Building Agile AI Systems Frameworks
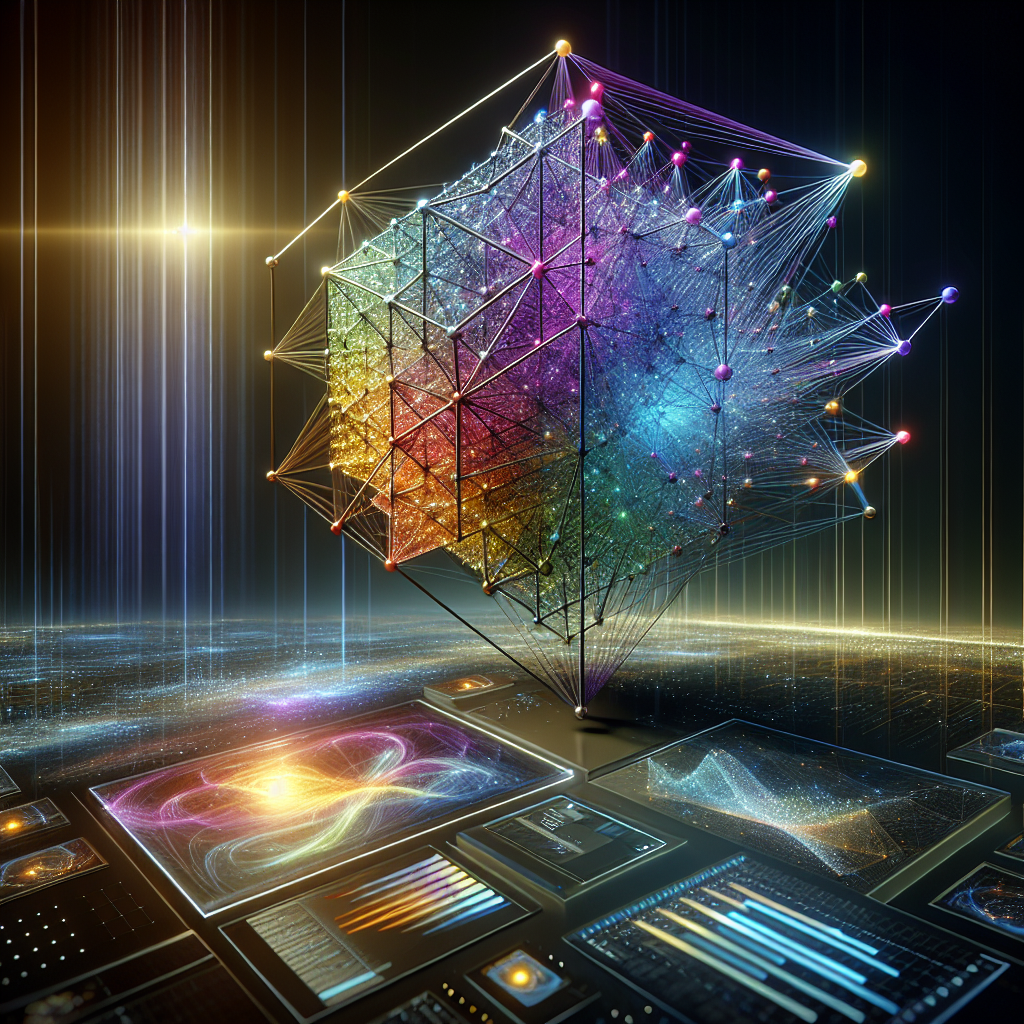
Introduction
The rise of AI has revolutionized industries worldwide, promising unprecedented efficiency and innovation. However, unlocking these benefits requires more than deploying machine learning models; it demands building agile AI systems that evolve with changing demands. According to Gartner, by 2024, 85% of enterprise-generated data will be processed outside traditional IT frameworks. This highlights the urgent need for adaptable solutions.
This article targets business professionals and decision-makers eager to implement cutting-edge AI technologies. By focusing on agile methodologies, scalable architectures, and seamless integration of machine learning models, you can transform your organization’s approach to AI. Additionally, understanding recent industry trends and future predictions will provide a broader context for the importance of agility in AI systems.
The Importance of Agile AI Development
Agile AI development leverages iterative processes and collaborative approaches to create flexible, adaptable AI systems. This methodology allows for continuous improvement and rapid adaptation to changing requirements, ensuring that AI solutions remain relevant over time.
Implementing Iterative Processes in AI System Design Enhances Flexibility
By adopting agile practices, businesses can design AI systems that are not only robust but also responsive to evolving business needs. Iterative processes enable teams to test and refine AI models continuously, leading to more accurate and effective outcomes. This iterative approach aligns with the core principles of agile software development, which emphasizes adaptability and rapid response to change.
Scalable AI Architectures: A Cornerstone for Growth
Scalable AI architectures empower businesses to handle increasing data volumes and computational demands without compromising performance. As organizations grow, their AI systems must be able to scale efficiently to accommodate new workloads and functionalities. This scalability ensures that AI solutions remain effective as the business evolves.
Integration of Machine Learning Models Within Agile Frameworks Ensures Adaptability
Integrating machine learning models within agile frameworks is crucial for maintaining adaptability. As market conditions change, businesses need AI systems that can pivot quickly to meet new demands. This integration allows organizations to update and refine their models without significant disruptions, ensuring continuous alignment with business goals.
Industry Trends and Future Predictions
The AI landscape is rapidly changing, driven by advancements in technology and shifts in consumer expectations. Several key trends are shaping the future of agile AI development:
- Increased Use of Automation: Automation technologies are becoming more sophisticated, enabling businesses to streamline operations and reduce manual intervention. Agile AI systems will play a crucial role in facilitating these automation efforts.
- Rise of Edge Computing: As data generation increases, edge computing is emerging as a solution for processing data closer to its source. This trend will influence how agile AI architectures are designed, emphasizing local processing capabilities alongside cloud integration.
- Focus on Ethical AI: With growing concerns about AI ethics and bias, businesses must prioritize transparency and fairness in their AI systems. Agile methodologies can help organizations quickly address ethical issues as they arise, ensuring responsible AI development.
- AI as a Service (AIaaS): The shift towards AIaaS models allows businesses to leverage advanced AI capabilities without significant upfront investment. This trend underscores the importance of scalable architectures that can be easily deployed and managed across various environments.
Case Studies and Examples
To illustrate the impact of agile AI development, let’s explore some real-world examples:
Google AI: Pioneering Agile Practices
Google AI has been at the forefront of implementing agile practices in AI development. By fostering a culture of innovation and collaboration, Google leverages iterative processes to refine its machine learning models continually. This approach allows the company to stay ahead of emerging trends and deliver cutting-edge solutions to its users.
Spotify Engineering Team: Agile Transformation
The Spotify engineering team has successfully transformed its operations through agile methodologies. By adopting an “agile” mindset, the team can quickly adapt to changes in user behavior and market conditions. This flexibility is crucial for maintaining Spotify’s competitive edge in the fast-paced music streaming industry.
Practical Advice for Implementing Agile AI Development
Implementing agile AI development requires a strategic approach that encompasses both technical and organizational aspects. Here are some actionable insights to guide your journey:
- Foster Cross-Functional Collaboration: Encourage collaboration between data scientists, engineers, and business stakeholders. This ensures that AI solutions are aligned with business objectives and can adapt quickly to changing needs.
- Invest in Continuous Learning: As AI technologies evolve rapidly, continuous learning is essential for staying ahead of the curve. Provide training opportunities for your team to keep their skills up-to-date and foster a culture of innovation.
- Embrace Iterative Development: Break down complex projects into smaller, manageable iterations. This allows teams to test and refine their models more frequently, leading to faster improvements and reduced risk.
- Prioritize Scalability from the Start: Design your AI architectures with scalability in mind. This proactive approach ensures that your systems can handle increased workloads as your business grows.
- Monitor and Evaluate Performance Continuously: Implement robust monitoring tools to track the performance of your AI models. Regular evaluations help identify areas for improvement and ensure that your solutions remain effective over time.
Conclusion
Building agile AI systems is essential for businesses seeking to thrive in today’s dynamic environment. By embracing iterative processes, scalable architectures, and seamless integration of machine learning models, organizations can create flexible, adaptable solutions that meet evolving demands.
As the AI landscape continues to evolve, staying informed about industry trends and future predictions will be crucial for maintaining a competitive edge. By following the insights and practical advice outlined in this guide, you can embark on an agile AI journey that unlocks new levels of innovation and efficiency.
Ready to take the next step? Contact us today to explore how our agile AI solutions can elevate your organization to new heights. Let’s build a future where technology empowers you to achieve your goals faster, smarter, and more effectively than ever before.
Frequently Asked Questions
What is Agile AI Development?
Agile AI development involves using iterative processes and collaborative approaches to create flexible and adaptable AI systems. This methodology allows for continuous improvement and quick adaptation to changing requirements.
How Do Scalable AI Architectures Benefit Businesses?
Scalable AI architectures enable businesses to handle increasing data volumes and computational demands without compromising performance, ensuring that their AI solutions remain efficient as they grow.
Why Is Integrating Machine Learning Models Within Agile Frameworks Important?
Integrating machine learning models within agile frameworks ensures adaptability. This allows AI systems to evolve alongside business needs and market changes, maintaining relevance and value over time.
What Challenges Might I Face in Implementing Agile AI Development?
Common challenges include resistance to change, lack of cross-functional collaboration, and insufficient resources for continuous learning. Addressing these issues early can help ensure a successful agile transformation.