Generative AI’s Role in the Future Data Science
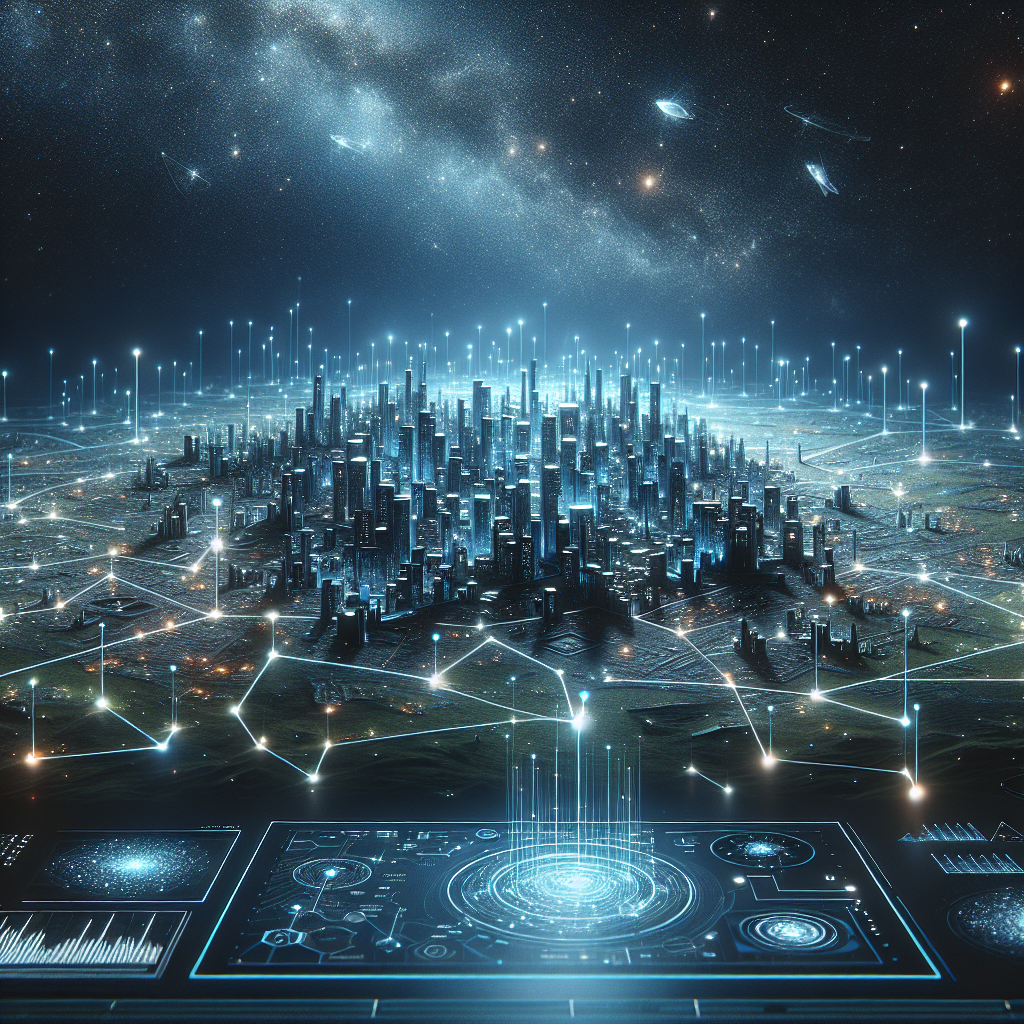
Generative AI’s Role in the Future of Data Science
The landscape of data science is undergoing a profound transformation, driven by advancements in artificial intelligence (AI). Among these developments, generative artificial intelligence stands out as a pivotal force reshaping how we understand and utilize data. As organizations like OpenAI and Google DeepMind lead the charge, the integration of generative AI models is revolutionizing predictive analytics and other key aspects of future data science applications. This blog post explores the transformative impact on data science brought by generative AI, focusing on its role in enhancing AI-driven analytics and driving machine learning advancements.
The Transformative Impact of Generative Artificial Intelligence
Generative artificial intelligence refers to models that create new data instances based on existing datasets. Unlike traditional AI focused solely on pattern recognition, generative AI can produce content such as text, images, or music that mimics human-like creativity. This capability is transforming the field of data science by expanding the horizons of what AI-driven analytics can achieve.
Enhancing Predictive Analytics
The integration of generative AI models is revolutionizing how data scientists approach predictive analytics. By providing richer datasets for analysis, these models enable a broader exploration of potential scenarios. This expanded dataset allows for more accurate predictions and refined decision-making processes. For instance, in financial forecasting, generative AI can simulate various market conditions to help analysts anticipate trends and risks more effectively.
Furthermore, generative AI facilitates the creation of synthetic data that mirrors real-world complexities, enabling models to be trained on a wider variety of scenarios without compromising privacy or security. This is particularly beneficial in sectors where access to large volumes of sensitive data is restricted, such as healthcare and finance.
Automation of Complex Data Analysis Tasks
One of the most significant benefits of generative AI is its ability to automate intricate data analysis tasks that were previously labor-intensive. As these models advance, they are set to become invaluable tools for data scientists, freeing up time and resources to focus on more strategic initiatives. This automation not only boosts productivity but also minimizes human error, leading to more reliable outcomes.
For example, in marketing analytics, generative AI can automate the generation of customer profiles based on existing data patterns. These profiles can then be used to tailor marketing strategies, enhancing customer engagement and satisfaction. By automating such processes, businesses can achieve higher efficiency and precision in their operations.
The Role of OpenAI and Google DeepMind
OpenAI and Google DeepMind are at the forefront of advancing generative AI models. Their innovations are setting new benchmarks for what is possible in future data science applications. Both organizations have made significant contributions to the field, pushing the boundaries of machine learning and artificial intelligence.
OpenAI’s Contributions
OpenAI has developed several groundbreaking models, such as GPT-3, which have demonstrated remarkable capabilities in natural language processing. These models can generate human-like text, enabling a wide range of applications from automated customer service chatbots to creative writing assistance. By providing tools that democratize access to advanced AI technologies, OpenAI is empowering developers and researchers worldwide to innovate and explore new possibilities.
Google DeepMind’s Innovations
Google DeepMind has made significant strides in areas such as reinforcement learning and neural networks. Their development of AlphaFold, a tool capable of predicting protein structures with high accuracy, exemplifies the potential of generative AI in scientific research. By accelerating discoveries in fields like biology and medicine, DeepMind’s work showcases how generative AI can drive transformative change across various domains.
Generative AI in Industry Applications
The impact of generative AI extends beyond academia and research; it is reshaping industries by enabling new capabilities and efficiencies.
Healthcare
In healthcare, generative AI is revolutionizing diagnostics and treatment planning. By generating synthetic patient data, researchers can train models to identify patterns and anomalies that may indicate disease. This not only enhances diagnostic accuracy but also facilitates the development of personalized medicine approaches tailored to individual patients’ needs.
Moreover, generative AI can simulate clinical trials, providing valuable insights into potential drug interactions and side effects without the need for extensive human testing. This accelerates the drug discovery process, potentially bringing life-saving treatments to market more quickly.
Finance
In the financial sector, generative AI is transforming risk management and fraud detection. By simulating various economic scenarios, banks can better prepare for potential downturns and optimize their investment strategies. Additionally, generative AI models can identify fraudulent transactions by recognizing patterns that deviate from typical customer behavior, enhancing security measures and protecting consumer assets.
Creative Industries
Generative AI is also making waves in creative industries such as media, advertising, and entertainment. In content creation, AI-generated scripts, music, and artwork are becoming increasingly sophisticated, offering new tools for artists and creators. These technologies can assist in generating ideas, refining drafts, and even producing final products, thereby expanding the scope of human creativity.
Addressing Ethical Considerations
As generative AI evolves, addressing ethical concerns such as data privacy and bias is crucial. Ensuring that these models generate fair and unbiased outputs while protecting user data is a significant challenge for developers and researchers. Striking the right balance between innovation and ethics will be key to harnessing the full potential of generative AI.
Data Privacy
Protecting user data is paramount, especially when generating synthetic datasets. Organizations must implement robust privacy measures to ensure that sensitive information remains secure and confidential. Techniques such as differential privacy can help mitigate risks by adding noise to data, making it difficult to trace back to individual users.
Bias Mitigation
Generative AI models are only as unbiased as the data they are trained on. It is essential to curate diverse and representative datasets to minimize biases in model outputs. Regular audits and transparency in model development can help identify and address potential biases, ensuring that generative AI technologies benefit all segments of society.
Conclusion
Generative artificial intelligence is poised to transform the field of data science profoundly. By revolutionizing predictive analytics and automating complex tasks, these models offer new opportunities for innovation across industries. Organizations like OpenAI and Google DeepMind are leading the charge, driving advancements that will shape the future of AI-driven analytics and machine learning.
As we embrace this transformative impact on data science, it is essential to consider both the opportunities and challenges presented by generative AI. By doing so, we can harness its full potential to create a more efficient, personalized, and ethical landscape in data science. The journey ahead is exciting, as generative AI continues to redefine what’s possible in future data science applications.
FAQs
What is Generative Artificial Intelligence?
Generative artificial intelligence refers to models that generate new data instances based on existing datasets. These models can create content such as text, images, or music that mimics human-like creativity.
How does generative AI impact predictive analytics?
By providing richer datasets for analysis, generative AI enables a broader exploration of potential scenarios, leading to more accurate predictions and refined decision-making processes.
What role do OpenAI and Google DeepMind play in generative AI development?
OpenAI and Google DeepMind are at the forefront of advancing generative AI models. Their innovations are setting new benchmarks for what is possible in future data science applications.
How does generative AI enhance machine learning advancements?
Generative AI provides more diverse training datasets, helping to train robust models that can generalize better across different scenarios and tasks.
What ethical considerations must be addressed with generative AI?
Addressing data privacy and bias mitigation are crucial to ensuring that generative AI technologies benefit all segments of society while protecting individual rights and freedoms.