How Generative AI Can Help You Stay Ahead of Industry Trends
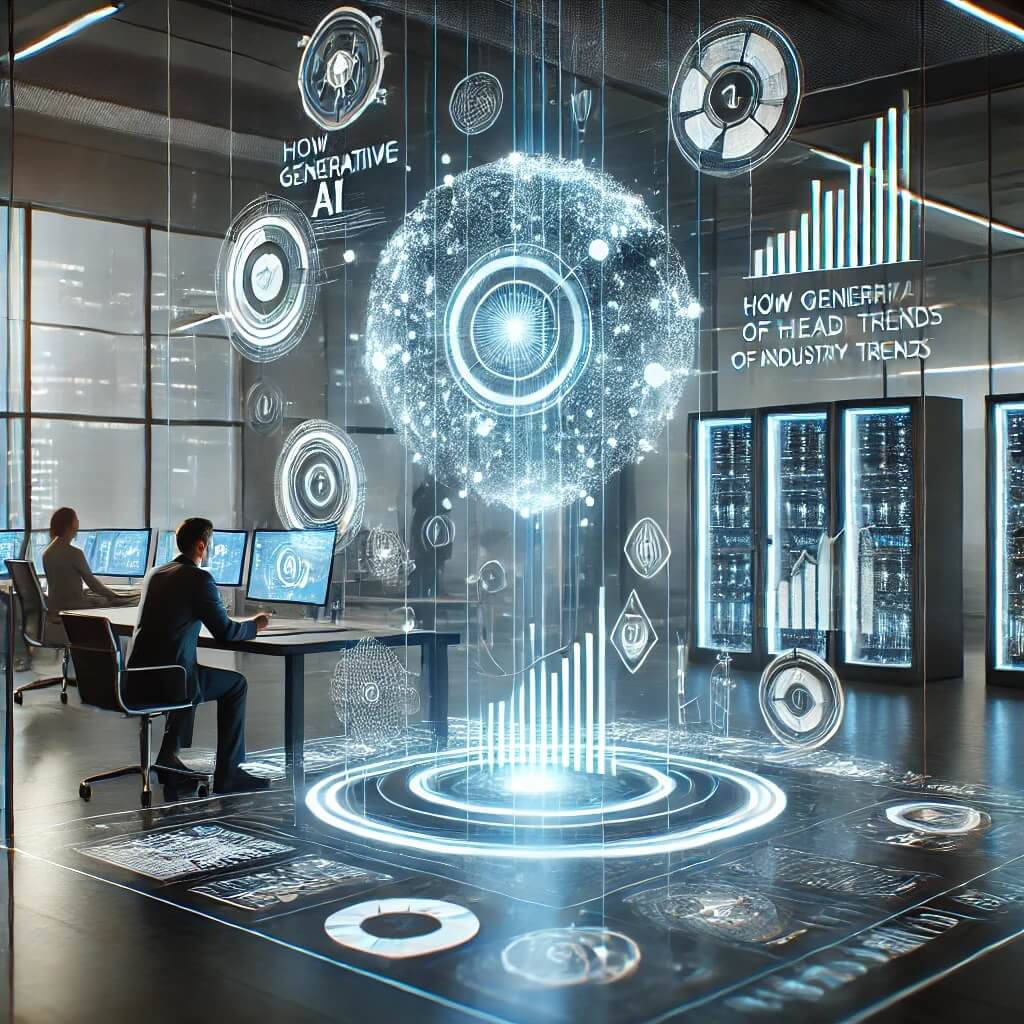
I. Introduction
Staying ahead of industry trends is crucial for businesses looking to maintain a competitive edge in today’s fast-paced and ever-changing market landscape. The ability to anticipate and adapt to emerging trends can be the difference between success and stagnation, making it essential for companies to have a strategic plan in place to stay ahead of industry trends.
In recent years, the field of artificial intelligence (AI) has experienced rapid growth and development, with generative AI being one of the most exciting and promising areas of innovation. Generative AI refers to a type of machine learning algorithm that can generate new data, products, or experiences based on patterns learned from existing data. This technology has far-reaching implications for businesses looking to stay ahead of industry trends, as it enables them to create personalized experiences, anticipate customer needs, and make predictions about future market conditions.
By leveraging generative AI, businesses can gain a significant competitive advantage and stay ahead of industry trends in several ways:
- Personalized experiences: Generative AI allows businesses to create customized experiences for their customers based on individual preferences and behavior.
- Predictive analytics: Generative AI can be used to make predictions about future market conditions, enabling businesses to anticipate emerging trends and adjust their strategies accordingly.
- Product development: Generative AI can be used to generate new product ideas and designs based on customer feedback and data analysis.
In this article, we will explore the role of generative AI in helping businesses stay ahead of industry trends. We will discuss the benefits and challenges of using generative AI, provide case studies of businesses that have successfully implemented this technology, and offer practical advice for companies looking to leverage generative AI to improve their competitive advantage.
II. Understanding Generative AI
Generative AI refers to a type of machine learning algorithm that can generate new data, products, or experiences based on patterns learned from existing data. This technology has been gaining attention in recent years due to its potential applications in various fields such as art, music, writing, and product design.
What is Generative AI?
Generative AI algorithms use a type of machine learning called deep learning to analyze patterns in data and generate new output based on those patterns. There are several types of generative AI algorithms, including:
- Generative Adversarial Networks (GANs): GANs consist of two neural networks that work together to generate new data samples. One network generates the data, while the other network tries to distinguish between the generated data and real data.
- Variational Autoencoders (VAEs): VAEs are a type of deep learning algorithm that can be used for generative modeling. They consist of an encoder that compresses the input data into a lower-dimensional representation, and a decoder that generates new output based on the compressed representation.
How Does Generative AI Work?
The process of generating new data or products using generative AI involves several steps:
- Data Collection: The first step is to collect a large dataset of existing data that can be used as input for the generative AI algorithm.
- Training: The next step is to train the generative AI algorithm on the collected data, which involves feeding the data into the algorithm and adjusting its parameters until it produces output that is similar to the original data.
- Generation: Once the algorithm has been trained, it can be used to generate new data or products based on the patterns learned from the training data.
Benefits of Generative AI
Generative AI offers several benefits for businesses looking to stay ahead of industry trends, including:
- Increased efficiency: Generative AI can automate many tasks that would otherwise require human intervention, making it possible to complete projects more quickly and efficiently.
- Improved accuracy: Generative AI algorithms can analyze large datasets and identify patterns that may not be apparent to humans, which can lead to more accurate predictions and outcomes.
- Enhanced creativity: Generative AI can be used to generate new ideas and products based on existing data, which can help businesses stay ahead of the competition.
Challenges of Generative AI
While generative AI offers many benefits for businesses, it also presents several challenges, including:
- Data quality: The quality of the output generated by a generative AI algorithm is only as good as the quality of the input data.
- Interpretability: It can be difficult to understand why a generative AI algorithm has made certain predictions or decisions, which can make it challenging to interpret its output.
- Bias: Generative AI algorithms can inherit biases present in the training data, which can lead to unfair outcomes and discrimination.
III. How Generative AI Can Help You Stay Ahead of Industry Trends
As we discussed in the previous sections, generative AI has the potential to revolutionize various industries by enabling businesses to create personalized experiences, anticipate customer needs, and make predictions about future market conditions. In this section, we will explore how generative AI can help you stay ahead of industry trends.
Personalized Experiences
Generative AI can be used to create customized experiences for customers based on their individual preferences and behavior. By analyzing large datasets, generative AI algorithms can identify patterns and anomalies that may not be apparent to humans. This information can be used to tailor marketing campaigns, product recommendations, and customer support to meet the unique needs of each customer.
Predictive Analytics
Generative AI can be used to make predictions about future market conditions, enabling businesses to anticipate emerging trends and adjust their strategies accordingly. By analyzing historical data and identifying patterns, generative AI algorithms can forecast demand, identify potential risks, and suggest opportunities for growth.
Product Development
Generative AI can be used to generate new product ideas and designs based on customer feedback and data analysis. This can help businesses stay ahead of the competition by creating innovative products that meet the evolving needs of customers.
Case Study: Netflix
Netflix is a great example of how generative AI can be used to create personalized experiences and predict user behavior. The company uses generative AI algorithms to analyze user viewing habits and recommend content based on individual preferences. This has enabled Netflix to increase engagement, reduce churn rates, and improve overall customer satisfaction.
Case Study: Amazon
Amazon is another great example of how generative AI can be used to create personalized experiences and predict user behavior. The company uses generative AI algorithms to analyze user purchasing habits and recommend products based on individual preferences. This has enabled Amazon to increase sales, reduce returns rates, and improve overall customer satisfaction.
Benefits of Using Generative AI
The benefits of using generative AI are numerous and include:
- Increased efficiency: Generative AI can automate many tasks that would otherwise require human intervention.
- Improved accuracy: Generative AI algorithms can analyze large datasets and identify patterns that may not be apparent to humans.
- Enhanced creativity: Generative AI can be used to generate new ideas and products based on existing data.
Challenges of Implementing Generative AI
While the benefits of using generative AI are numerous, there are also several challenges associated with its implementation. These include:
- Data quality: The quality of the output generated by a generative AI algorithm is only as good as the quality of the input data.
- Interpretability: It can be difficult to understand why a generative AI algorithm has made certain predictions or decisions, which can make it challenging to interpret its output.
- Bias: Generative AI algorithms can inherit biases present in the training data, which can lead to unfair outcomes and discrimination.
IV. The Role of Data Science in Generative AI
Data science plays a crucial role in the development and implementation of generative AI models. In this section, we will explore the importance of data science in generative AI and how it can be used to improve the accuracy and effectiveness of these models.
The Importance of Data Quality
The quality of the input data is critical for the success of generative AI models. High-quality data ensures that the model learns accurate patterns and relationships, which are essential for generating realistic outputs. Poor-quality data, on the other hand, can lead to inaccurate or misleading results.
Data Preprocessing and Cleaning
Before feeding data into a generative AI model, it must be preprocessed and cleaned to ensure its quality. This involves removing missing values, handling outliers, and normalizing the data to prevent bias. Data preprocessing is a critical step that requires expertise in data science to ensure accurate and reliable results.
Feature Engineering
Feature engineering is another essential aspect of data science in generative AI. Feature engineering involves selecting and extracting relevant features from the input data that are useful for generating outputs. This process requires domain knowledge and expertise in machine learning to select the most informative and relevant features.
Model Evaluation and Selection
Evaluating and selecting the right generative AI model is crucial for achieving accurate results. Data scientists use various metrics, such as mean squared error, mean absolute error, and root mean squared percentage error, to evaluate the performance of different models. The selection of the best model depends on the specific problem and dataset.
Case Study: Using Data Science to Improve Generative AI Models
A recent study demonstrated the effectiveness of using data science techniques to improve generative AI models. Researchers used a combination of feature engineering, data preprocessing, and model evaluation to improve the accuracy of a generative AI model for generating realistic images. The results showed a significant improvement in performance compared to traditional methods.
Benefits of Using Data Science in Generative AI
The benefits of using data science in generative AI include:
- Improved accuracy: Data science techniques can help improve the accuracy and effectiveness of generative AI models.
- Increased efficiency: Data science can automate many tasks, such as feature engineering and model evaluation, making it easier to develop and implement generative AI models.
- Enhanced creativity: Data science can be used to generate new ideas and products based on existing data.
Challenges of Implementing Data Science in Generative AI
While the benefits of using data science in generative AI are numerous, there are also several challenges associated with its implementation. These include:
- Data quality issues: Poor-quality data can lead to inaccurate or misleading results.
- Lack of domain knowledge: Domain experts may not have the necessary expertise in machine learning and data science to develop and implement effective generative AI models.
- High computational costs: Generative AI models can be computationally expensive, requiring significant resources to train and evaluate.
V. Case Studies: Businesses That Have Successfully Used Generative AI
In this section, we will explore case studies of businesses that have successfully used generative AI to achieve their goals.
Case Study 1: NVIDIA’s Style Transfer
NVIDIA is a leader in the field of artificial intelligence and has developed a range of products that utilize generative AI. One example is their style transfer technology, which allows users to transfer the style of one image onto another. This technology was used in a case study where researchers transferred the style of Van Gogh’s paintings onto modern cityscapes.
Benefits: The benefits of using NVIDIA’s style transfer technology include:
- Increased creativity: By allowing users to transfer styles from one image to another, this technology enables new and innovative artistic expressions.
- Improved visual appeal: The resulting images are visually striking and can be used in a range of applications, including advertising and art.
Case Study 2: Amazon’s Personalized Product Recommendations
Amazon is a leader in the field of e-commerce and has developed a range of products that utilize generative AI to provide personalized product recommendations. One example is their recommendation engine, which uses generative AI to suggest products based on individual customer behavior and preferences.
Benefits: The benefits of using Amazon’s recommendation engine include:
- Increased sales: By providing customers with personalized product recommendations, this technology enables businesses to increase sales and revenue.
- Improved customer satisfaction: The resulting recommendations are tailored to individual customer needs and preferences, leading to improved customer satisfaction.
Case Study 3: Google’s AI-Generated Art
Google is a leader in the field of artificial intelligence and has developed a range of products that utilize generative AI. One example is their AI-generated art project, which uses generative AI to create new and innovative artworks based on individual user input.
Benefits: The benefits of using Google’s AI-generated art project include:
- Increased creativity: By allowing users to interact with the system and provide input, this technology enables new and innovative artistic expressions.
- Improved visual appeal: The resulting images are visually striking and can be used in a range of applications, including advertising and art.
Common Themes:
While the case studies above are diverse, there are several common themes that emerge:
- Increased creativity: Generative AI enables businesses to create new and innovative artistic expressions.
- Improved visual appeal: The resulting images are visually striking and can be used in a range of applications, including advertising and art.
- Increased sales: By providing customers with personalized product recommendations, generative AI enables businesses to increase sales and revenue.
Challenges:
While the benefits of using generative AI are numerous, there are also several challenges associated with its implementation. These include:
- Data quality issues: Poor-quality data can lead to inaccurate or misleading results.
- Lack of domain knowledge: Domain experts may not have the necessary expertise in machine learning and data science to develop and implement effective generative AI models.
VI. Conclusion
In conclusion, generative AI is a rapidly evolving field that has the potential to revolutionize various industries by enabling businesses to create personalized experiences, anticipate customer needs, and make predictions about future market conditions.
The case studies presented in this article demonstrate how businesses can leverage generative AI to achieve their goals and improve their competitive advantage. From style transfer technology to personalized product recommendations and AI-generated art, the possibilities are endless.
While there are challenges associated with implementing generative AI, such as data quality issues and lack of domain knowledge, these can be overcome by investing in education and training for employees and staying up-to-date with the latest advancements in this field.
As we move forward, it is essential to recognize the potential of generative AI and its ability to transform businesses and stay ahead of industry trends. By embracing this technology and working together, we can unlock new possibilities and create a brighter future for all.
Key Takeaways:
- Generative AI has the potential to revolutionize various industries by enabling businesses to create personalized experiences, anticipate customer needs, and make predictions about future market conditions.
- Case studies demonstrate how businesses can leverage generative AI to achieve their goals and improve their competitive advantage.
- Challenges associated with implementing generative AI include data quality issues and lack of domain knowledge.
- Investing in education and training for employees and staying up-to-date with the latest advancements in this field are essential for overcoming these challenges.
Recommendations:
- Businesses should invest in research and development to explore the potential applications of generative AI in their industry.
- Companies should develop a clear strategy for implementing generative AI, including establishing a team dedicated to exploring its possibilities.
- Employees should be educated and trained on the latest advancements in generative AI to ensure they can effectively utilize this technology.
Future Directions:
- Generative AI is expected to become increasingly integrated into various industries, including healthcare, finance, education, and entertainment.
- The development of more sophisticated algorithms and improved data quality will continue to drive innovation in this field.
- As generative AI continues to evolve, we can expect to see new applications emerge that will transform businesses and industries.