Boosting Efficiency with Automated Insights from Generative AI Models
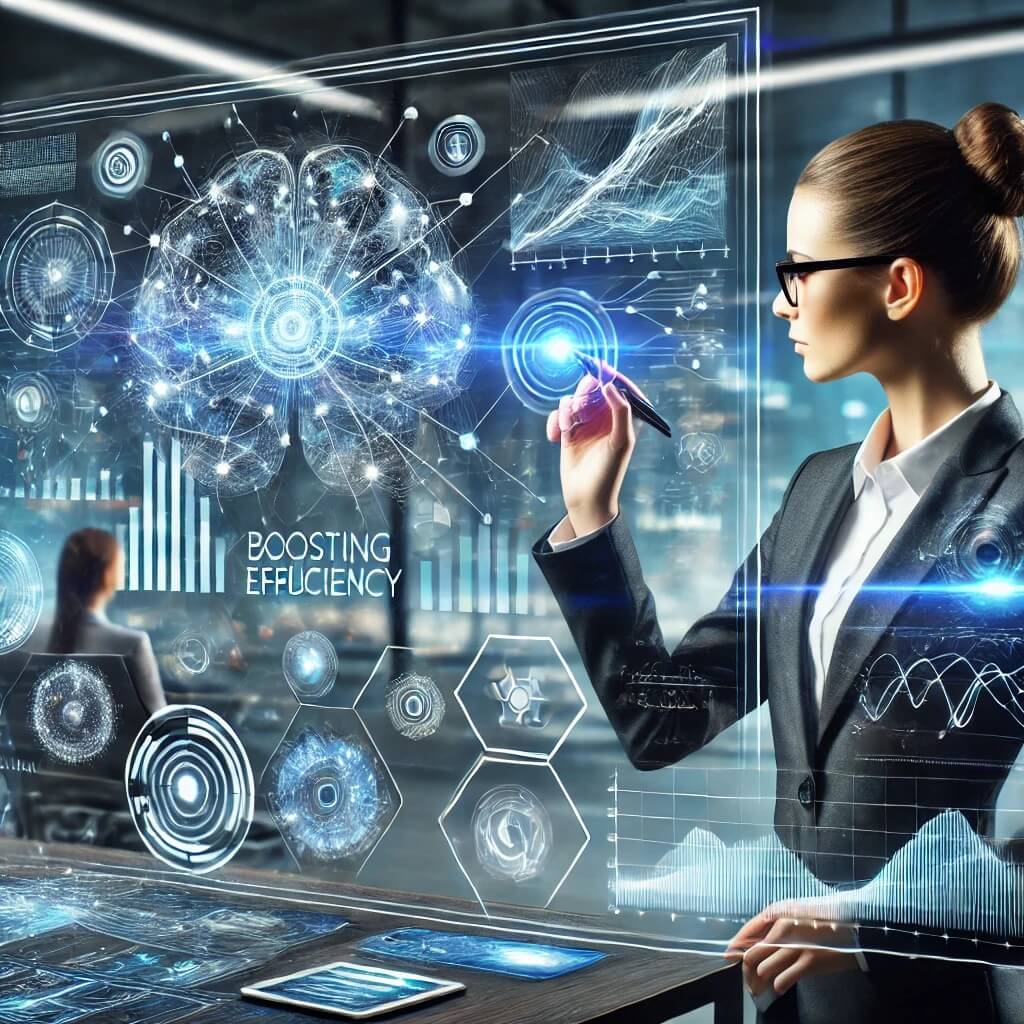
In today’s fast-paced business environment, efficiency is key to staying competitive and achieving success. As the volume and complexity of data continue to grow, organizations are under increasing pressure to make informed decisions quickly, while also reducing costs and improving resource allocation. However, manual insights generation, which has been a cornerstone of decision-making for decades, can no longer keep pace with these demands.
The Limits of Manual Insights Generation
Manual insights generation involves human analysts sifting through vast amounts of data, identifying patterns and relationships, and drawing conclusions based on their expertise and experience. While this approach has served businesses well in the past, it is often time-consuming, labor-intensive, and prone to errors and biases. As a result, many organizations are struggling to keep up with the demands of big data, leading to delays, inefficiencies, and missed opportunities.
The Need for Automated Insights
In recent years, advances in artificial intelligence (AI) have opened up new possibilities for automating insights generation. Generative AI models, which can process and analyze large datasets, identify patterns, and make predictions, are increasingly being used by businesses to gain a competitive edge. By leveraging these models, organizations can streamline their operations, reduce costs, and make data-driven decisions with greater accuracy and speed.
The Power of Generative AI
Generative AI models have the potential to revolutionize insights generation by providing business leaders with access to high-quality, actionable information in real-time. These models can process vast amounts of data, identify complex patterns, and make predictions that inform strategic decision-making. By automating insights generation, businesses can free up resources for more strategic activities, improve productivity, and drive growth.
The Focus of This Article
This article will explore the benefits of using generative AI models to automate insights generation in business. We will discuss the challenges associated with manual insights generation, the capabilities of generative AI models, and the benefits of implementing these models in organizational settings. Our aim is to provide business leaders with a comprehensive understanding of how generative AI can be used to boost efficiency and drive success.
The Challenges of Manual Insights Generation
Manual insights generation has been a cornerstone of business decision-making for decades, but it is no longer an effective approach in today’s fast-paced and data-rich environment. Despite its limitations, many businesses continue to rely on manual insights generation, often with suboptimal results.
Time-Consuming and Labor-Intensive
Manual insights generation involves human analysts sifting through vast amounts of data, identifying patterns and relationships, and drawing conclusions based on their expertise and experience. This process is time-consuming and labor-intensive, requiring significant resources to analyze and interpret large datasets. As a result, businesses often struggle to keep up with the demands of big data, leading to delays and inefficiencies.
Prone to Errors and Biases
Human analysts are prone to errors and biases when generating insights manually. These can arise from various sources, including:
- Confirmation bias: Analysts may be more likely to confirm their pre-existing views rather than seeking alternative perspectives.
- Availability heuristic: Analysts may overestimate the importance of information that is readily available, rather than seeking a broader range of data.
- Anchoring effect: Analysts may rely too heavily on initial impressions or data points, without fully considering other factors.
Limited Scalability
Manual insights generation is often limited in its scalability. As data volumes grow, it becomes increasingly difficult for human analysts to keep pace with the demands of big data. This can lead to:
- Delays: Businesses may struggle to respond quickly to changing market conditions or customer needs.
- Inefficiencies: Manual insights generation can be a resource-intensive process, requiring significant time and labor.
Costly Consequences
The consequences of manual insights generation can be costly for businesses. By failing to leverage the power of data-driven decision-making, organizations may:
- Miss opportunities: Businesses may miss out on growth opportunities or fail to capitalize on market trends.
- Make poor decisions: Manual insights generation can lead to poor decision-making, resulting in wasted resources and lost revenue.
The Need for Automated Insights
In response to these challenges, businesses are turning to automated insights generation using generative AI models. These models have the potential to revolutionize the way organizations make decisions, by providing high-quality, actionable information in real-time. By automating insights generation, businesses can:
- Improve efficiency: Automate manual processes and reduce resource consumption.
- Enhance decision-making: Provide accurate and reliable data-driven insights.
- Drive growth: Capitalize on market trends and opportunities.
What are Generative AI Models?
Generative AI models are a type of artificial intelligence (AI) that can process and analyze large datasets to identify patterns, relationships, and trends. These models have the ability to generate new data or insights based on their training data, making them particularly useful for tasks such as predictive modeling, clustering analysis, and anomaly detection.
Definition and Explanation
Generative AI models are typically trained on a dataset that is representative of the problem domain. The model uses this training data to learn patterns and relationships within the data, which it can then use to generate new insights or predictions. There are several types of generative AI models, including:
- Generative Adversarial Networks (GANs): These models consist of two neural networks that work together to generate new data samples that are similar to the training data.
- Variational Autoencoders (VAEs): These models use a combination of encoding and decoding layers to learn the underlying structure of the data, which can then be used for tasks such as dimensionality reduction and anomaly detection.
- Recurrent Neural Networks (RNNs): These models are designed for sequential data and have been successfully applied to tasks such as natural language processing, speech recognition, and time series prediction.
Capabilities
Generative AI models have several key capabilities that make them useful for a wide range of applications:
- Pattern recognition: Generative AI models can identify complex patterns within large datasets, which can be used for tasks such as predictive modeling and clustering analysis.
- Data generation: These models can generate new data samples that are similar to the training data, making them useful for tasks such as synthetic data creation and data augmentation.
- Feature learning: Generative AI models can learn features from raw data, which can then be used for tasks such as dimensionality reduction and anomaly detection.
Real-World Applications
Generative AI models have been successfully applied to a wide range of real-world applications, including:
- Image recognition: These models have been used in image recognition systems to identify objects within images.
- Speech recognition: Generative AI models have been used in speech recognition systems to recognize spoken words and phrases.
- Predictive modeling: These models have been used for predictive modeling tasks such as demand forecasting and stock price prediction.
The Power of Generative AI
Generative AI models have the power to revolutionize many industries by providing high-quality, actionable insights that can be used to drive business decisions. By leveraging these models, businesses can improve efficiency, enhance decision-making, and drive growth.
How Do Generative AI Models Automate Insights?
Generative AI models have the ability to automate insights by processing and analyzing large datasets, identifying patterns and relationships, and generating new data or predictions based on their training data.
Automated Pattern Recognition
Generative AI models use complex algorithms to identify patterns within large datasets. These models can recognize patterns that are difficult for humans to detect, such as anomalies, clusters, and correlations. By automating pattern recognition, generative AI models can provide insights into the underlying structure of the data, which can inform business decisions.
Automated Data Generation
Generative AI models have the ability to generate new data samples that are similar to the training data. This can be useful for tasks such as synthetic data creation and data augmentation, where additional data is needed to improve model performance or to simulate real-world scenarios.
Automated Feature Learning
Generative AI models can learn features from raw data, which can then be used for tasks such as dimensionality reduction and anomaly detection. By automating feature learning, generative AI models can reduce the need for human analysts to manually extract features from large datasets.
How Generative AI Models Work
Generative AI models work by using a combination of machine learning algorithms and data preprocessing techniques to transform raw data into a format that can be used to train the model. The model is then trained on this transformed data, which enables it to learn patterns and relationships within the data.
Key Steps in the Process
- Data collection: Raw data is collected from various sources.
- Data preprocessing: The raw data is preprocessed to transform it into a format that can be used for training.
- Model selection: A generative AI model is selected based on the type of problem being addressed and the characteristics of the data.
- Training: The model is trained on the transformed data using machine learning algorithms.
- Evaluation: The performance of the model is evaluated using metrics such as accuracy, precision, and recall.
The Benefits of Automating Insights with Generative AI
Automating insights with generative AI models has several benefits, including:
- Improved efficiency: By automating pattern recognition, data generation, and feature learning, businesses can improve efficiency and reduce the time and resources required to analyze large datasets.
- Enhanced decision-making: By providing high-quality, actionable insights, generative AI models can inform business decisions and improve outcomes.
- Increased accuracy: By reducing human bias and error, generative AI models can provide more accurate insights than human analysts.
Benefits of Automated Insights from Generative AI Models
Automated insights from generative AI models have several benefits that make them an attractive solution for businesses and organizations.
Improved Efficiency
One of the primary benefits of automated insights from generative AI models is improved efficiency. By automating pattern recognition, data generation, and feature learning, businesses can reduce the time and resources required to analyze large datasets. This allows analysts to focus on higher-level tasks that require human judgment and expertise, such as strategy development and decision-making.
Enhanced Decision-Making
Automated insights from generative AI models also provide enhanced decision-making capabilities. By providing high-quality, actionable insights, businesses can make more informed decisions that are based on data-driven analysis rather than intuition or guesswork. This leads to better outcomes and improved performance.
Increased Accuracy
Another benefit of automated insights from generative AI models is increased accuracy. By reducing human bias and error, these models provide more accurate insights than human analysts. This is particularly important in industries where small errors can have significant consequences, such as healthcare and finance.
Cost Savings
Automated insights from generative AI models can also lead to cost savings. By automating tasks that were previously performed by humans, businesses can reduce labor costs and improve productivity. Additionally, the accuracy and quality of automated insights can help reduce errors and rework, which can save significant amounts of money over time.
Scalability
Automated insights from generative AI models are also highly scalable. As data volumes grow, these models can be easily scaled up to handle larger datasets without requiring significant increases in resources or personnel. This makes them an attractive solution for businesses that need to analyze large datasets on a regular basis.
Real-Time Insights
Finally, automated insights from generative AI models provide real-time insights. By analyzing data as it is generated, these models can provide immediate feedback and recommendations to businesses and organizations. This allows them to respond quickly to changing market conditions or customer needs, which can be a significant competitive advantage.
Benefits for Specific Industries
Automated insights from generative AI models have specific benefits for various industries, including:
- Healthcare: Improved patient outcomes and reduced medical errors
- Finance: Enhanced risk management and improved investment decisions
- Retail: Increased sales and revenue through targeted marketing campaigns
- Manufacturing: Improved quality control and reduced production costs
Implementation and Integration of Generative AI Models
Implementing and integrating generative AI models into an organization’s existing infrastructure can be a complex task that requires careful planning and execution.
Pre-Implementation Steps
Before implementing a generative AI model, it is essential to:
- Define business objectives: Clearly articulate the goals and expectations of the project.
- Identify key stakeholders: Determine who will be impacted by the implementation and ensure their buy-in.
- Assess existing infrastructure: Evaluate the organization’s current systems, processes, and data management capabilities.
- Choose the right model: Select a generative AI model that aligns with the business objectives and is compatible with the existing infrastructure.
Implementation Steps
Once the pre-implementation steps are complete, the next step is to:
- Develop a implementation plan: Create a detailed plan outlining the scope, timeline, and resources required for the project.
- Prepare data: Clean, preprocess, and format the data for use with the generative AI model.
- Train and validate the model: Train the model on the prepared data and validate its performance using metrics such as accuracy, precision, and recall.
- Integrate the model into existing systems: Integrate the trained model into the organization’s existing infrastructure, ensuring seamless communication between the model and other systems.
Integration with Existing Systems
When integrating a generative AI model into an organization’s existing systems, it is essential to consider:
- Data integration: Ensure that the data used by the model is integrated with the organization’s existing data management systems.
- API integration: Integrate the model’s API with other systems and applications to enable seamless communication.
- Security and compliance: Implement robust security measures and ensure compliance with relevant regulations and standards.
Benefits of Implementation
The implementation of a generative AI model can bring numerous benefits, including:
- Improved decision-making: The model can provide data-driven insights that inform business decisions.
- Increased efficiency: The model can automate tasks, reducing the time and resources required for manual processing.
- Enhanced customer experience: The model can help organizations better understand their customers’ needs and preferences.
Challenges and Considerations
While implementing a generative AI model can bring numerous benefits, there are also challenges and considerations to be aware of, including:
- Data quality issues: Poor data quality can negatively impact the performance of the model.
- Regulatory compliance: Ensure that the organization complies with relevant regulations and standards when implementing a generative AI model.
- Model bias: The model may reflect biases present in the training data.
Conclusion
In conclusion, generative AI models have the potential to revolutionize various industries and aspects of our lives by providing high-quality, actionable insights that can be used to inform business decisions, improve efficiency, and enhance customer experiences.
Key Takeaways
- Generative AI models: Can process and analyze large datasets to identify patterns, relationships, and trends.
- Improved decision-making: Can provide data-driven insights that inform business decisions.
- Increased efficiency: Can automate tasks, reducing the time and resources required for manual processing.
- Enhanced customer experience: Can help organizations better understand their customers’ needs and preferences.
Implementation and Integration
Implementing and integrating generative AI models into an organization’s existing infrastructure can be a complex task that requires careful planning and execution. It is essential to define business objectives, identify key stakeholders, assess existing infrastructure, and choose the right model before implementing a generative AI model.
Benefits and Considerations
The benefits of using generative AI models include improved decision-making, increased efficiency, and enhanced customer experiences. However, there are also challenges and considerations to be aware of, such as data quality issues, regulatory compliance, and model bias.
Future Directions
As the field of generative AI continues to evolve, we can expect to see new applications and use cases emerge. Some potential future directions include:
- Increased adoption: Wider adoption of generative AI models across various industries.
- Improved performance: Advancements in model architectures and algorithms that lead to improved performance and accuracy.
- New applications: New applications and use cases for generative AI models, such as in healthcare, finance, and education.
Conclusion
In conclusion, generative AI models have the potential to revolutionize various industries and aspects of our lives. While there are challenges and considerations to be aware of, the benefits of using generative AI models make them an attractive solution for organizations looking to improve decision-making, increase efficiency, and enhance customer experiences.