Enhancing Agent Reasoning Techniques
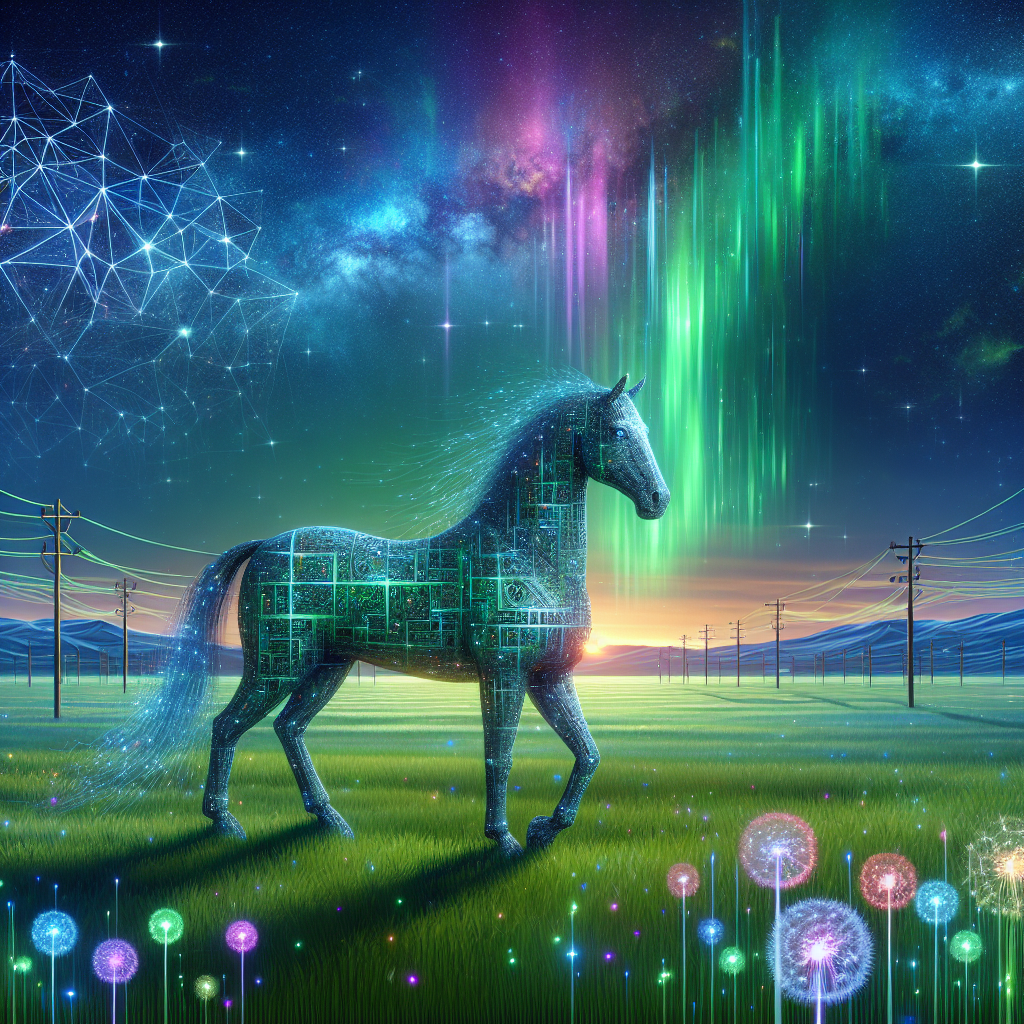
In a rapidly evolving technological landscape, enhancing agent reasoning techniques is pivotal for innovation across industries. As artificial intelligence (AI) becomes increasingly sophisticated, understanding and implementing advanced reasoning algorithms is crucial for decision-makers aiming to fully harness the potential of cognitive computing strategies. This article explores the intricacies of AI agent decision-making, offering actionable insights through a data-driven approach and engaging storytelling to demystify complex topics.
Introduction
In today’s competitive environment, businesses are turning to artificial intelligence (AI) as a catalyst for innovation and efficiency. Recent studies indicate that organizations integrating AI technologies can boost productivity by up to 40%. Central to achieving such gains is the enhancement of agent reasoning techniques—enabling AI agents to replicate human decision-making processes more effectively.
This article delves into how machine learning integration with cognitive computing strategies can revolutionize AI agent decision-making, drawing insights from leading institutions like OpenAI, Stanford University, and MIT Media Lab. By the end of this piece, you will gain a comprehensive understanding of advanced reasoning algorithms and actionable steps to refine your AI models.
The Evolution of AI Agent Decision-Making
From Simple Algorithms to Advanced Reasoning Systems
Initially, AI agents operated on simple, rule-based algorithms that lacked flexibility. Today, they have evolved into sophisticated systems capable of mimicking human thought processes through advanced reasoning algorithms.
Key Drivers of Evolution:
- Data Quality and Volume: Access to vast amounts of high-quality data has enabled more accurate predictions and decisions.
- Computational Power: Enhanced processing capabilities allow AI agents to analyze complex datasets swiftly, leading to more nuanced decision-making.
- Algorithmic Advancements: Innovations in machine learning techniques have significantly improved the ability of AI systems to learn from data autonomously.
Historical Context
AI’s journey began with basic automation tasks and has since advanced into realms requiring sophisticated reasoning. Early systems relied heavily on predefined rules, but these were limited by their lack of adaptability. With breakthroughs in deep learning—a subset of machine learning—AI agents now possess the capacity to learn patterns from data without explicit programming, revolutionizing decision-making processes.
Improving Artificial Intelligence Models
To better mimic human decision processes, it is essential to focus on improving artificial intelligence models. This involves integrating diverse data sources and refining algorithms to increase accuracy and reliability.
Integrating Machine Learning Techniques for Problem-Solving
Integrating machine learning techniques into AI agents allows them to solve problems more effectively by analyzing patterns and making informed predictions. By employing supervised, unsupervised, and reinforcement learning, AI models can adapt to new information and continuously improve their decision-making capabilities.
Types of Machine Learning Techniques:
- Supervised Learning: Utilizes labeled data to train algorithms that predict outcomes based on historical examples.
- Unsupervised Learning: Involves identifying patterns in unlabeled data, enabling the discovery of hidden structures or features.
- Reinforcement Learning: Employs trial-and-error learning where agents learn optimal actions through rewards and penalties.
Case Study: Predictive Maintenance
A real-world application is predictive maintenance in manufacturing. Companies utilize AI models to predict equipment failures before they occur, reducing downtime and maintenance costs. By integrating machine learning algorithms, these systems analyze data from sensors and historical records to forecast potential breakdowns, demonstrating the transformative power of advanced reasoning algorithms.
Cognitive Computing Strategies
Cognitive computing represents a paradigm shift, empowering machines to simulate human thought processes in complex scenarios.
Core Elements:
- Natural Language Processing (NLP): Enables AI agents to understand and generate human language, facilitating more intuitive interactions.
- Knowledge Representation: Involves structuring information so that it is accessible for machine interpretation, enhancing decision-making accuracy.
- Machine Perception: Allows systems to interpret sensory data—such as images or sounds—to make informed decisions.
Example: Customer Service Automation
AI-powered chatbots equipped with NLP capabilities can handle customer inquiries efficiently. By understanding and processing language nuances, these agents provide accurate responses and escalate issues when necessary, exemplifying cognitive computing strategies in action.
Industry Trends and Future Predictions
As AI continues to evolve, several trends are shaping the future of agent reasoning:
- Increased Use of Explainable AI (XAI): There is a growing demand for transparency in AI decision-making processes. Companies are investing in XAI to make AI systems more understandable and trustworthy.
- Ethical Considerations: As AI becomes integral to business operations, ethical guidelines surrounding data privacy and bias mitigation are gaining prominence.
- Integration with IoT: The convergence of AI and the Internet of Things (IoT) is paving the way for smarter environments where devices communicate seamlessly to optimize processes.
Future Outlook
Experts predict that by 2030, AI will be capable of autonomous reasoning in complex scenarios, further blurring the lines between human and machine capabilities. This evolution promises unprecedented opportunities across sectors, from healthcare diagnostics to financial analytics.
Actionable Insights for Decision-Makers
To effectively leverage AI agent reasoning techniques, decision-makers should consider the following strategies:
- Invest in Data Infrastructure: Ensure access to high-quality data as it is foundational for developing robust AI models.
- Foster Interdisciplinary Collaboration: Encourage collaboration between data scientists, domain experts, and IT professionals to drive innovation.
- Prioritize Ethical AI Practices: Implement guidelines to address ethical concerns such as bias and privacy in AI systems.
Practical Advice
- Conduct Pilot Projects: Start with small-scale implementations to test AI solutions before full deployment.
- Stay Informed on Technological Advancements: Continuously update your knowledge of the latest AI developments to maintain a competitive edge.
Ready to Transform Your Business with AI?
Unlock the full potential of your operations by integrating cutting-edge AI agent reasoning techniques. Our AI Agentic software development and AI Cloud Agents services are tailored to enhance decision-making processes, driving efficiency and innovation across industries. We’ve collaborated with companies in healthcare, finance, and customer service to implement solutions that significantly improve outcomes.
By partnering with us, you’ll gain access to expert guidance on the latest advancements from institutions like OpenAI, Stanford University, and MIT Media Lab. Whether refining your AI models or exploring new machine learning applications, we’re here to help. Contact us today to learn how our solutions can transform your business.
For inquiries and further information, visit our website or reach out through our contact page. We look forward to helping you achieve your AI goals!