Data Science in Sports Analytics
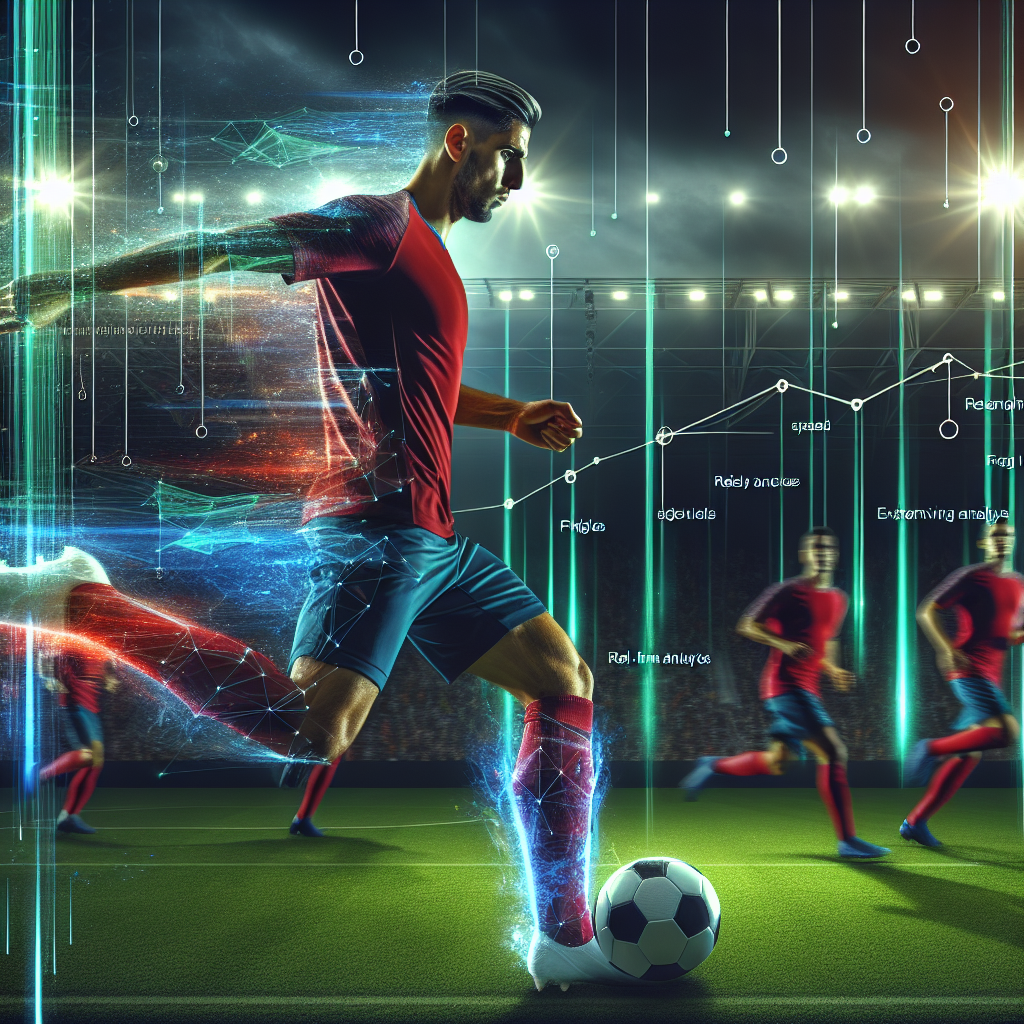
Data Science in Sports Analytics: Enhancing Performance and Strategy
In today’s fast-paced world of professional sports, data science is becoming an indispensable tool that helps teams gain a competitive edge. By leveraging vast amounts of data through sports analytics, organizations can make informed decisions to optimize player performance, develop strategic game plans, and improve overall team dynamics. Whether it’s predicting injuries or personalizing fan experiences, data-driven insights are transforming the way sports are played, managed, and analyzed.
The Role of Data Science in Sports Analytics
1. Player Performance Analysis
One of the most significant applications of data science in sports is player performance analysis. By collecting detailed metrics such as speed, endurance, and accuracy, teams can identify areas for improvement and tailor training programs to enhance individual skills. For example, ESPN uses advanced analytics to track player statistics and provide insights that help coaches make informed decisions.
Detailed Metrics Collection
Detailed metrics collection involves using wearable technology and sensors to gather data on players’ physical performance during games and practices. This includes GPS tracking devices that monitor speed and distance covered, heart rate monitors that gauge endurance, and motion capture systems that assess technique and accuracy. The resulting data can be analyzed in real-time or retrospectively to provide actionable insights.
Tailored Training Programs
Tailored training programs are designed based on the specific needs of each player as identified through performance analysis. For instance, if a running back shows signs of fatigue during key plays, coaches might incorporate additional conditioning exercises focused on improving endurance. Conversely, if an outfielder struggles with ball tracking, they could receive specialized drills to enhance their accuracy.
Example: The Chicago Bulls and SAP SportsAnalytics
The Chicago Bulls have been at the forefront of integrating data science into player performance analysis. They use SAP SportsAnalytics to collect and analyze large datasets from games, practices, and training sessions. This information helps coaches identify strengths and weaknesses, optimize play strategies, and improve overall team performance.
2. Injury Prevention
Injuries are a significant risk in sports, often leading to extended downtime and potential career-ending setbacks. Data science can play a crucial role in injury prevention by identifying patterns that may indicate an increased risk of harm. Advanced analytics can monitor player activity levels during training sessions to predict when players might be at risk for overuse injuries. Teams can then adjust their practice schedules accordingly, reducing the likelihood of these incidents.
Activity Level Monitoring
Activity level monitoring involves tracking the physical exertion and stress placed on players during practices and games. This data can include metrics such as distance covered, acceleration/deceleration rates, heart rate variability, and time spent in high-intensity activities. By analyzing these factors over time, teams can identify patterns that may indicate an increased risk of injury.
Predictive Analytics for Injury Risk
Predictive analytics for injury risk uses machine learning algorithms to analyze historical data on player performance and injury history. These models can identify specific biomarkers or activity levels that are associated with a higher likelihood of injury. Teams can then use this information to adjust practice schedules, workload distribution, and training intensity to minimize the risk of injuries.
Example: The NFL and Injury Prediction Models
The National Football League (NFL) has implemented predictive analytics for injury prevention in collaboration with various data science firms. These models analyze player performance data and other factors such as medical history to predict which players are at highest risk of injury during the season. Teams can use this information to make strategic decisions about player workload, rest periods, and recovery protocols.
3. Strategic Decision-Making
Data-driven insights are invaluable in developing strategic game plans and making key decisions during matches. Predictive modeling for game outcomes uses historical data on team performance, player statistics, and external factors such as weather conditions to forecast the likelihood of various outcomes. This information can help coaches make more informed decisions about tactics, substitutions, and resource allocation.
Predictive Modeling for Game Outcomes
Predictive modeling for game outcomes involves using statistical techniques and machine learning algorithms to analyze historical data on team performance and player statistics. These models can account for a wide range of variables such as offensive/defensive strengths, recent form, home field advantage, and even external factors like weather conditions.
Key Decision Support
Key decision support systems provide coaches with real-time insights during matches to help them make critical decisions. For example, if predictive models indicate that the opposing team is more likely to score in the next 15 minutes, a coach might choose to substitute key players or adjust defensive strategies accordingly. Similarly, if models suggest that a particular play has a high success rate, coaches could consider incorporating it into their game plan.
Example: Stats Perform and Predictive Analytics
Stats Perform is a leading provider of data analytics services for the sports industry. They use advanced statistical models and machine learning algorithms to provide predictive analytics for game outcomes across various sports. Their insights help teams make strategic decisions about tactics, player rotations, and injury management.
4. Fan Engagement and Experience
Data science also plays a crucial role in enhancing fan engagement and experience. Teams can leverage data from social media interactions, ticket sales, and merchandise purchases to create personalized experiences for fans. Personalized Content Delivery involves using machine learning algorithms to analyze individual preferences and deliver relevant content such as game highlights, player interviews, or behind-the-scenes footage.
Personalized Content Delivery
Personalized content delivery uses machine learning algorithms to analyze fan behavior and preferences. This includes metrics such as social media engagement, ticket purchase history, merchandise purchases, and app usage data. By analyzing these factors, teams can create personalized content recommendations that resonate with individual fans.
Enhanced Fan Experience
Enhanced fan experience involves using data-driven insights to improve various aspects of the fan experience. For example, teams can use predictive analytics to optimize seating arrangements based on fan preferences, provide real-time game updates through mobile apps, and offer exclusive behind-the-scenes content to season ticket holders.
Example: The Atlanta Braves and SAP SportsAnalytics
The Atlanta Braves have been successful in leveraging data science to enhance fan engagement. They use SAP SportsAnalytics to analyze data from social media interactions, ticket sales, and merchandise purchases. This information helps them create personalized experiences for fans, such as targeted promotions, content recommendations, and interactive game-day features.
The Future of Data Science in Sports Analytics
As technology continues to advance, the role of data science in sports analytics will only grow. Teams that embrace these tools will have a significant competitive advantage, while those that lag behind risk falling short. By leveraging big data, predictive modeling, and advanced analytics, organizations can optimize performance, enhance fan engagement, and drive financial success.
Advancements in Technology
Advancements in technology are driving the evolution of data science in sports analytics. New sensors and wearable devices are providing more granular and real-time data on player performance and health. Machine learning algorithms are becoming more sophisticated, enabling teams to extract deeper insights from large datasets. Cloud computing is making it easier for teams to store, process, and analyze vast amounts of data.
Ethical Considerations
Ethical considerations are an important aspect of the future of data science in sports analytics. Teams must ensure that they handle player data responsibly and transparently, respecting privacy rights and complying with relevant regulations. They should also consider the potential biases that can arise from data-driven decision-making processes and take steps to mitigate these risks.
Collaboration and Innovation
Collaboration and innovation are key drivers of progress in the field of sports analytics. Teams can benefit from partnering with data science firms, universities, and research institutions to stay up-to-date on the latest advancements and best practices. By fostering a culture of collaboration and continuous improvement, organizations can unlock new opportunities for success.
Conclusion
In conclusion, data science is reshaping the world of professional sports. From player performance analysis to strategic decision-making, these tools are providing valuable insights that help teams gain a competitive edge. As we continue to explore new applications of data-driven technologies, the future of sports analytics looks brighter than ever before.
So whether you’re a coach looking to optimize team strategies or a fan eager for personalized experiences, data science is transforming the way we engage with and enjoy professional sports. Embrace these advancements, and you’ll be at the forefront of this exciting evolution.