Deep Learning Innovations in the AI Landscape
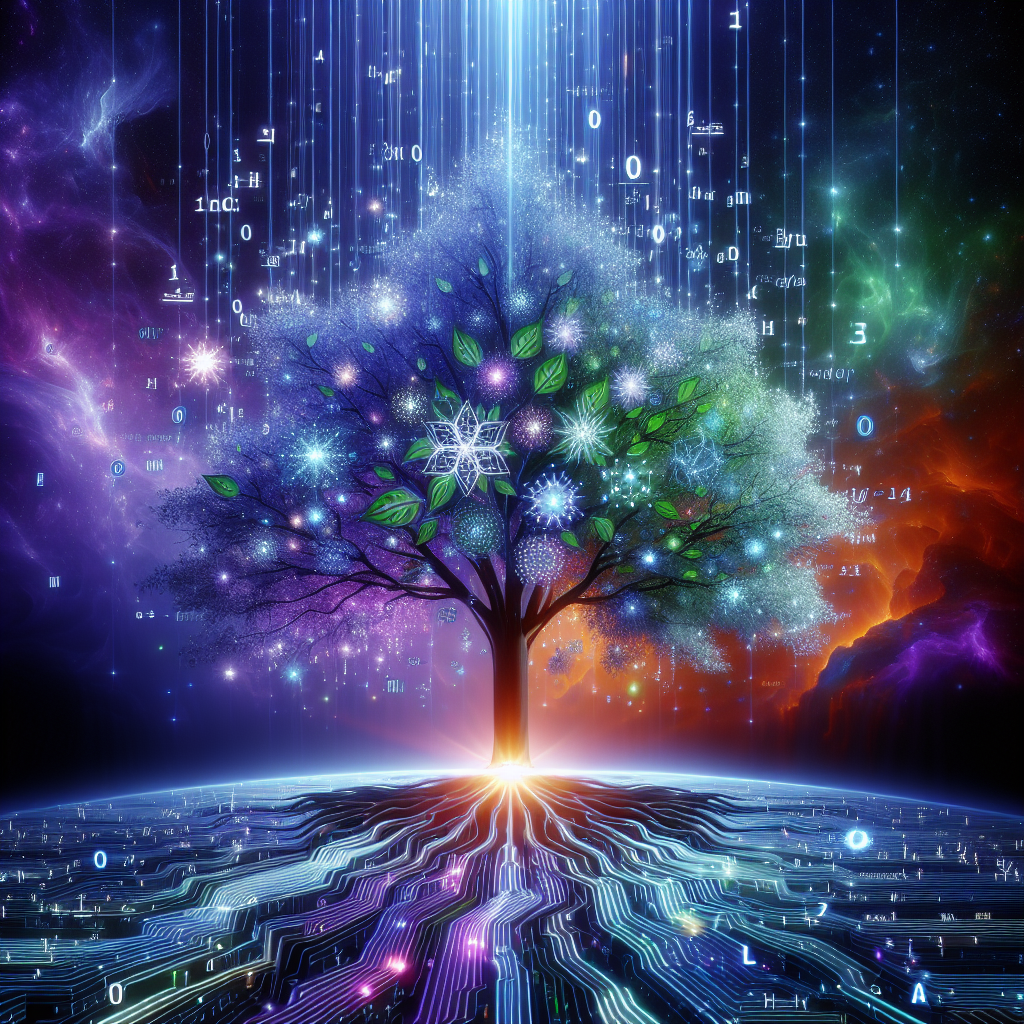
The integration of deep learning techniques has significantly enhanced AI capabilities. As a cornerstone of modern artificial intelligence, deep learning continues to evolve rapidly, driving technological advancements and opening new avenues for innovation across various sectors. This blog post explores cutting-edge innovations in deep learning, focusing on applications, research developments, and influential figures like Geoffrey Hinton.
Introduction
Artificial Intelligence (AI) has witnessed an unparalleled surge in growth over recent years, largely fueled by advances in deep learning. Deep learning, a subset of machine learning involving neural networks with many layers, enables machines to learn from data in complex ways. This technology has revolutionized fields ranging from healthcare and finance to autonomous vehicles and natural language processing.
In this exploration, we delve into the transformative impact of deep learning applications, recent AI technological advancements, and innovative neural network models being developed by leading entities such as OpenAI and pioneers like Geoffrey Hinton. Silicon Valley remains a hotbed for these innovations, fostering an ecosystem where groundbreaking ideas thrive.
AI Landscape with Deep Learning Innovations
The Rise of Deep Learning Applications
Deep learning has broadened the horizons of AI applications, offering unprecedented capabilities:
- Computer Vision: Neural networks excel at tasks such as image recognition and object detection. Innovations in this field enable technologies like facial recognition systems and autonomous vehicles to improve accuracy and efficiency.
- Natural Language Processing (NLP): Deep learning models, particularly transformers, have dramatically enhanced language understanding. Applications include real-time translation services, sentiment analysis tools, and AI-driven chatbots that provide customer support.
- Healthcare: In the medical field, deep learning aids in diagnostics by analyzing complex data from MRIs, CT scans, and genetic information, offering faster and more accurate diagnoses than traditional methods.
Expanded Insights:
Deep learning applications are expanding into new domains such as agriculture, where they optimize crop yield predictions and pest detection. In retail, these models enhance customer experiences through personalized recommendations and inventory management systems. Moreover, deep reinforcement learning is being applied to energy management systems, optimizing grid operations and reducing carbon footprints.
Recent AI Technological Advancements
The landscape of AI is continually evolving with novel technological advancements:
- Transformers and BERT Models: Introduced by Google and further developed by entities like OpenAI, transformer architectures have revolutionized NLP tasks by enabling models to understand context better than ever before.
- Generative Adversarial Networks (GANs): These networks create realistic data outputs, useful in generating art, enhancing photo realism, and even simulating medical scenarios for training purposes.
- Reinforcement Learning: This approach has seen applications in gaming and robotics, where AI agents learn optimal strategies through trial and error, mimicking human learning processes.
Expanded Insights:
Recent advancements also include the development of self-supervised learning techniques that reduce dependency on labeled data, making machine learning models more efficient and scalable. Additionally, federated learning is emerging as a crucial technology for training models across decentralized devices while maintaining privacy.
Innovative Neural Network Models
Researchers are exploring new neural architectures to improve machine learning outcomes:
- Capsule Networks: These models promise better spatial data understanding, potentially outperforming traditional convolutional networks in certain tasks.
- Neural Architecture Search (NAS): Automated techniques for optimizing model design efficiently, reducing the need for manual intervention.
Expanded Insights:
Emerging neural network innovations include attention mechanisms that improve focus on relevant parts of input data, boosting performance in tasks like image captioning. Also noteworthy is the exploration of spiking neural networks, which mimic biological processes to enhance energy efficiency and processing speed.
The Role of Key Players
OpenAI and Silicon Valley’s Influence
OpenAI continues to lead with groundbreaking research and open-sourced projects that empower developers worldwide. Silicon Valley serves as a fertile ground for these innovations, hosting numerous startups and established companies dedicated to advancing AI technology.
Expanded Insights:
Other key players in the deep learning space include DeepMind, known for its success in game-playing AI like AlphaGo, and Facebook’s AI Research (FAIR), which contributes significantly to NLP advancements. Collaborations between academia and industry are also pivotal, with institutions such as Stanford University driving forward theoretical understanding while practical applications are honed by tech giants.
Geoffrey Hinton: A Pioneer in Deep Learning
Geoffrey Hinton, often referred to as the “godfather of deep learning,” has been instrumental in developing key algorithms that underpin many modern neural network models. His contributions continue to inspire a new generation of researchers exploring innovative applications and advancements.
Expanded Insights:
Hinton’s work on backpropagation laid the groundwork for training multi-layered neural networks, an essential component in today’s AI systems. His recent endeavors focus on enhancing deep learning interpretability, aiming to make models more transparent and trustworthy.
Future Directions and Challenges
As we look ahead, deep learning is poised to tackle even more complex challenges. Integrating quantum computing with deep learning could revolutionize computational capabilities, addressing limitations faced by classical systems. Moreover, ethical considerations are gaining prominence, with researchers striving to mitigate biases in AI models and ensure equitable access to technology.
Expanded Insights:
The scalability of deep learning models presents both opportunities and challenges. While larger datasets can improve model accuracy, they also demand more computational resources. Innovations like edge computing are helping address these issues by processing data closer to the source, reducing latency and bandwidth usage.
Conclusion
Deep learning continues to push the boundaries of what AI can achieve, transforming industries and impacting our daily lives in profound ways. The ongoing collaboration between researchers, industry leaders, and academia is crucial for overcoming existing challenges and unlocking new possibilities. As we embrace these innovations, it’s essential to ensure that they are developed responsibly and equitably, benefiting society as a whole.
Looking Ahead
The future of deep learning is bright, with endless potential for growth and innovation. By continuing to explore new architectures, techniques, and applications, the field will undoubtedly lead us into uncharted territories, shaping the technological landscape of tomorrow.