Building Agile RAG Systems Today
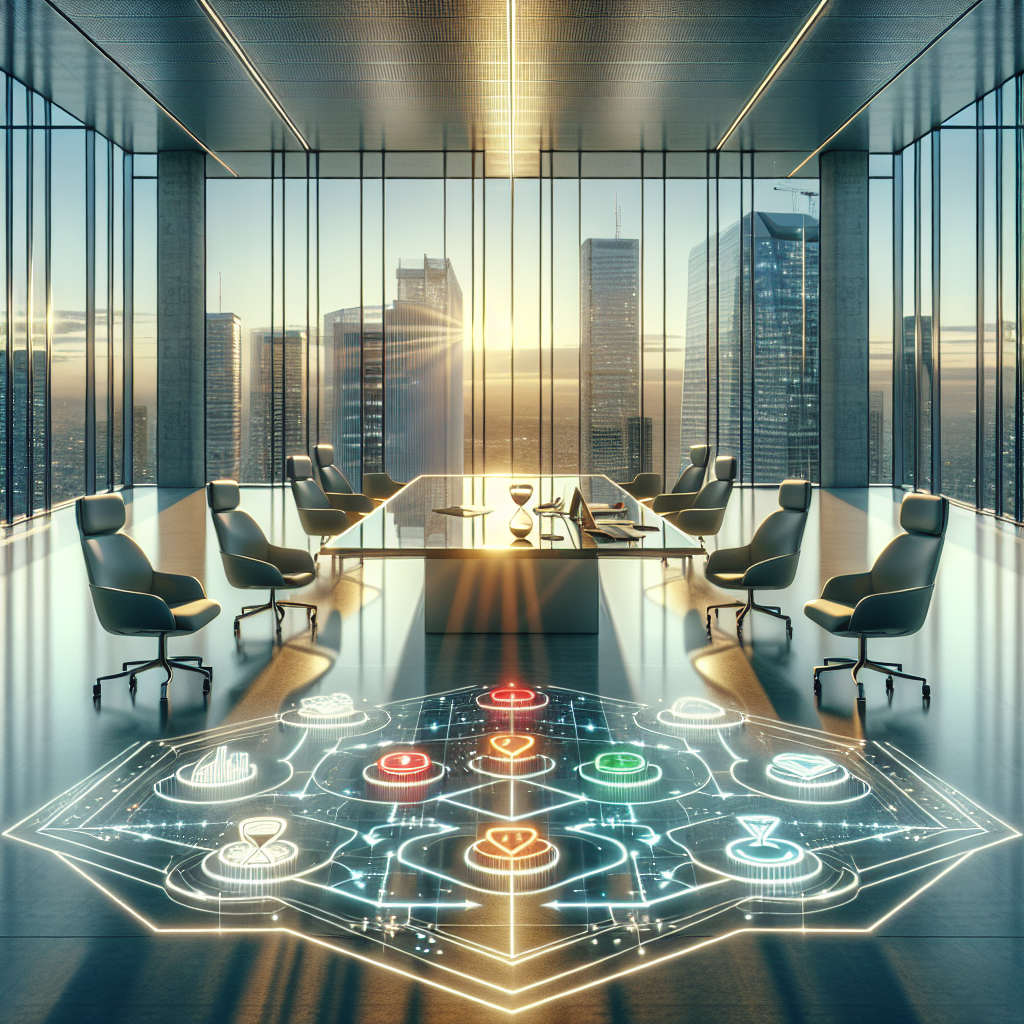
In today’s rapidly evolving digital landscape, businesses are under constant pressure to innovate and stay ahead of their competitors. According to a recent study by Forrester, 70% of businesses acknowledge that their rivals are more agile and innovative than they are, highlighting the critical need for advanced technology solutions like Retrieval-Augmented Generation (RAG) systems. This article delves into how integrating machine learning techniques, leveraging real-time data processing, and adopting agile development methodologies can enhance the capabilities of RAG systems, drawing on insights from leading tech entities such as Google AI Research and Microsoft Azure Cognitive Services.
Introduction: The Imperative for Agile RAG Systems
The integration of advanced technology solutions like Retrieval-Augmented Generation (RAG) systems is not just an option but a business imperative in today’s fast-paced digital economy. As companies strive to deliver personalized customer experiences, make data-driven decisions swiftly, and maintain operational efficiency, the role of agile development methodologies, machine learning techniques, and real-time data processing becomes increasingly crucial.
The growing complexity and volume of data have necessitated systems that can not only process information efficiently but also provide insights in a timely manner. RAG systems, which combine retrieval-based approaches with generative models, offer a unique advantage by enabling businesses to harness the power of vast datasets while generating relevant content or predictions on demand. As such, understanding and implementing agile RAG systems is essential for any business looking to remain competitive.
Proposing Machine Learning Integration to Enhance RAG Systems
The Power of Machine Learning in RAG Systems
Machine learning techniques are at the heart of enhancing RAG system capabilities. By incorporating advanced algorithms that can learn from data, these systems become more adept at analyzing vast amounts of information, identifying patterns, and making predictions with high accuracy. This integration not only improves the efficiency of data processing but also enriches the quality of insights generated, enabling businesses to make informed decisions swiftly.
Moreover, machine learning allows RAG systems to adapt over time, improving their performance as they encounter more data. This aspect is particularly valuable in industries where trends and consumer preferences evolve rapidly, such as fashion, technology, or finance.
Case Study: Google AI Research’s Approach
Google AI Research has been pioneering in applying machine learning techniques to enhance RAG systems. By leveraging neural networks and deep learning algorithms, Google has significantly improved the accuracy and relevance of search results, demonstrating the transformative potential of integrating machine learning with RAG technologies.
A notable project involved enhancing their search engine’s ability to provide contextually relevant information by retrieving documents from a vast index and generating summaries or answers based on user queries. This approach not only streamlined the search process but also provided users with more comprehensive insights, showcasing the power of machine learning in refining RAG system outputs.
Additional Insights: Machine Learning Techniques
To further illustrate the impact of machine learning on RAG systems, consider techniques such as natural language processing (NLP) and computer vision. NLP enables machines to understand human language, making it possible for RAG systems to process textual data more effectively. Meanwhile, computer vision allows these systems to interpret visual information, expanding their applicability across different sectors.
Mapping Real-Time Data Processing Advantages for Business Operations
The Impact of Real-Time Data on Operational Efficiency
Real-time data processing is a game-changer for businesses seeking to enhance operational efficiency. By analyzing data as it’s generated, companies can respond immediately to emerging trends, customer feedback, and market changes. This capability allows organizations to make swift adjustments to their strategies, ensuring they remain aligned with current demands.
Incorporating real-time data into RAG systems further amplifies these benefits by providing up-to-the-minute insights that are critical for decision-making processes. For instance, in the e-commerce sector, real-time analysis of customer behavior can inform targeted marketing efforts and inventory management decisions.
Case Study: Microsoft Azure Cognitive Services
Microsoft Azure Cognitive Services exemplify how real-time data processing can be integrated into RAG systems to enhance business operations. By offering a suite of AI services that enable developers to build intelligent applications without having direct AI or data science expertise, Azure has empowered businesses across industries to leverage real-time insights.
For example, Azure’s Text Analytics API can process large volumes of text in real time, providing sentiment analysis and key phrase extraction. When integrated into RAG systems, these capabilities allow companies to monitor brand perception continuously and adjust their customer engagement strategies accordingly.
Adopting Agile Development Methodologies for RAG System Implementation
The Role of Agile in RAG System Development
Agile development methodologies emphasize flexibility, collaboration, and incremental progress. Applying agile principles to the development of RAG systems ensures that these complex technologies can be adapted quickly to meet evolving business needs and technological advancements.
By breaking down the development process into manageable sprints, teams can focus on delivering specific features or improvements in a timely manner. This approach not only accelerates the deployment of RAG systems but also facilitates continuous feedback and refinement based on user experiences and changing requirements.
Practical Advice for Implementing Agile Methodologies
To effectively implement agile methodologies in RAG system development, businesses should consider forming cross-functional teams that include members from IT, data science, and business units. This collaboration fosters a holistic understanding of the project goals and challenges, ensuring that the developed systems align with organizational objectives.
Additionally, embracing tools and platforms that support agile practices can enhance communication and coordination among team members. Regular sprint reviews and retrospectives are also crucial for assessing progress and identifying areas for improvement.
Future Trends and Predictions in RAG Systems
As we look to the future, several trends are likely to shape the development and application of RAG systems:
- Increased Personalization: With advancements in machine learning and data analytics, RAG systems will become more adept at providing personalized content and recommendations, enhancing user engagement across various platforms.
- Integration with IoT Devices: The proliferation of Internet of Things (IoT) devices presents opportunities for RAG systems to process real-time data from a multitude of sources, offering insights that can drive innovation in sectors such as healthcare, transportation, and smart cities.
- Enhanced Security Measures: As RAG systems handle increasingly sensitive information, implementing robust security protocols will be paramount to protect against data breaches and ensure user privacy.
- Ethical Considerations: The ethical use of AI and machine learning in RAG systems will continue to be a critical focus area, with businesses needing to address concerns related to bias, transparency, and accountability.
Conclusion
Building agile RAG systems today is more than just an advantage—it’s a strategic imperative for businesses aiming to thrive in the digital age. By integrating machine learning techniques, leveraging real-time data processing, and adopting agile development methodologies, organizations can enhance their operational efficiency, foster innovation, and deliver personalized experiences that resonate with customers.
As technology continues to evolve, staying informed about industry trends and future predictions will be essential for maintaining a competitive edge. With the insights and strategies outlined in this guide, business professionals and decision-makers are well-equipped to navigate the complexities of implementing agile RAG systems, ensuring their organizations remain at the forefront of technological innovation.