improve the decision-making capabilities of artificial intelligence through continuous learning processes.
Introduction
Artificial Intelligence (AI) agents are increasingly being employed in diverse applications, ranging from customer service bots to autonomous vehicles. The effectiveness of these systems largely depends on their ability to learn, adapt, and make informed decisions. Enhancing agent intelligence involves more than just feeding data; it requires creating robust learning environments that enable continuous improvement.
In this post, we delve into various strategies for enhancing AI agent intelligence. We focus on machine learning techniques, effective training methodologies, and the implementation of advanced algorithms. Insights from leading institutions like Google DeepMind, OpenAI, and Stanford University provide a comprehensive understanding of how to boost AI agent intelligence through effective learning.
The Importance of Continuous Learning in AI
Improving Decision-Making Capabilities through Continuous Learning Processes
Continuous learning is pivotal for improving decision-making capabilities of artificial intelligence. It ensures that AI systems remain relevant and adaptable by allowing them to adjust to new scenarios and challenges. This adaptability enhances real-time data processing and adaptive learning models, significantly improving the responsiveness of AI-driven applications.
Implementing Advanced Algorithms to Enhance Adaptability
Advanced algorithms, such as deep learning and reinforcement learning, play a crucial role in enhancing the adaptability and responsiveness of AI agents. These techniques enable AI systems to recognize patterns, learn from past experiences, and apply knowledge across different domains effectively. By doing so, they enhance the overall capability of AI agents to respond to new situations.
Case Studies: Successful Implementations
To better understand the impact of continuous learning, consider case studies where businesses have successfully integrated these methodologies:
- Healthcare: AI systems in healthcare are increasingly used for diagnostics and treatment recommendations. Continuous learning allows these systems to integrate the latest medical research, improving their diagnostic accuracy over time.
- Finance: In finance, AI-driven trading algorithms benefit from continuous learning by adapting to market changes quickly, providing more accurate predictions and better risk management.
The Role of Big Data in Continuous Learning
Big data plays a crucial role in enhancing AI agent intelligence. By leveraging vast datasets, AI systems can refine their models continuously, ensuring they remain relevant with evolving trends and information. This is particularly significant in industries where the volume of data is immense and constantly growing, such as e-commerce and social media.
Effective Training Strategies for Intelligent Systems
Structured Learning Environments
Creating structured learning environments is essential for effective training strategies for intelligent systems. These environments provide a systematic approach that helps in developing robust models capable of handling diverse challenges. They foster an atmosphere where AI agents can learn efficiently and effectively. For instance, using hierarchical reinforcement learning structures allows AI to understand tasks at different levels of complexity.
Simulation-Based Learning
Simulation-based learning allows AI agents to practice skills in a virtual environment before applying them in real-world scenarios. This is particularly beneficial for training autonomous vehicles or robotics, where mistakes in the real world can be costly. By simulating various conditions and environments, AI systems gain valuable experience without risk.
Incremental Task Complexity
Gradually increasing task complexity helps AI agents build upon their learning incrementally. Starting with simpler tasks allows them to establish a foundation of knowledge before moving on to more complex challenges. This approach mimics human learning processes and has proven effective in enhancing the capabilities of AI systems, especially in educational technology applications.
Leveraging Expert Systems
Expert systems store vast amounts of domain-specific knowledge that can be used to train AI agents. By incorporating these systems into the training process, AI can learn from accumulated expertise and apply it in relevant contexts. This is particularly useful in fields like law and medicine, where specialized knowledge is crucial.
Collaborative Learning with Human Experts
Incorporating human feedback into the learning process can significantly enhance AI agent intelligence. Through collaborative learning, AI systems benefit from human intuition and decision-making skills. This approach also ensures that AI aligns with ethical standards and societal norms, which are often best guided by human judgment.
The Future of Agent Intelligence Enhancement
Emerging Technologies and Trends
As technology advances, new methodologies for enhancing AI agent intelligence continue to emerge. Techniques such as federated learning, where models are trained across decentralized devices while keeping data localized, offer promising avenues for privacy-preserving machine learning. Additionally, the integration of quantum computing could revolutionize how AI processes information by exponentially increasing computational power.
The Intersection with Other AI Domains
The enhancement of agent intelligence is closely linked with advancements in other AI domains such as natural language processing (NLP) and computer vision. Improvements in these areas can significantly boost an agent’s ability to understand and interact with the world, leading to more sophisticated applications across industries.
Ethical Considerations and Responsibilities
As AI agents become more intelligent, ethical considerations must be addressed. Ensuring that AI systems operate transparently, fairly, and without bias is crucial. Moreover, developers have a responsibility to consider the societal impact of these technologies and work towards solutions that benefit humanity as a whole.
Conclusion
Enhancing agent intelligence through effective learning strategies is essential for maximizing the potential of AI in various industries. By leveraging continuous learning processes, advanced algorithms, and collaborative approaches, we can develop intelligent systems capable of adapting to new challenges and improving decision-making capabilities over time. Insights from leading institutions like Google DeepMind, OpenAI, and Stanford University continue to guide these advancements, ensuring that AI technology evolves responsibly and effectively.
Frequently Asked Questions
What are some effective training strategies for enhancing AI agent intelligence?
Effective training strategies include structured learning environments, simulation-based learning, incremental task complexity, leveraging expert systems, and collaborative learning with human experts. These approaches help create robust models capable of handling diverse challenges.
How do advanced algorithms enhance the adaptability of AI agents?
Advanced algorithms, such as deep learning and reinforcement learning, allow AI agents to recognize patterns, learn from experiences, and apply knowledge across different domains effectively. This enhances their ability to adapt to new situations.
What role does continuous learning play in improving decision-making capabilities?
Continuous learning ensures that AI systems remain relevant and effective by allowing them to adapt to new scenarios and challenges. It enables real-time data processing and adaptive learning models, which improve the overall responsiveness of AI-driven applications.
How have institutions like Google DeepMind contributed to enhancing AI agent intelligence?
Google DeepMind has developed sophisticated algorithms such as deep reinforcement learning and created detailed simulated environments for training AI agents. These contributions have significantly improved the adaptability and decision-making capabilities of AI systems.
What ethical considerations should be taken into account when developing intelligent AI agents?
Ethical considerations include ensuring that AI advancements align with societal values, prioritizing transparency in AI processes, and considering potential impacts on privacy and security. Institutions like Stanford University emphasize these aspects to develop responsible AI technologies.