Exploring Swarm Intelligence in AI Networks
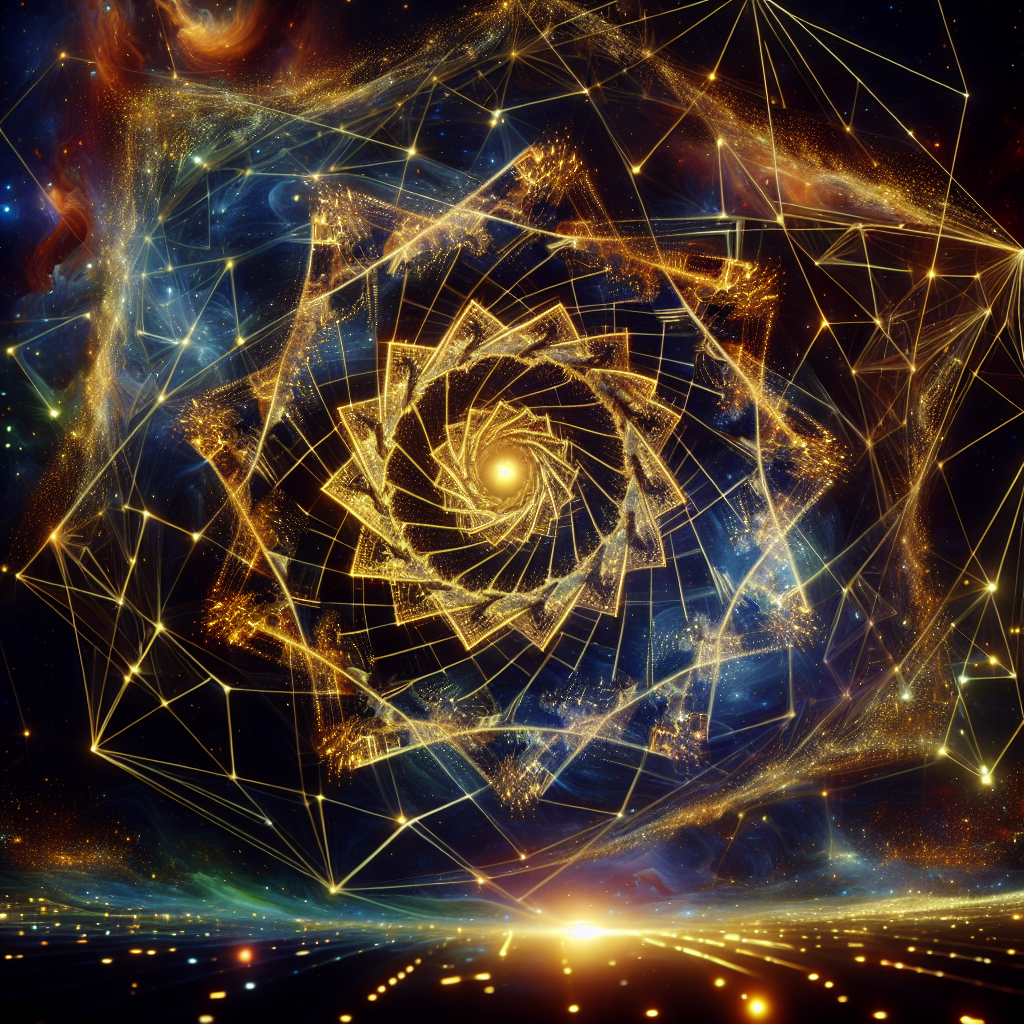
Introduction
Swarm intelligence is a transformative concept inspired by the collective behavior of social organisms like ants, bees, and birds. This innovative approach is reshaping artificial intelligence (AI) networks through decentralized network algorithms. By leveraging swarm intelligence applications, decision-making processes in autonomous systems are optimized with unprecedented efficiency and robustness. In this blog post, we’ll explore how these principles enhance AI optimization and pave the way for more adaptive and resilient technologies.
Leading institutions such as Harvard University and tech giants like Google DeepMind are spearheading research into decentralized network algorithms. Our exploration will uncover the transformative potential of swarm intelligence across various sectors, showcasing its ability to improve efficiency and robustness in AI networks. From optimizing traffic flow in urban centers to enhancing resource distribution in smart grids, the applications are vast and varied.
The Foundations of Swarm Intelligence
What is Swarm Intelligence?
Swarm intelligence involves studying collective behavior in decentralized systems, drawing inspiration from nature. Here, simple individuals following basic rules result in complex group behaviors that can be harnessed by artificial systems to solve optimization problems without centralized control or extensive computational resources.
- Decentralization: Unlike traditional AI networks relying on a central authority, swarm intelligence operates through numerous autonomous agents collaborating without direct oversight.
- Scalability: The system’s ability to scale efficiently is inherent in its design. Adding more agents typically enhances the collective problem-solving capability.
- Robustness: A key advantage of swarm systems is their resilience; even if some components fail, the network continues functioning.
Swarm Intelligence Enhances Decision-Making Processes
Swarm intelligence significantly boosts decision-making processes in autonomous systems by allowing distributed and collaborative solutions. This enhancement leads to more effective responses and adaptability within complex environments. For example, in a swarm-based traffic management system, each vehicle operates as an agent that communicates with others to optimize routes dynamically, reducing congestion and improving overall flow.
Artificial Intelligence Optimization through Swarm Principles
The integration of swarm intelligence principles improves efficiency and robustness in AI networks. These advancements lead to streamlined resource allocation, optimized network routing, and heightened performance across diverse applications. By mimicking the behavior seen in natural swarms, these systems can adapt quickly to changing conditions and demands without requiring a centralized control mechanism.
Real-World Applications
Swarm intelligence is used in fields like robotics, traffic management, energy distribution, and network optimization. In smart cities like Berlin, these principles efficiently manage traffic signals and energy resources. For instance, swarm algorithms are employed to dynamically adjust traffic lights based on real-time data from sensors and vehicles, significantly reducing wait times and emissions.
In the realm of robotics, swarm intelligence is used to coordinate groups of robots for tasks such as search-and-rescue missions or agricultural monitoring. These robotic swarms can cover large areas quickly, adapting their strategies based on environmental feedback without human intervention.
Energy distribution systems also benefit from swarm principles by optimizing grid management and load balancing. By employing decentralized algorithms, these systems can respond swiftly to fluctuations in demand and supply, ensuring consistent energy availability while minimizing waste.
Pioneering Research: Harvard University and Google DeepMind
Harvard University researchers focus on applying swarm principles to enhance the navigation capabilities of robotics and autonomous vehicles in complex environments. Their work significantly advances our understanding of decentralized AI systems by developing algorithms that allow robots to navigate efficiently through dynamic, unpredictable settings, akin to how birds flock or fish school.
Google DeepMind explores how swarm intelligence can be integrated into machine learning models to improve decision-making and problem-solving efficiency. By utilizing swarms’ adaptive nature, their research aims to push the boundaries of artificial intelligence optimization, making systems more resilient and capable in uncertain conditions.
Case Study: Autonomous Vehicles
At Google DeepMind, researchers have developed algorithms that enable groups of autonomous vehicles to communicate and coordinate seamlessly, much like a flock of birds. This innovation allows for smoother traffic flow and reduced congestion by dynamically adjusting routes based on collective data gathered from all participating vehicles.
Challenges and Future Prospects
While implementing swarm intelligence in AI systems presents challenges such as designing effective algorithms and managing computational costs during setup, ongoing research aims to streamline these processes. The future holds promising developments, including integrating swarm algorithms with quantum computing for enhanced optimization capabilities and expanding applications into diverse fields like healthcare and finance.
In healthcare, swarm intelligence could optimize resource allocation within hospitals or improve diagnostic procedures by analyzing vast datasets collaboratively. In the financial sector, these principles might enhance trading strategies through decentralized decision-making processes that adapt to market changes in real-time.
Conclusion
Swarm intelligence represents a paradigm shift in how AI networks are designed and operated. By drawing inspiration from nature, these systems enhance decision-making processes, improve efficiency, and increase robustness in autonomous technologies. Institutions like Harvard University and Google DeepMind continue to push the boundaries of swarm-based algorithms, while cities like Berlin demonstrate their practical applications on a large scale.
As we look towards the future, the integration of swarm intelligence with emerging technologies promises even greater advancements, making it an exciting area for continued research and development in artificial intelligence. The potential for swarm intelligence to revolutionize various sectors underscores its importance as a key driver of innovation in the AI landscape.