From Data to Decisions | Leveraging Generative AI in the Enterprise
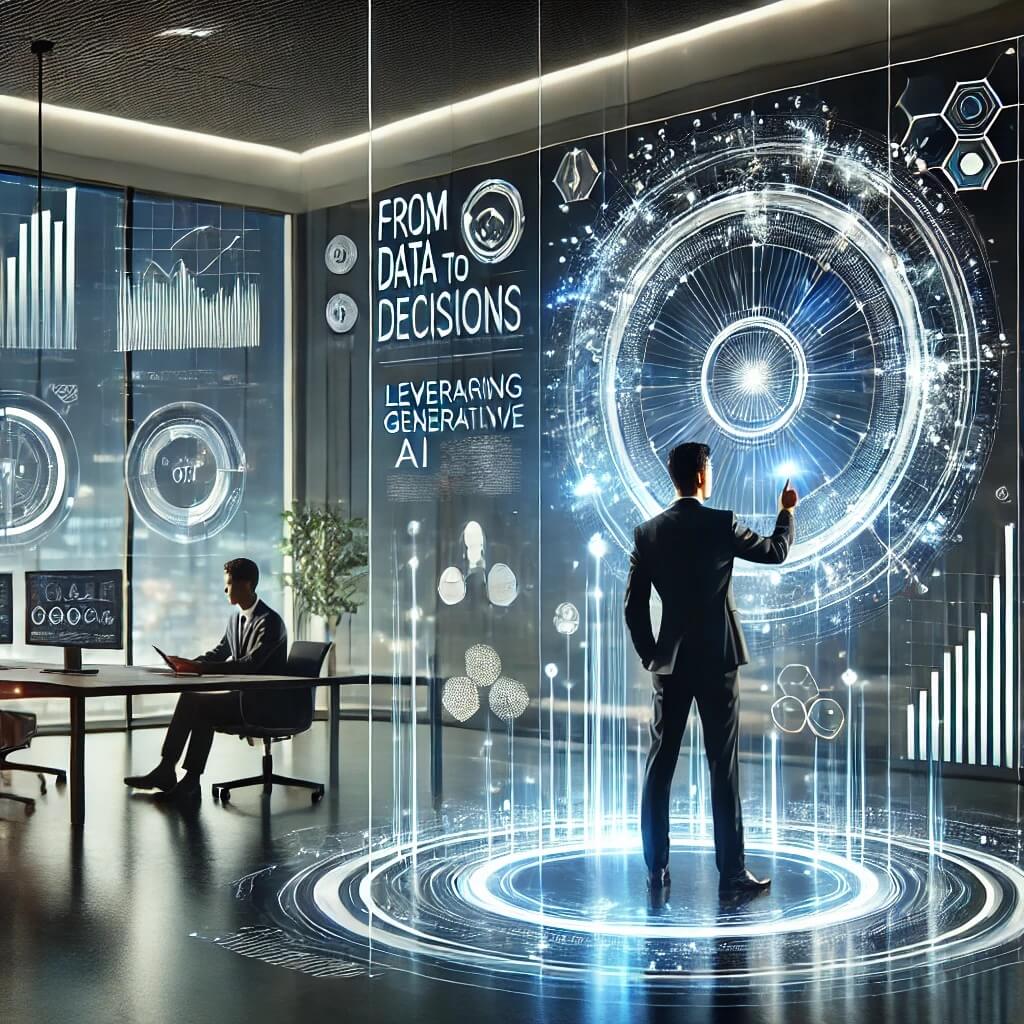
As businesses continue to navigate an increasingly complex and competitive landscape, one thing remains clear: data-driven decision making has become a critical component of success. In today’s fast-paced world, companies that can quickly analyze vast amounts of data, identify trends, and make informed decisions are better equipped to stay ahead of the curve.
However, traditional approaches to data analysis often fall short in meeting the demands of modern business. Manual data collection, processing, and analysis can be time-consuming, resource-intensive, and limited in their ability to provide actionable insights. This is where generative AI comes into play – a revolutionary technology that enables businesses to unlock new levels of value and competitiveness by leveraging the power of machine learning.
What is Generative AI?
Generative AI refers to a family of machine learning models that can generate new data, images, or even entire videos based on patterns learned from existing data. This capability has far-reaching implications for various industries, including finance, healthcare, marketing, and more. By analyzing vast amounts of data, AI generative models can identify complex relationships, predict future trends, and provide recommendations that inform business decisions.
The Promise of Generative AI
In the context of enterprise decision making, generative AI offers a compelling promise: to transform data into actionable insights that drive business value and competitiveness. By leveraging generative AI, companies can:
- Analyze vast amounts of data in real-time, identifying trends and patterns that inform strategic decisions
- Predict future outcomes with greater accuracy, enabling proactive planning and resource allocation
- Automate manual processes, freeing up resources for more strategic and high-value activities
In the following sections, we will delve deeper into the world of generative AI, exploring its applications, benefits, and challenges in the context of enterprise decision making. We will examine case studies and examples of successful Generative Machine Learning implementations, discuss key steps involved in integrating this technology into an organization’s data infrastructure, and address common concerns and misconceptions about gen AI.
The Challenges of Data-Driven Decision Making
As we discussed in the previous section, data-driven decision making has become a critical component of business success. However, traditional approaches to data analysis often fall short in meeting the demands of modern business. In this section, we will explore the current state of data management in enterprises and highlight the challenges associated with traditional approaches.
The Current State of Data Management
Many organizations still rely on manual data collection, processing, and analysis methods, which can be time-consuming, resource-intensive, and limited in their ability to provide actionable insights. This approach often leads to:
- Data silos: Information is fragmented across different departments, teams, or systems, making it difficult to access and analyze.
- Inconsistent data quality: Data may be inaccurate, incomplete, or inconsistent, leading to poor decision making.
- Limited scalability: As data volumes grow, manual processes become increasingly time-consuming and resource-intensive.
The Challenges of Traditional Approaches
Traditional approaches to data analysis often face significant challenges, including:
- Time-consuming data preparation: Manually collecting, cleaning, and formatting data can take weeks or even months.
- Lack of expertise: Organizations may not have the necessary skills or knowledge to analyze complex data sets.
- Limited insights: Manual analysis methods often provide limited insights, making it difficult to make informed decisions.
The Need for a New Approach
In today’s fast-paced business environment, organizations need a more agile and efficient approach to data analysis. This is where generative AI comes into play – a revolutionary technology that can help businesses unlock new levels of value and competitiveness by leveraging the power of machine learning.
Generative AI: A Potential Solution
Generative AI offers several benefits over traditional approaches, including:
- Faster data analysis: Gen AI models can analyze vast amounts of data in real-time, providing faster insights.
- Improved accuracy: Gen AI models can identify complex relationships and patterns in data, reducing the risk of human error.
- Increased scalability: Gen AI models can handle large volumes of data, making them ideal for organizations with growing data needs.
In our next section, we will explore what gen AI is, its key benefits and applications, and how it can be used to drive business value and competitiveness.
What is Generative AI?
In the previous sections, we introduced gen AI as a revolutionary technology that can help businesses unlock new levels of value and competitiveness by leveraging the power of machine learning. But what exactly is generative AI?
Definition and Explanation
Gen AI refers to a family of machine learning models that can generate new data, images, or even entire videos based on patterns learned from existing data. These models use complex algorithms to analyze vast amounts of data and identify relationships between variables.
Key Concepts:
- Deep Learning: Gen AI models rely on deep learning techniques, which involve training neural networks with multiple layers to learn abstract representations of data.
- Generative Models: Gen models are trained on a dataset and can generate new, synthetic data that is similar in distribution and characteristics to the original data.
Types of Generative AI Models:
There are several types of generative AI models, including:
- Generative Adversarial Networks (GANs): GANs consist of two neural networks that work together to generate new data. One network generates synthetic data, while the other network tries to distinguish between real and fake data.
- Variational Autoencoders (VAEs): VAEs are a type of generative model that uses an encoder to compress input data into a lower-dimensional representation and a decoder to reconstruct the original input from this compressed representation.
Benefits and Applications
Generative AI offers several benefits over traditional approaches, including:
- Faster Data Analysis: Gen AI models can analyze vast amounts of data in real-time, providing faster insights.
- Improved Accuracy: Gen AI models can identify complex relationships and patterns in data, reducing the risk of human error.
- Increased Scalability: Gen AI models can handle large volumes of data, making them ideal for organizations with growing data needs.
Real-World Applications:
Generative AI has a wide range of applications across various industries, including:
- Predictive Analytics: Gen AI models can be used to predict future outcomes and identify trends in data.
- Anomaly Detection: Gen AI models can detect anomalies and outliers in data, helping organizations identify potential security threats or errors.
- Image and Video Generation: Gen AI models can generate realistic images and videos, which can be used for entertainment, education, or marketing purposes.
Leveraging Generative AI in the Enterprise
In the previous sections, we introduced generative Artificial Intelligence as a revolutionary technology that can help businesses unlock new levels of value and competitiveness by leveraging the power of machine learning. We also explored what gen AI is, its key benefits and applications, and how it can be used to drive business value and competitiveness.
Benefits for Enterprises:
AI-Powered Generation offers several benefits for enterprises, including:
- Improved decision making: Gen AI models can analyze vast amounts of data in real-time, providing faster insights that inform strategic decisions.
- Increased efficiency: Gen AI models can automate manual processes, freeing up resources for more strategic and high-value activities.
- Enhanced customer experience: Gen AI models can help businesses personalize their offerings and improve customer engagement.
Real-World Applications:
AI-Powered Generation has a wide range of applications across various industries, including:
- Predictive maintenance: Gen AI models can predict when equipment is likely to fail, reducing downtime and improving overall efficiency.
- Credit risk assessment: Gen AI models can analyze vast amounts of data to assess credit risk and identify potential security threats.
- Supply chain optimization: Gen AI models can optimize supply chains by predicting demand and identifying bottlenecks.
Case Studies:
Several companies have successfully implemented gen AI solutions, including:
- Google: Google uses gen AI to improve its search results and provide more accurate information to users.
- Amazon: Amazon uses gen AI to personalize its product recommendations and improve customer engagement.
- Ford Motor Company: Ford uses gen AI to predict when equipment is likely to fail, reducing downtime and improving overall efficiency.
Key Steps for Implementation:
To successfully implement AI-Powered Generation solutions, enterprises should follow these key steps:
- Define business objectives: Clearly define the business objectives that gen AI can help achieve.
- Develop a data strategy: Develop a data strategy that includes collecting, processing, and storing relevant data.
- Choose the right model: Choose the right generative AI model for the specific use case.
- Train and validate the model: Train and validate the model using high-quality data.
- Monitor and adjust: Monitor the performance of the model and make adjustments as needed.
Addressing Concerns:
Some enterprises may be concerned about the potential risks associated with gen AI, including:
- Bias and fairness: Gen AI models can perpetuate biases and unfairness if they are trained on biased data.
- Security and compliance: Gen AI models can pose security and compliance risks if they are not properly monitored and controlled.
To address these concerns, enterprises should implement robust governance and risk management practices to ensure that generative AI solutions are used responsibly and ethically.
Addressing Concerns and Challenges
In the previous sections, we explored the benefits and applications of generative AI in the enterprise. However, like any emerging technology, AI-Powered generation also raises concerns and challenges that need to be addressed.
Concerns about Bias and Fairness
One of the most significant concerns about generative AI is its potential for bias and unfairness. If a generative model is trained on biased data, it can perpetuate those biases in its outputs, leading to discriminatory outcomes. This is particularly concerning when using gen AI for applications such as credit risk assessment or hiring decisions.
Mitigating Bias and Fairness Risks
To mitigate these risks, organizations should take several steps:
- Use diverse and representative data: Ensure that the training data used to develop the generative model is diverse and representative of the population it will be applied to.
- Regularly audit and update models: Regularly audit and update the generative model to ensure that it remains fair and unbiased.
- Use techniques such as debiasing and fairness metrics: Use techniques such as debiasing and fairness metrics to detect and mitigate bias in the generative model.
Security and Compliance Concerns
Another concern about generative Artificial Intelligence is its potential security and compliance risks. If a generative model is not properly monitored and controlled, it can be used for malicious purposes or lead to unauthorized access to sensitive data.
Mitigating Security and Compliance Risks
To mitigate these risks, organizations should take several steps:
- Implement robust governance and risk management practices: Implement robust governance and risk management practices to ensure that generative AI is developed and deployed in a responsible and compliant manner.
- Use secure and auditable frameworks: Use secure and auditable frameworks for developing and deploying generative models.
- Regularly monitor and audit model performance: Regularly monitor and audit the performance of generative models to detect any security or compliance risks.
Addressing Other Concerns
Other concerns about generative Artificial Intelligence include its potential impact on jobs, data quality, and interpretability. To address these concerns, organizations should take several steps:
- Develop clear policies and guidelines: Develop clear policies and guidelines for the development and deployment of generative models.
- Provide training and education: Provide training and education to employees about the benefits and risks of generative AI.
- Encourage transparency and accountability: Encourage transparency and accountability in the development and deployment of generative models.
Key Takeaways
- AI Generative Models can improve decision making: By analyzing vast amounts of data in real-time, generative AI models can provide faster insights that inform strategic decisions.
- AI Generative Models can increase efficiency: By automating manual processes, generative AI models can free up resources for more strategic and high-value activities.
- AI Generative Models requires careful planning and execution: To ensure successful implementation, organizations should develop clear policies and guidelines, provide training and education to employees, and encourage transparency and accountability.
Future Directions
As the technology continues to evolve, we can expect to see even more exciting developments in the field of generative AI. Some potential future directions include:
- Increased use of natural language processing: AI Generative Models may become increasingly sophisticated in their ability to understand and generate human-like language.
- Greater emphasis on explainability and transparency: As concerns about bias and fairness continue to grow, we can expect to see increased focus on developing techniques for explaining the decisions made by generative AI models.
- More widespread adoption across industries: As the benefits of generative AI become more widely understood, we can expect to see greater adoption across a wider range of industries.
Call to Action
To stay ahead of the curve and take advantage of the opportunities presented by generative AI, organizations should:
- Stay informed about the latest developments: Keep up-to-date with the latest research and advancements in the field.
- Develop clear policies and guidelines: Establish clear policies and guidelines for the development and deployment of generative models.
- Provide training and education: Provide training and education to employees about the benefits and risks of gen AI.
Conclusion
In conclusion, generative AI has the potential to revolutionize the way businesses operate by providing faster insights, improved decision making, and increased efficiency. By understanding its benefits and challenges, organizations can ensure successful implementation and take advantage of the opportunities presented by this exciting technology.