Cloud-Native AI Platforms for Data Scientists
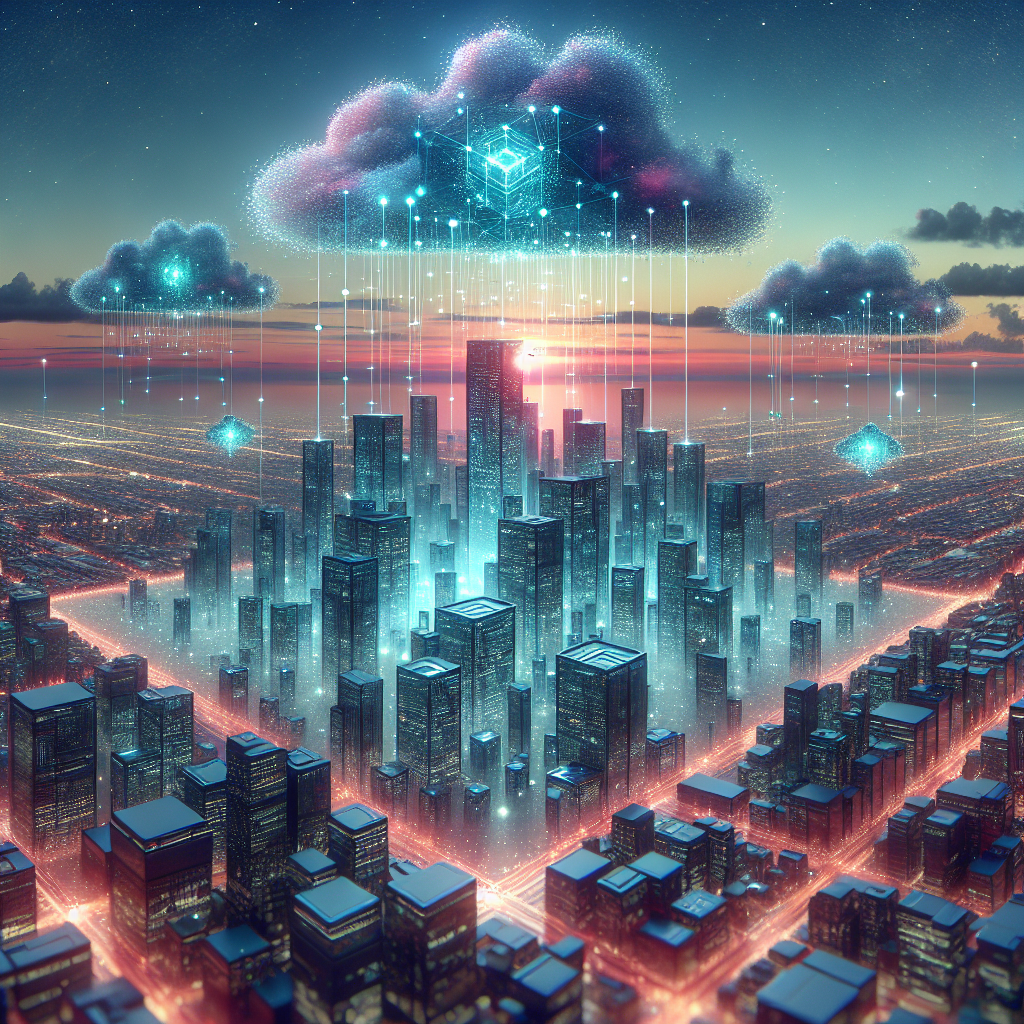
Cloud-Native AI Platforms for Data Scientists: Revolutionizing the Future of Data Analysis
The integration of Cloud Native technologies with Artificial Intelligence (AI) has given rise to a new generation of platforms that are specifically designed for Data Scientists. These Cloud-Native AI Platforms offer a wide range of benefits, including scalability, flexibility, and cost-effectiveness, making them an attractive option for organizations looking to leverage the power of AI in their data analysis. By exploring cloud-native AI platforms for data scientists, organizations can gain a deeper understanding of the advantages of cloud-native AI platforms and how they can be used to drive business success.
Introduction to Cloud-Native AI Platforms
Cloud-Native AI platforms are designed to take advantage of the scalability and flexibility of cloud computing, allowing Data Scientists to build, deploy, and manage AI models more efficiently. These platforms provide a suite of tools and services that enable Data Scientists to focus on building and training AI models, rather than worrying about the underlying infrastructure. With Cloud-Native AI Platforms, Data Scientists can quickly spin up and down resources as needed, reducing costs and increasing productivity. For example, Azure’s cloud-based platform provides a scalable and secure environment for Data Scientists to build and deploy AI models.
Benefits of Cloud-Native AI Platforms for Data Scientists
The benefits of using Cloud-Native AI platforms for Data Scientists are numerous. Some of the key advantages include:
- Scalability: Cloud-Native AI platforms allow Data Scientists to scale their resources up or down as needed, making it easier to handle large datasets and complex models.
- Flexibility: These platforms provide a wide range of tools and services, allowing Data Scientists to choose the best approach for their specific use case.
- Cost-effectiveness: By only paying for the resources they use, organizations can reduce costs and increase ROI on their AI investments.
- Collaboration: Cloud-Native AI platforms make it easier for Data Scientists to collaborate with each other, regardless of location or organization. Understanding the advantages of cloud-native AI platforms is crucial for organizations looking to unlock the full potential of AI.
Key Features of Cloud-Native AI Platforms
Some of the key features of Cloud-Native AI platforms include:
- Automated Machine Learning: Automated machine learning (AutoML) capabilities that enable Data Scientists to build and train models quickly and efficiently.
- Data Preparation: Tools and services for data preparation, including data ingestion, processing, and visualization.
- Model Deployment: Capabilities for deploying AI models in a variety of environments, including cloud, on-premises, and edge devices.
- Monitoring and Maintenance: Features that allow Data Scientists to monitor and maintain their AI models in real-time, ensuring optimal performance and accuracy.
Real-World Applications of Cloud-Native AI Platforms
Cloud-Native AI platforms have a wide range of applications across various industries, including:
- Healthcare: Cloud-Native AI platforms can be used to develop personalized medicine, predict patient outcomes, and improve disease diagnosis.
- Finance: These platforms can be used to detect fraudulent transactions, predict stock prices, and optimize investment portfolios.
- Retail: Cloud-Native AI platforms can be used to personalize customer experiences, predict demand, and optimize supply chain operations.
Use Cases for Cloud-Native AI Platforms
Some use cases for Cloud-Native AI platforms include:
- Predictive Maintenance: Using machine learning algorithms to predict when equipment is likely to fail, allowing for proactive maintenance and reducing downtime.
- Customer Segmentation: Using clustering algorithms to segment customers based on their behavior and preferences, allowing for targeted marketing and improved customer experiences.
- Image Classification: Using deep learning algorithms to classify images into different categories, such as objects, scenes, or activities.
Best Practices for Implementing Cloud-Native AI Platforms
To get the most out of Cloud-Native AI platforms, organizations should follow best practices such as:
- Start Small: Begin with a small pilot project to test and refine your approach before scaling up.
- Choose the Right Tools: Select tools and services that align with your organization’s goals and requirements.
- Develop a Strong Data Strategy: Ensure that you have a robust data strategy in place, including data ingestion, processing, and storage.
Future of Cloud-Native AI Platforms
The future of Cloud-Native AI platforms is promising, with advancements in technologies such as edge computing, 5G networks, and quantum computing. As these technologies continue to evolve, we can expect to see even more innovative applications of Cloud-Native AI platforms across various industries. Some potential areas of growth include:
- Edge AI: Using Cloud-Native AI platforms to develop AI models that can run on edge devices, reducing latency and improving real-time decision-making.
- Explainable AI: Developing AI models that can provide transparent and interpretable results, helping to build trust and confidence in AI decision-making.
Getting Started with Cloud-Native AI Platforms
To get started with Cloud-Native AI platforms, organizations should:
- Assess their current infrastructure: Evaluate their existing infrastructure and identify areas where Cloud-Native AI platforms can be integrated.
- Develop a cloud strategy: Create a comprehensive cloud strategy that aligns with their business goals and objectives.
- Choose the right platform: Select a Cloud-Native AI platform that meets their specific needs and requirements.
By following these steps and understanding the advantages of cloud-native AI platforms, organizations can unlock the full potential of AI and drive business success in today’s fast-paced digital landscape.
Conclusion
In conclusion, Cloud-Native AI platforms offer a wide range of benefits for Data Scientists, including scalability, flexibility, cost-effectiveness, and collaboration. By exploring cloud-native AI platforms for data scientists and understanding the advantages of cloud-native AI platforms, organizations can gain a deeper understanding of how to use these platforms to drive business success. With the right strategy and support, Cloud-Native AI platforms can help organizations to unlock the full potential of AI and achieve their goals.
By following these strategies and understanding the advantages of cloud-native AI platforms, organizations can unlock the full potential of AI and drive business success. Whether you’re just starting out with Cloud-Native AI platforms or looking to expand your existing capabilities, there’s never been a better time to explore the possibilities of AI and cloud computing. With the right approach and support, you can achieve your goals and stay ahead of the competition in today’s fast-paced digital landscape.
Frequently Asked Questions
1. What are Cloud-Native AI Platforms?
Cloud-Native AI platforms are designed to take advantage of the scalability and flexibility of cloud computing, allowing Data Scientists to build, deploy, and manage AI models more efficiently.
2. What are the benefits of using Cloud-Native AI Platforms for Data Scientists?
The benefits of using Cloud-Native AI platforms for Data Scientists include scalability, flexibility, cost-effectiveness, and collaboration.
3. What are some key features of Cloud-Native AI Platforms?
Some key features of Cloud-Native AI platforms include automated machine learning, data preparation, model deployment, and monitoring and maintenance.
4. What are some use cases for Cloud-Native AI Platforms?
Some use cases for Cloud-Native AI platforms include predictive maintenance, customer segmentation, and image classification.
5. How can organizations overcome the challenges and limitations of Cloud-Native AI Platforms?
To overcome the challenges and limitations of Cloud-Native AI platforms, organizations can develop a cloud strategy, invest in security and compliance measures, provide training and support for Data Scientists, and partner with cloud providers to access expertise and resources.