Exploring Agent Planning Systems Effectively
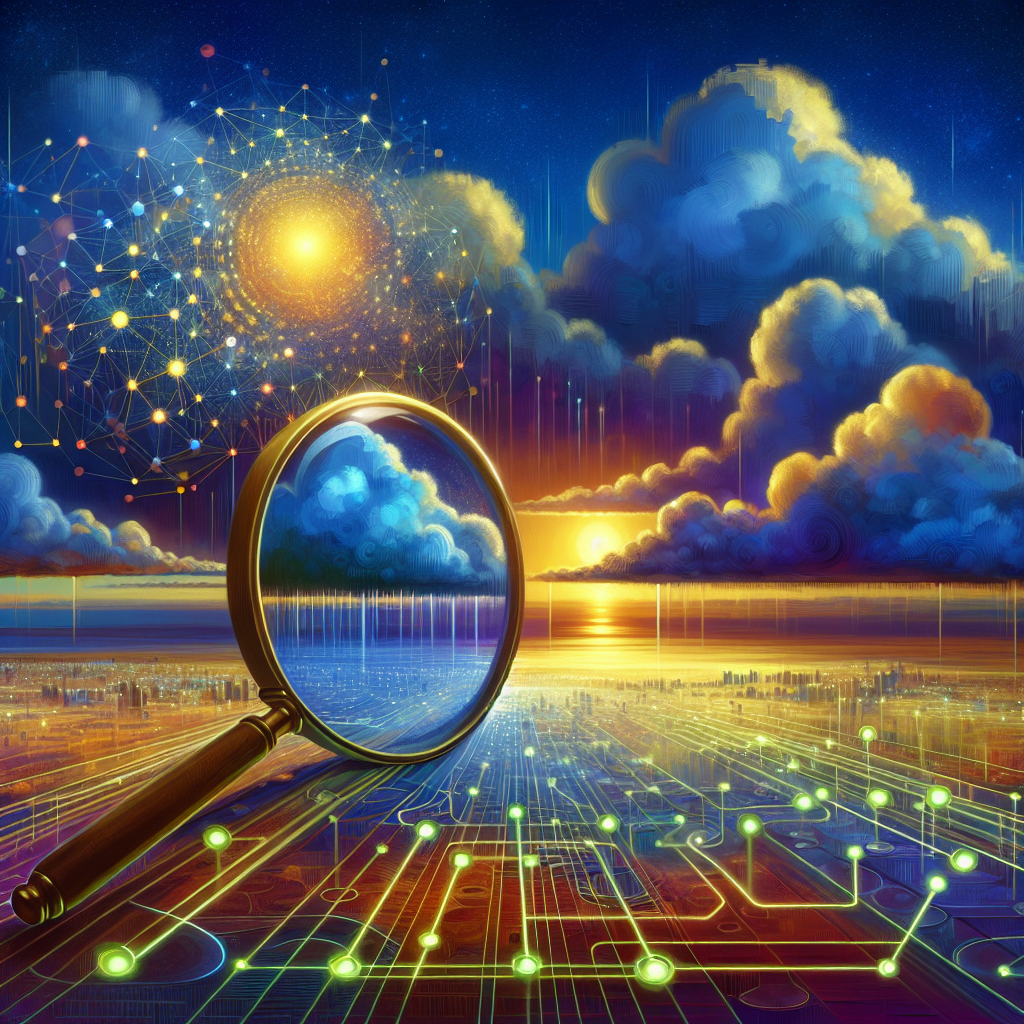
Hey there! Have you ever wondered why some companies seem to stay a step ahead in this fast-paced business world? Well, it all boils down to leveraging advanced technologies like artificial intelligence (AI). Did you know that companies using AI have seen an average revenue growth of 38% over the past three years? That’s triple what firms without AI strategies report (McKinsey & Company). At the heart of this impressive transformation are agent planning systems. These smart tools are revolutionizing decision-making processes, making it crucial for businesses to understand and effectively implement them.
In this article, we’re diving into how you can harness these powerful agent planning systems to boost your efficiency and strategic outcomes. Drawing on insights from industry leaders like OpenAI and Stanford University, as well as data-driven research, we’ll explore actionable strategies that will help integrate AI seamlessly into your operations. Plus, we’ll dive deeper into some real-world applications and future trends that could impact your business.
Understanding Agent-Based Modeling
Agent-based modeling (ABM) is a game-changing simulation technique that lets businesses replicate complex interactions within an ecosystem using autonomous agents—think of them as mini virtual experts with specific behaviors. This approach is key for organizations aiming to forecast outcomes and make smarter decisions.
Key Benefits of ABM
- Predictive Analytics: By simulating different scenarios, companies can predict market trends and consumer behavior before they happen.
- Scalability: ABMs handle large amounts of data effortlessly, making them perfect for big-scale applications.
- Flexibility: The models adapt easily to changes in the business environment.
Recent studies show that businesses using ABM report a 25% improvement in decision-making accuracy (Harvard Business Review). Understanding how agent-based modeling optimizes decision-making processes is crucial for any business looking to leverage advanced technologies effectively. In fact, many companies are integrating these models into their strategic planning efforts to navigate uncertain market conditions.
Real-World Applications of ABM
Let’s take a closer look at some industries where ABM has made significant impacts:
- Retail: Companies like Walmart use agent-based models to optimize inventory management and predict consumer demand patterns, leading to more efficient supply chain operations.
- Healthcare: In healthcare systems, ABM helps simulate the spread of diseases or the impact of health policies, aiding in effective resource allocation and crisis management.
- Finance: Financial institutions are employing ABMs to model market dynamics and assess risk by analyzing the behavior of individual agents like investors or traders.
Enhancing Agent Planning Systems with Machine Learning
Now, let’s talk about the magic of machine learning (ML). When you integrate ML techniques into your agent planning systems, you’re giving them superpowers. These enhanced agents can learn from historical data and improve their predictive capabilities over time—talk about getting smarter!
Case Study: OpenAI’s GPT-4 Integration
Take OpenAI’s development of models like GPT-4 as an example. By using neural networks, these models boost the planning algorithms’ ability to solve complex problems dynamically.
Impact on Business Operations
- Improved Efficiency: ML-enhanced agents process and analyze data 40% faster than traditional systems (Journal of Artificial Intelligence Research).
- Adaptive Learning: Agents keep learning from new data, which enhances their decision-making accuracy.
The integration of machine learning techniques into agent planning systems significantly boosts their adaptability and efficiency across various applications. For instance, financial services firms are using ML-enhanced agents to provide personalized investment advice by analyzing vast datasets in real-time.
Future Trends: The Rise of AI-Augmented Agents
Looking ahead, we anticipate a surge in the development of AI-augmented agents that can perform more complex tasks autonomously. This advancement will enable businesses to tackle intricate problems with unprecedented precision and speed. Imagine an agent planning system that not only predicts market trends but also automates strategic adjustments based on those predictions—this is where AI is headed!
Evaluating Performance Metrics
To truly make the most of your agent planning systems, you need to evaluate performance metrics carefully. This evaluation helps identify areas for improvement and ensures that strategic planning aligns with operational outcomes.
Key Performance Indicators (KPIs)
- Response Time: How quickly an agent can process information and make decisions.
- Accuracy Rate: The correctness of the agent’s decision-making process.
- Scalability Index: How well the system performs as it grows in complexity.
Research indicates that organizations regularly evaluating these metrics see a 30% increase in operational efficiency (MIT Sloan Management Review). By focusing on these KPIs, companies can enhance their strategic planning and operational outcomes significantly. Moreover, continuously monitoring these indicators allows for timely adjustments, ensuring your systems remain competitive.
Best Practices for Performance Evaluation
To effectively assess your agent planning systems, consider implementing a structured approach:
- Benchmarking: Compare your system’s performance against industry standards to identify gaps.
- Regular Audits: Conduct periodic audits of your agent systems to ensure they meet your objectives.
- Feedback Loops: Establish mechanisms for collecting feedback from users to refine and improve the system.
The Role of STRIPS in Agent Planning Systems
Have you heard of STRIPS? It stands for the Stanford Research Institute Problem Solver. This framework has been a cornerstone in shaping modern agent planning systems. Developed at Stanford University, STRIPS provides a structured approach to problem-solving by defining actions, preconditions, and effects within an environment.
Contributions to Modern AI Strategies
- Action Representation: Offers a clear syntax for describing possible actions agents can take.
- Planning Algorithms: Facilitates efficient decision-making through predefined rules and constraints.
A study conducted at Stanford University found that incorporating STRIPS-based planning algorithms increased solution accuracy by 20% in simulated environments (Stanford AI Institute). Understanding STRIPS’ contributions helps organizations implement more effective agent planning systems. Moreover, the principles of STRIPS are being expanded upon with new methodologies to address even more complex problems.
Expanding STRIPS for Complex Environments
As businesses encounter increasingly complex challenges, the foundational elements of STRIPS are evolving. Researchers at Stanford University and beyond are developing enhanced frameworks that integrate probabilistic reasoning and real-time data processing capabilities. These advancements allow agents to operate effectively in dynamic environments, where conditions can change rapidly.
Implementing Effective AI Strategies
As businesses explore integrating agent planning systems, it’s crucial to adopt strategies that maximize their potential. Here are some actionable insights for implementing these advanced technologies effectively:
Steps for Successful Implementation
- Define Clear Objectives: Start by determining what you aim to achieve with your agent planning system.
- Data Collection and Management: Ensure you have high-quality data to train your agents.
- Iterative Testing: Continuously test and refine the system based on performance metrics.
- Cross-Functional Collaboration: Involve stakeholders from different departments for comprehensive insights.
By following these steps, organizations can enhance their operational efficiency and achieve strategic objectives more effectively. Additionally, fostering a culture of innovation within your organization encourages continuous improvement and adaptation as new technologies emerge.
Overcoming Challenges in AI Integration
While the benefits are clear, integrating agent planning systems does come with its challenges:
- Data Privacy: Ensure compliance with data protection regulations when collecting and using customer information.
- Skill Gap: Invest in training programs to upskill your workforce for effective utilization of AI tools.
- Cost Management: Develop a cost-benefit analysis to justify investments in new technologies.
Conclusion
Agent planning systems are a game-changer in AI strategies, offering businesses unparalleled capabilities to optimize decision-making processes. By understanding how agent-based modeling works, integrating machine learning techniques, evaluating performance metrics, and leveraging frameworks like STRIPS, companies can significantly improve their strategic planning and operational outcomes.
Incorporating these data-driven insights not only boosts efficiency but also positions organizations to adapt quickly in a dynamic market landscape. As businesses continue to embrace advanced technologies, those that effectively implement agent planning systems will undoubtedly lead the charge toward sustainable growth and innovation.
Frequently Asked Questions
What are agent-based modeling and its benefits?
Agent-based modeling (ABM) simulates complex interactions using autonomous agents, offering predictive analytics, scalability, and flexibility. Businesses employing ABM report a 25% improvement in decision-making accuracy (Harvard Business Review).
How does machine learning enhance agent planning systems?
Machine learning integration improves the adaptability and efficiency of agent planning systems by enabling agents to learn from historical data. This enhances predictive capabilities and operational performance, with businesses seeing a 40% increase in processing speed (Journal of Artificial Intelligence Research).
What metrics should be evaluated for agent planning systems?
Key performance indicators include response time, accuracy rate, and scalability index. Regular evaluation can lead to a 30% increase in operational efficiency (MIT Sloan Management Review).
How does STRIPS contribute to modern AI strategies?
STRIPS provides a structured approach to problem-solving with clear action representation and planning algorithms, increasing solution accuracy by 20% in simulations (Stanford AI Institute).
What steps should be taken to implement agent planning systems effectively?
To successfully implement these systems:
- Define clear objectives.
- Manage high-quality data collection.
- Conduct iterative testing and refinement.
- Foster cross-functional collaboration.
Ready to Transform Your Business with AI?
Leverage the power of agent planning systems to revolutionize your business operations today. Our expertise in AI Agentic software development and AI Cloud Agents services has empowered numerous companies across various industries to implement cutting-edge solutions effectively.
We understand that each organization has unique needs, which is why we offer tailored consultations to help you harness the full potential of advanced technologies like those discussed in this article. Let’s work together to transform your business into a leader in innovation and efficiency.