Can AI Replace Scientists | Facts and Myths
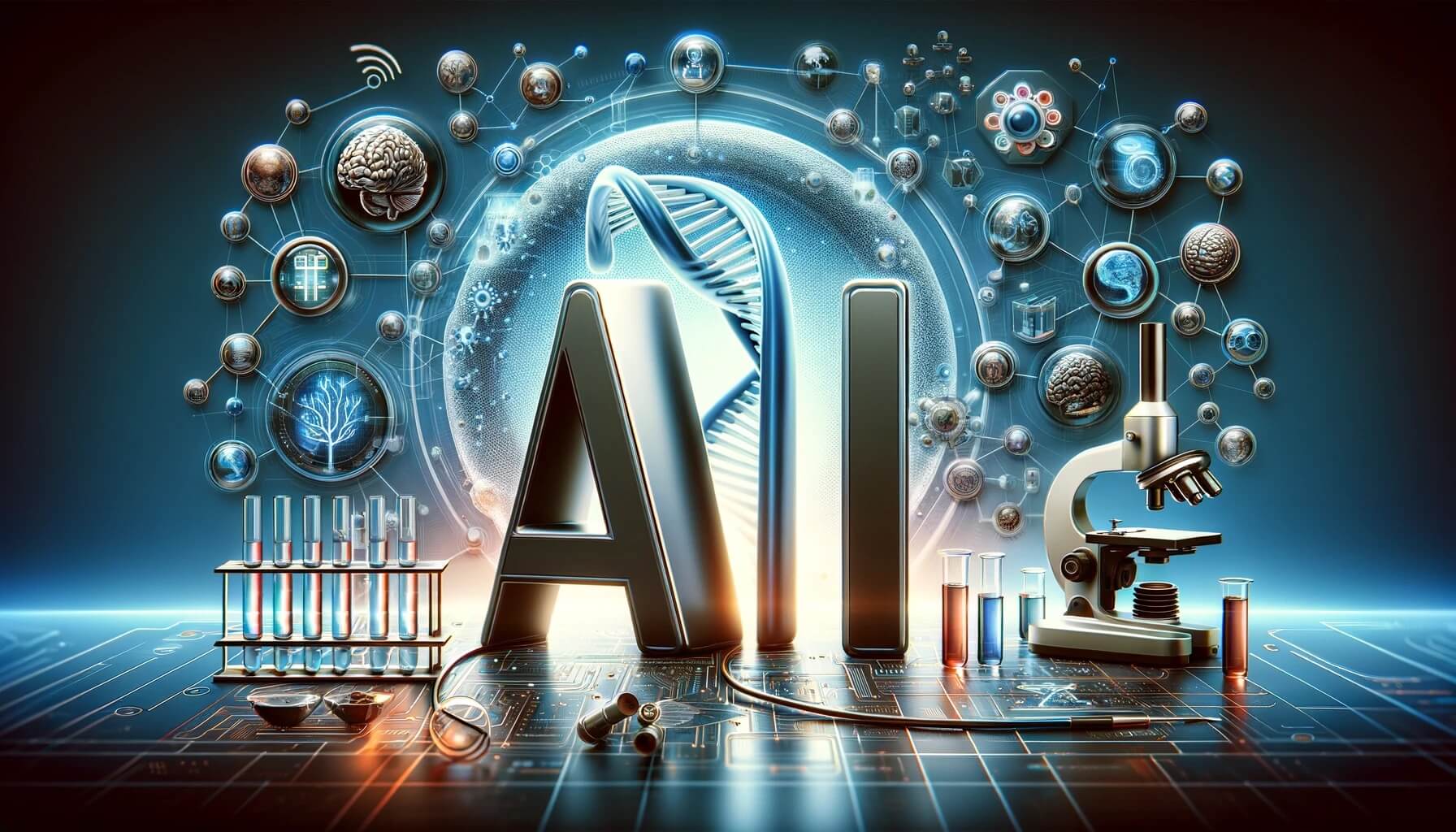
Introduction
In recent years, artificial intelligence (AI) has progressed from a promising technological frontier to a transformative force across myriad industries. The current capabilities of AI span from automating routine tasks to facilitating groundbreaking discoveries in fields as diverse as healthcare, finance, environmental science, and beyond. This rapid evolution is powered by advances in machine learning, neural networks, generative AI, and large language models (LLM) like ChatGPT, which have enabled machines to analyze complex data, predict outcomes, and even generate human-like text.
The impact of AI on various industries has been profound. In healthcare, AI algorithms process vast datasets to diagnose diseases earlier and with greater accuracy than ever before. In finance, machine learning models predict market trends and automate trading strategies, revolutionizing the sector’s approach to investment. Environmental scientists use AI to model climate change scenarios and develop more effective conservation strategies. These examples underscore AI’s potential to augment human capabilities, streamline operations, and unlock new avenues for innovation.
However, as AI’s capabilities continue to expand, so does the debate around its potential to replace human roles. The discourse is particularly intense in the realm of scientific research, where the very essence of discovery and innovation is at stake. Proponents argue that AI can accelerate research processes, analyze data with unparalleled precision, and even pioneer new scientific fields. Critics, however, caution against overreliance on AI, citing concerns over the loss of human intuition, creativity, and the ethical implications of autonomous scientific decision-making.
The question of whether AI can replace scientists touches on fundamental aspects of both AI and human intellect. It raises issues of alignment—ensuring AI technologies work towards human goals and values—and of the intrinsic qualities that define scientific inquiry. While AI can process and generate data at an extraordinary scale and speed, the depth of understanding, critical thinking, and creative insight that scientists bring to their work poses a complex challenge to the notion of replacement.
As we delve into this debate, it’s essential to distinguish between AI’s role as a tool that enhances scientific research and the more speculative view of AI as a standalone entity capable of supplanting human scientists. The nuanced reality likely lies somewhere in between, with AI and human scientists collaborating in ways that leverage the strengths of each to push the boundaries of what’s possible in scientific exploration and discovery.
The Rise of AI in Science
The integration of artificial intelligence (AI) and machine learning (ML) into scientific research represents one of the most significant shifts in how the scientific community approaches discovery and innovation. At its core, AI encompasses a broad range of technologies designed to emulate human intelligence, including reasoning, learning, and problem-solving, through the use of algorithms and neural networks. Machine learning, a subset of AI, focuses on the ability of machines to learn from and interpret data without being explicitly programmed for each task. This dynamic duo of AI and ML has begun to play a pivotal role in scientific research, offering tools and insights that were previously beyond reach.
Overview of Artificial Intelligence and Machine Learning in Scientific Research
AI and ML’s roles in scientific research are manifold and transformative. By harnessing vast amounts of data, these technologies can identify patterns and insights at a speed and scale unattainable by human researchers alone. Neural networks, which mimic the structure and function of the human brain, enable machines to learn from data iteratively, improving their accuracy over time. This capability is crucial for tasks ranging from analyzing complex genetic sequences to modeling climate change impacts.
Examples of AI Applications in Science
The applications of AI in science are as diverse as the fields of study themselves, each showcasing the potential of AI to revolutionize traditional methodologies:
- Data Analysis and Management: In fields like genomics and epidemiology, AI algorithms process and analyze large datasets to identify disease patterns, genetic markers, and potential treatment paths. This ability to sift through and make sense of vast data sets is changing the landscape of medical research and public health.
- Predictive Modeling: AI excels at predictive modeling, using historical data to forecast future events. In environmental science, AI models predict climate change effects, such as temperature fluctuations and sea-level rise, informing mitigation and adaptation strategies. Similarly, in physics, AI helps model complex systems and phenomena, pushing the boundaries of what is known about the universe.
- Automation of Routine Tasks: Many scientific endeavors require repetitive, time-consuming tasks that are essential but not intellectually stimulating. AI and ML automate these tasks, from sorting through astronomical images to identify celestial bodies to scanning electron microscope images for material defects. This automation allows scientists to devote more time to creative and analytical processes, thereby accelerating the pace of research and discovery.
The rise of AI in science is not about replacing human intellect but augmenting it. By taking over labor-intensive and computationally demanding tasks, AI frees scientists to engage more deeply with the conceptual and innovative aspects of their work. This collaboration between human and artificial intelligence is paving the way for breakthroughs that were once considered impossible, demonstrating that the true potential of scientific research is unlocked when human ingenuity and AI’s capabilities converge.
Key Technologies Behind AI’s Advancement
Neural Networks and Machine Learning
The surge of artificial intelligence (AI) in transforming industries and scientific research largely hinges on two pivotal technologies: neural networks and machine learning. These technologies form the backbone of AI’s ability to learn, adapt, and make decisions, mirroring some aspects of human cognition. Understanding neural networks and machine learning is essential to grasp the extent of AI’s capabilities and its potential to complement or even augment human roles in various fields, including science.
Neural Networks: Mimicking Human Brain Functionality
Neural networks are inspired by the biological neural networks that constitute animal brains. At their core, they are algorithms designed to recognize patterns by interpreting sensory data through a kind of machine perception, labeling, or clustering of raw input. The architecture of a neural network is layered, consisting of an input layer, one or more hidden layers, and an output layer. Each layer is made up of units or neurons, which process inputs and pass their output to the next layer. The strength and the connection between these neurons are adjusted through learning, much like how synapses strengthen in the human brain to form memories or skills.
The significance of neural networks in AI development cannot be overstated. They are the foundation of deep learning technologies, enabling machines to process complex data structures and perform tasks that require human-like understanding, such as image and speech recognition, natural language processing, and even creative tasks like generating art and music.
Machine Learning: Learning from Data
Machine learning, a subset of AI, enables systems to learn from data, identify patterns, and make decisions with minimal human intervention. The effectiveness of machine learning algorithms improves with experience, utilizing training data through two primary approaches: supervised and unsupervised training.
- Supervised Training: In this method, the model learns from a labeled dataset. It means that each example in the training set is paired with the correct output. The algorithm makes predictions during training, and adjustments are made until the model achieves a high level of accuracy. Supervised learning is commonly used for classification and regression tasks, such as identifying spam emails or predicting house prices.
- Unsupervised Training: Unlike supervised training, unsupervised learning involves working with datasets without labeled responses. The goal here is to explore the data and find some structure within. It’s used for clustering, association, and dimensionality reduction tasks. Examples include customer segmentation in marketing and anomaly detection in network security.
Both neural networks and machine learning are instrumental in pushing the boundaries of what AI can achieve. Their applications in scientific research are vast, from analyzing large datasets beyond human capability to making predictive models that can forecast climate changes or disease outbreaks. The continuous improvement of these technologies through advances in computational power and algorithmic efficiency holds the promise of even more significant contributions to science, complementing the work of human scientists rather than replacing them.
Generative AI and Transformers
The landscape of artificial intelligence (AI) has been significantly enriched by the advent of generative AI and transformers, technologies that have redefined the boundaries of machine capability in generating new, original content. Among the most prominent advancements in this area are generative pre-trained transformer (GPT) models, which have showcased remarkable proficiency in processing natural language and generating text that closely mimics human writing styles.
Generative AI: Pioneering Content Creation
Generative AI refers to the subset of AI technologies capable of creating content, ranging from text, images, music, and even video. These systems do not just analyze data but can produce new data instances that retain the statistical properties of their training datasets. The applications of generative AI are vast, from creating realistic computer graphics and composing music to generating written content and developing new drug formulations. The essence of generative AI lies in its ability to learn from existing data and use that knowledge to generate new, unseen instances, opening up possibilities for innovation in content creation and beyond.
Transformers: Revolutionizing Natural Language Processing
Transformers, introduced in the seminal paper “Attention is All You Need” in 2017, represent a breakthrough in how machines handle natural language processing (NLP) tasks. Unlike previous models that processed data sequentially, transformers use a mechanism called ‘attention’ to weigh the relevance of different words within a sentence, allowing for a more nuanced understanding and generation of language. This architecture has proven highly effective for a range of NLP tasks, including translation, summarization, and question-answering.
Generative Pre-trained Transformer (GPT) models, developed by OpenAI, are a prime example of transformers applied to generative AI tasks. GPT models are pre-trained on vast datasets of text and then fine-tuned for specific tasks, enabling them to generate coherent and contextually relevant text based on prompts. The latest iterations, such as GPT-3, have demonstrated an astonishing capacity to produce written content that can often be indistinguishable from that written by humans.
The Role of Generative AI and Transformers in Content Generation
The combination of generative AI and transformer technology has paved the way for AI systems to not only understand and process natural language but also to create it. In the context of scientific research, these technologies can assist in drafting research papers, generating hypotheses, or even creating educational content. Beyond text, generative AI models are being explored for their potential to simulate experimental data, predict chemical reactions, and visualize scientific concepts, thereby enhancing the tools available to scientists for exploration and communication.
Generative AI and transformers represent a significant leap forward in AI’s ability to interact with and generate human-like content, facilitating a deeper integration of AI into creative and scientific processes. While these technologies herald new possibilities for automating content creation, their true potential is realized in augmenting human creativity and scientific inquiry, offering tools that can enhance productivity and foster innovation.
Large Language Models (LLM) and ChatGPT
The advent of large language models (LLMs) such as ChatGPT marks a significant milestone in the journey of artificial intelligence (AI) towards achieving a deep understanding and generation of human-like text. These models, built on the foundation of transformer architectures, have the ability to process vast amounts of information, learn from it, and generate responses that are not only relevant and coherent but often indistinguishable from those a human might produce. Their impact is being felt across a wide range of fields, with scientific research standing out as a domain where their potential is particularly transformative.
Unveiling the Capabilities of LLMs
Large language models like ChatGPT are trained on extensive corpuses of text data, encompassing a wide spectrum of human knowledge and discourse. This training enables them to grasp the nuances of language, context, and even the subtleties of different fields of study. As a result, LLMs can engage in detailed discussions, answer complex questions, and generate content on a myriad of topics with impressive accuracy and depth. Their ability to understand context and generate text based on that understanding has set a new benchmark in natural language processing (NLP), pushing the boundaries of AI’s capability in mimicking human cognitive functions.
Impact on Scientific Research
In the realm of scientific research, LLMs like ChatGPT are revolutionizing the way information is processed, analyzed, and generated. One of the most time-consuming aspects of research is the literature review – a task that LLMs can significantly expedite. By quickly processing and summarizing vast amounts of scientific literature, these models can assist researchers in identifying relevant studies, understanding current trends, and even uncovering gaps in the knowledge base.
Furthermore, LLMs are being utilized in hypothesis generation. Their capacity to analyze existing data and generate insights can lead to the formulation of new research questions and hypotheses. This not only accelerates the research process but also encourages the exploration of novel ideas and perspectives that may not have been considered otherwise.
The potential of LLMs in scientific research extends beyond text-based tasks. Their ability to generate coherent and contextually relevant content can be leveraged to write research proposals, draft scientific papers, and even generate educational materials. By automating aspects of the writing and data analysis process, LLMs can free up researchers to focus more on experimental design, data collection, and the creative aspects of their work.
Facts: How AI Complements Scientists
The narrative around artificial intelligence (AI) often veers towards the sensational, with debates frequently centering on AI’s potential to replace human roles. However, the reality is more collaborative and nuanced, particularly in the realm of scientific research. AI technologies, including machine learning, neural networks, and large language models, have become invaluable tools for scientists, augmenting their research capabilities and enabling discoveries at a pace and scale previously unimaginable. Below, we explore concrete examples of how AI is complementing scientific endeavors, enhancing rather than replacing the role of human scientists.
Drug Discovery
One of the most promising areas where AI complements scientists is in the field of drug discovery. The traditional process of developing new medications is notoriously time-consuming and costly, often taking over a decade and billions of dollars to bring a single drug to market. AI, particularly machine learning models, can dramatically accelerate this process by predicting how different chemical compounds might interact with targets in the human body. For instance, AI algorithms can sift through vast chemical libraries at unprecedented speeds to identify potential drug candidates, a task that would be unfeasible for humans alone.
In recent years, AI-driven platforms have been instrumental in identifying potential treatments for diseases like COVID-19, cancer, and Alzheimer’s disease. By analyzing the structure of disease-causing molecules and simulating millions of potential interactions, AI models have helped narrow down the list of promising compounds for further testing, significantly cutting down the research and development timeline.
Environmental Modeling
AI’s ability to handle and analyze vast datasets is also transforming environmental science. Climate change, with its complex web of causes and effects, presents a daunting challenge for researchers. AI models, particularly those capable of processing and analyzing large-scale environmental data, are proving invaluable in predicting climate patterns, assessing the impact of human activities on ecosystems, and exploring mitigation strategies.
For example, machine learning algorithms are used to improve the accuracy of climate models, predict extreme weather events, and optimize renewable energy production. By analyzing data from satellites, sensors, and historical records, AI can uncover patterns and trends that would be impossible for humans to discern manually, providing insights that inform policy decisions and environmental management strategies.
Handling Vast Amounts of Data
The exponential growth of data across scientific disciplines is another area where AI proves indispensable. From genomics to astrophysics, modern research generates data on a scale that is beyond human capacity to analyze without assistance. AI, with its ability to efficiently process, analyze, and draw insights from large datasets, enables scientists to uncover hidden patterns, correlations, and anomalies that could lead to new discoveries.
In genomics, for example, AI algorithms analyze genetic sequences to identify mutations and variants associated with diseases, accelerating the path towards personalized medicine. Similarly, in astrophysics, AI helps process images and data from telescopes, aiding in the identification of new celestial bodies and phenomena.
Myths: Limitations of AI in Science
While the capabilities of artificial intelligence (AI) in augmenting scientific research are undeniable, several myths and misconceptions cloud the understanding of AI’s role in science. Central to these myths is the overestimation of AI’s autonomy and the underappreciation of the indispensable qualities that human scientists bring to the table. This section aims to demystify some of these misconceptions, emphasizing the limitations of AI and the irreplaceable value of human intuition, creativity, and ethical oversight in scientific discovery.
The Myth of AI Autonomy
One prevalent myth is the belief that AI systems, especially advanced models equipped with machine learning and neural networks, can operate with complete autonomy in conducting scientific research. While AI can process and analyze data at speeds and scales beyond human capability, its function remains largely dependent on human-defined parameters and objectives. AI lacks the intrinsic curiosity and intuition that drive human scientists to ask novel questions, explore uncharted territories, and make intuitive leaps. Human oversight is crucial in guiding AI’s focus, interpreting its findings in a broader context, and making connections that AI on its own might not discern.
Moreover, the development and refinement of AI models require deep domain knowledge and expertise, underscoring the importance of a collaborative relationship between AI and human scientists. AI’s insights and predictions, while valuable, need to be evaluated, validated, and contextualized by human experts who can assess their significance within the larger body of scientific knowledge and societal implications.
The Myth of Replacement
Closely related to the myth of autonomy is the misconception that AI can or will fully replace human scientists. This notion overlooks the complex, multi-faceted nature of scientific inquiry, which encompasses not just the analysis of data but also the formulation of hypotheses, the design of experiments, and the ethical considerations of research. Human scientists possess a unique blend of creativity, ethical judgment, and the ability to synthesize disparate pieces of information into cohesive theories and narratives—capabilities that AI currently cannot replicate.
Furthermore, scientific discovery often involves navigating the unknown and making value judgments about what directions to pursue, areas where human intuition and experience are paramount. AI can provide tools and insights to aid in these endeavors, but it cannot replicate the passion, curiosity, and ethical compass that human scientists bring to their work.
The Essential Role of Human Oversight and Ethical Considerations
Another critical aspect where AI falls short is in ethical considerations and the broader societal impacts of scientific research. The development and application of AI in science must be guided by ethical principles to ensure that research benefits humanity and minimizes harm. Decisions about what projects to pursue, how to interpret findings, and how to apply scientific knowledge in the real world require a moral and ethical framework that AI systems do not possess.
Human scientists play an essential role in ensuring that AI is used responsibly, addressing potential biases in AI algorithms, and considering the long-term implications of their work. The collaboration between AI and human scientists is not just a matter of efficiency or capability but also of ethical responsibility, ensuring that the pursuit of knowledge remains aligned with societal values and the greater good.
The Future of AI and Science
Collaboration Between AI and Scientists
The true potential of artificial intelligence (AI) in science lies not in its capacity to replace human scientists but in its ability to serve as a powerful collaborative tool, enhancing the efficiency, depth, and breadth of scientific research. This synergy between AI and human intelligence is already catalyzing significant breakthroughs and holds the promise for even more revolutionary advancements in the future. By understanding AI as a partner in the quest for knowledge, we can unlock new possibilities and accelerate the pace of discovery.
Enhancing Research Efficiency and Effectiveness
AI’s ability to process and analyze vast datasets rapidly, identify patterns, and generate predictions can significantly reduce the time required for various stages of scientific research. This efficiency boost allows scientists to dedicate more time to the creative and innovative aspects of their work, such as hypothesis formulation, experiment design, and the exploration of new research avenues. For example, in drug discovery, AI algorithms can quickly screen millions of compounds to identify potential candidates for further investigation, a process that would take humans years to complete manually.
Moreover, AI can enhance the effectiveness of research by offering insights and predictions based on data analysis that might be too complex or subtle for human researchers to discern. In climate science, machine learning models are instrumental in predicting climate change impacts with a level of detail and accuracy that was previously unattainable, informing policy decisions and mitigation strategies.
Successful Collaborations and Future Breakthroughs
One emblematic example of successful collaboration between AI and scientists is the use of AI in decoding complex biological processes. AlphaFold, an AI developed by DeepMind, made headlines by solving the protein folding problem, accurately predicting the 3D shapes of proteins based on their amino acid sequences. This breakthrough has profound implications for understanding diseases and developing new treatments, showcasing how AI can tackle challenges that have baffled scientists for decades.
Another area ripe for future breakthroughs through AI-human collaboration is environmental conservation. AI models are being used to monitor wildlife populations, track deforestation, and even predict poaching threats, helping conservationists protect endangered species and habitats more effectively. These applications illustrate how AI can not only process data at an unprecedented scale but also translate it into actionable insights for real-world impact.
The Path Forward
The collaboration between AI and scientists is fostering a new era of research where the analytical and computational prowess of AI complements the creativity, intuition, and ethical judgment of human researchers. Together, they form a potent force for tackling some of the most pressing and complex challenges facing humanity.
As we look to the future, the continued integration of AI into scientific research promises to open up new frontiers of knowledge and innovation. The key to unlocking this potential lies in fostering a collaborative mindset, where AI is seen as an essential partner in the scientific endeavor, amplifying our capabilities and accelerating our quest for understanding and discovery.
This partnership between AI and human intelligence heralds a future where the boundaries of what is possible are continually expanded, driven by the synergistic power of collaboration. As AI technologies evolve and mature, their role in augmenting the capabilities of scientists will undoubtedly grow, leading to breakthroughs that we can scarcely imagine today. The journey ahead is one of shared endeavor, where AI and scientists work together to unveil the mysteries of the universe and harness knowledge for the benefit of all.
Ethical and Societal Implications
As artificial intelligence (AI) becomes increasingly integrated into scientific research, it brings to the forefront critical ethical and societal implications that must be navigated carefully. The alignment of AI development with ethical boundaries ensures that these powerful tools serve the greater good while minimizing potential harms. This section delves into the paramount importance of ethical alignment in AI development and the principles guiding the responsible use of AI in science, with a particular focus on data privacy and security.
Ethical Alignment in AI Development
The development of AI technologies, especially those deployed in scientific research, must be underpinned by a strong ethical framework. This framework should ensure that AI systems are designed and operated in ways that respect human rights, dignity, and diversity. Ethical alignment involves several key aspects, including fairness, transparency, accountability, and inclusivity. AI systems must be free from biases that could lead to discriminatory outcomes, and their decision-making processes should be transparent and understandable to those impacted by their applications. Moreover, there should be mechanisms for accountability, where errors or unintended consequences can be addressed and rectified.
The alignment of AI with ethical standards is not just a technical challenge but also a moral imperative. It requires ongoing collaboration between AI developers, ethicists, policymakers, and the broader scientific community to continually assess and refine these technologies according to ethical principles and societal values.
Responsible Use of AI in Science
The responsible use of AI in scientific research extends beyond ethical alignment to include the careful consideration of data privacy and security. Scientific research often involves sensitive data that must be handled with the utmost care to protect individuals’ privacy and maintain public trust.
Data privacy in the context of AI-driven research entails ensuring that personal information used by AI systems is anonymized and securely stored, minimizing the risk of breaches that could expose sensitive information. Additionally, there should be clear consent protocols for the use of personal data in research, ensuring that individuals are informed about how their data will be used and have control over their participation.
Security measures are equally crucial, as AI systems processing vast amounts of scientific data become attractive targets for cyberattacks. Implementing robust security protocols to protect data integrity and prevent unauthorized access is essential for maintaining the credibility and reliability of scientific research.
Looking Forward
The ethical and societal implications of integrating AI into scientific research underscore the need for a proactive and reflective approach to AI development and deployment. By prioritizing ethical alignment and the responsible use of AI, the scientific community can harness the benefits of these technologies while navigating potential pitfalls. This approach ensures that AI-driven advancements in science are achieved responsibly, with a commitment to upholding ethical standards, protecting data privacy, and securing the trust and well-being of society at large.
As we continue to explore the vast potential of AI in scientific discovery, the principles of ethical alignment and responsible use serve as guiding lights, ensuring that our journey is marked by innovation that is both groundbreaking and grounded in the highest ethical standards.
Preparing for a Multi-Modal Future
The future of artificial intelligence (AI) in scientific research is increasingly multi-modal, characterized by systems capable of analyzing and interpreting various types of data. This evolution towards multi-modal AI promises to further enhance the depth and breadth of scientific inquiries, enabling researchers to gain more comprehensive insights and make novel discoveries that were previously unattainable.
Understanding Multi-Modal AI Systems
Multi-modal AI systems are designed to process and integrate different forms of data, such as text, images, audio, and video, simultaneously. Unlike traditional AI models that typically specialize in one type of data, multi-modal systems can draw on a diverse array of data sources to make more informed analyses and predictions. This capability is particularly valuable in scientific research, where complex phenomena often need to be understood through multiple data lenses.
For instance, in environmental science, a multi-modal AI system could analyze satellite imagery, temperature data, textual reports, and social media posts to assess the impact of climate change on a specific ecosystem. By synthesizing these varied data types, the system can provide a more holistic view of environmental changes and their socio-economic ramifications.
Advancing Scientific Research with Multi-Modal AI
The integration of multi-modal AI in scientific research opens up new avenues for exploration and discovery. By leveraging the strengths of different data modalities, researchers can formulate more nuanced hypotheses and conduct more comprehensive studies. For example, in health sciences, multi-modal AI can improve disease diagnosis and treatment by analyzing a patient’s genetic data, medical imaging, electronic health records, and even lifestyle information from wearable devices. This holistic approach can lead to personalized medicine strategies that consider the full spectrum of factors influencing an individual’s health.
Furthermore, multi-modal AI systems can uncover correlations and patterns across different data types that might be invisible when examining data in isolation. In fields like astrophysics or quantum physics, where experiments can generate an overwhelming amount of data in various formats, multi-modal AI can help scientists navigate this complexity, identifying critical insights among the noise.
Embracing the Multi-Modal Future
To prepare for a future dominated by multi-modal AI, the scientific community must invest in the development of advanced AI models capable of handling the complexity and diversity of multi-modal data. This includes not only technical advancements but also the creation of robust datasets that encompass multiple data types and the development of new methodologies for integrating and interpreting this information.
Moreover, ethical considerations remain paramount, as the use of multi-modal data raises complex issues around data privacy, consent, and security. Ensuring that multi-modal AI systems are developed and used in a manner that respects individual rights and societal values is essential for their successful integration into scientific research.
The shift towards multi-modal AI represents a significant leap forward in our ability to understand and address the complex challenges facing the world today. By harnessing the power of diverse data types, scientists equipped with multi-modal AI tools can drive forward the frontiers of knowledge, making discoveries that enrich our understanding of the universe and improve the quality of human life. As we stand on the brink of this multi-modal future, the potential for transformative scientific breakthroughs has never been more exciting.
Conclusion to AI Replacing Scientists
The exploration of artificial intelligence (AI) in scientific research has revealed a landscape rich with potential, marked by both extraordinary capabilities and important limitations. AI, with its diverse applications from neural networks and machine learning to generative AI, transformers, and multi-modal systems, stands as a testament to human ingenuity and the relentless pursuit of knowledge. However, the journey through this landscape underscores a fundamental truth: AI is a tool for augmentation, not a replacement for the irreplaceable depth, creativity, and ethical judgment of human scientists.
Embracing AI as a Collaborative Partner
The key to unlocking the full potential of AI in science lies in recognizing and embracing its role as a collaborative partner. AI’s ability to process vast amounts of data, identify patterns, and generate predictions can significantly enhance the efficiency and scope of scientific research. From accelerating drug discovery and environmental modeling to providing comprehensive insights across various fields, AI systems augment human capabilities, allowing scientists to tackle more significant challenges and explore new frontiers of understanding.
Navigating Ethical and Societal Implications
As we integrate AI more deeply into the fabric of scientific inquiry, navigating its ethical and societal implications with care and foresight becomes paramount. Ensuring that AI systems operate within ethical boundaries, respecting data privacy, and securing against potential biases, underscores the need for a collaborative approach that includes ethicists, policymakers, and the broader community alongside scientists and AI developers.
Looking to the Future
The future of scientific research, enriched by multi-modal AI, promises a multi-dimensional approach to understanding and solving the complex problems facing humanity. As we prepare for this future, the emphasis on AI as a tool for augmentation rather than replacement remains critical. By fostering a symbiotic relationship between AI and human scientists, we can leverage the strengths of both to achieve a greater collective impact.
The importance of embracing AI in science cannot be overstated. It represents a powerful ally in our ongoing quest for knowledge, offering the promise of faster breakthroughs, deeper insights, and the exploration of previously unimaginable realms of discovery. However, the heart of scientific inquiry—characterized by curiosity, creativity, and ethical consideration—remains distinctly human.
In conclusion to AI replacing scientists, as we stand at the intersection of human intelligence and artificial intelligence, the path forward is one of collaboration and mutual enhancement. By embracing AI as an augmentative tool, the scientific community can navigate the complexities of the natural world with greater agility and precision, heralding a new era of discovery that respects both the potential of technology and the irreplaceable value of human insight.
FREQUENTLY ASKED QUESTIONS
These FAQs underscore the balanced view of AI’s role in science, highlighting its potential to augment human capabilities while dispelling myths about its limitations and the fear of replacement. Embracing AI as a collaborative partner in scientific research holds the promise of a brighter, more informed future.