Human-in-the-Loop – Revolutionizing Data Science Applications
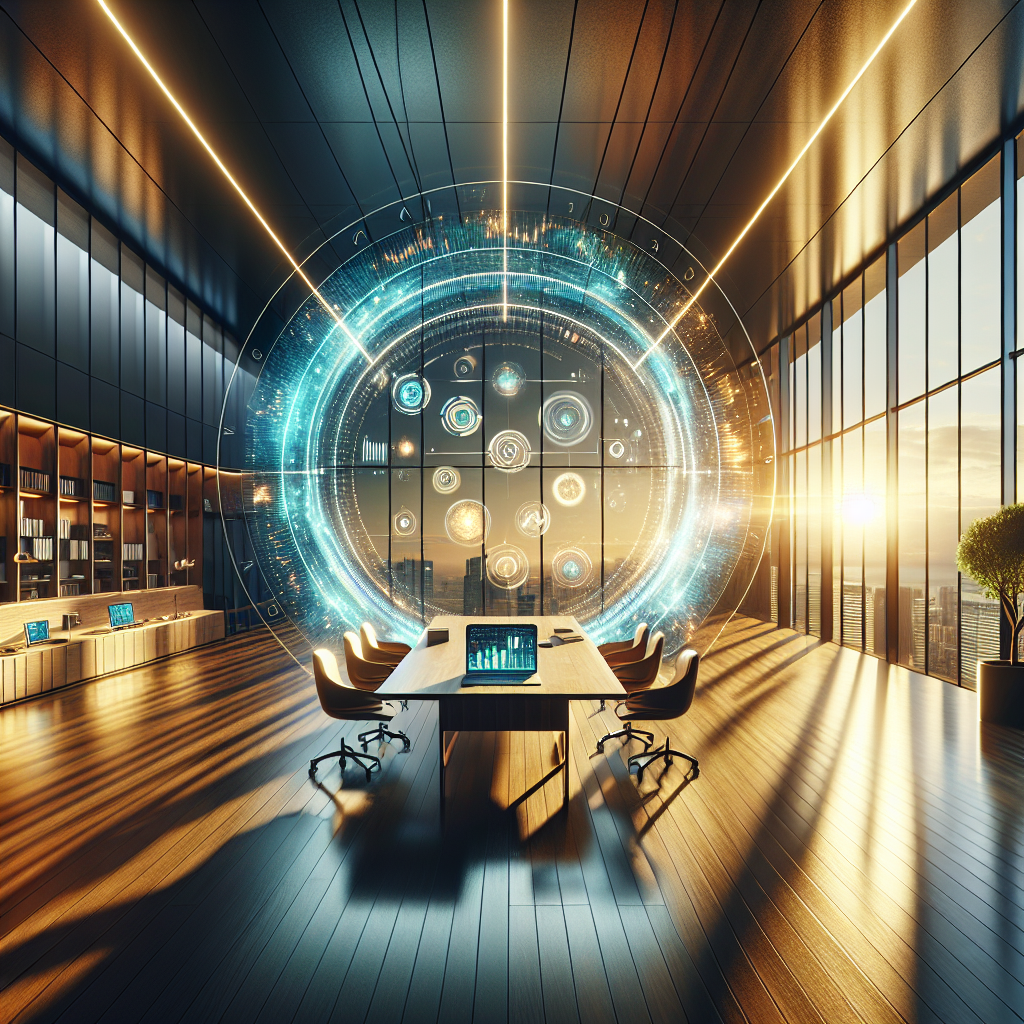
In an era where artificial intelligence (AI) and machine learning are revolutionizing industries worldwide, a transformative approach is emerging—human-in-the-loop data science. This methodology marries AI’s computational prowess with human intuition, creating collaborative systems that drive innovation and efficiency. Leading organizations like Google DeepMind, IBM Watson, and Stanford University are at the forefront of this movement, demonstrating how AI model collaboration with humans can lead to groundbreaking advancements.
What is Human-in-the-Loop Data Science?
Human-in-the-loop data science involves continuous interaction between humans and machine learning systems. This approach emphasizes a symbiotic relationship where human judgment enhances algorithmic accuracy and performance. By integrating real-time feedback from users, these systems refine predictive models, allowing for more adaptive and precise outcomes.
The Core of AI Model Collaboration with Humans
At the heart of human-in-the-loop methodologies lies the principle of collaboration between AI models and humans. This partnership ensures that while machines handle vast datasets and complex calculations, humans provide context, insight, and ethical oversight. Such collaboration not only improves model accuracy but also enhances trust in AI systems.
Enhancing Algorithmic Accuracy
One of the most significant benefits of human-in-the-loop data science is its ability to enhance algorithmic accuracy. By involving human judgment in the development process, these systems can identify and correct errors that machines might overlook. This leads to more reliable and effective predictive models, which are crucial across various industries.
Key Applications of Human-in-the-Loop Methodologies
Human-in-the-loop methodologies have a broad range of applications, demonstrating their versatility and impact:
Interactive Machine Learning
Interactive machine learning is one of the most exciting applications of human-in-the-loop data science. Here, humans actively participate in training AI models by providing feedback during the learning process. This real-time interaction allows for quicker adjustments and more accurate model development.
- Case Study: Google DeepMind
At Google DeepMind, interactive machine learning is used to create advanced algorithms that can learn from human input. This approach has been instrumental in developing AI systems capable of complex problem-solving tasks, such as mastering games like Go and chess at superhuman levels. The collaboration between humans and AI models here showcases the potential for creating more intelligent and adaptive systems.
Healthcare Diagnostics
In healthcare, the utilization of real-time feedback from users to refine predictive models is proving invaluable. Human-in-the-loop methodologies enable more accurate diagnostics and personalized treatment plans by combining machine learning’s data processing capabilities with human medical expertise.
- Example: IBM Watson in Oncology
IBM Watson uses human-in-the-loop approaches to assist oncologists in diagnosing cancer. By integrating real-time feedback from healthcare professionals, Watson provides more precise recommendations for patient care, improving outcomes and treatment efficacy. This collaboration ensures that AI systems are not only data-driven but also contextually aware, enhancing their utility in critical fields like medicine.
Customer Service Solutions
Customer service is another domain where human-in-the-loop methodologies shine. By involving customer service representatives in the training of chatbots and virtual assistants, companies can ensure these tools provide more accurate and empathetic responses to customer inquiries.
- Example: Enhancing Chatbot Efficiency
A leading e-commerce platform implemented a human-in-the-loop system for its customer support chatbot. Representatives were involved in reviewing and correcting the bot’s interactions with customers. This process allowed the AI to learn from real-world scenarios, significantly improving response accuracy and customer satisfaction. The result was a more efficient customer service operation that reduced response times and increased resolution rates.
Challenges and Considerations
While human-in-the-loop methodologies offer numerous benefits, they also come with challenges that need careful consideration:
Resource Intensity
Involving humans in the AI training process can be resource-intensive. It requires skilled personnel to provide feedback and oversee model development. Organizations must balance these needs with their available resources to ensure sustainable implementation.
Ensuring High-Quality Human Input
The quality of human input is crucial for the success of human-in-the-loop systems. Inaccurate or biased feedback can lead to flawed models. Therefore, it’s essential to have robust processes in place to verify and validate human contributions.
The Future of Human-in-the-Loop Data Science
As technology continues to evolve, so too will the methodologies surrounding human-in-the-loop data science:
Technological Advancements
Future advancements may include more sophisticated interfaces for human-AI interaction, making it easier for non-experts to contribute effectively. Additionally, developments in natural language processing could enhance how humans and AI communicate, leading to even more seamless collaboration.
Broader Industry Adoption
As industries recognize the benefits of human-in-the-loop methodologies, we can expect broader adoption across various sectors. This widespread use will likely drive innovation and improve outcomes in fields ranging from finance to environmental science.
Conclusion
Human-in-the-loop data science represents a significant shift in how AI systems are developed and deployed. By leveraging human judgment to enhance algorithmic accuracy and performance, this approach fosters more reliable, ethical, and effective AI solutions. As organizations like Google DeepMind, IBM Watson, and Stanford University continue to pioneer these methodologies, the potential for innovation and improvement across industries is vast.
Leading institutions are already paving the way, demonstrating the transformative potential of human-in-the-loop data science. By embracing this approach, organizations can harness the full power of AI while ensuring that technology serves humanity’s best interests.
Frequently Asked Questions (FAQs)
What is human-in-the-loop data science?
Human-in-the-loop data science involves continuous interaction between humans and machine learning systems to enhance algorithmic accuracy and performance. This collaboration allows for more adaptive and precise predictive models.
How does human-in-the-loop improve AI model accuracy?
By involving human judgment in the development process, human-in-the-loop methodologies help identify and correct errors that machines might overlook. This leads to more reliable and effective AI models.
What are some key applications of human-in-the-loop methodologies?
Key applications include interactive machine learning, healthcare diagnostics, and customer service solutions. These areas benefit from real-time feedback from users, leading to improved outcomes and performance.
What challenges exist in scaling human-in-the-loop systems?
Challenges include the resource-intensive nature of involving humans in training processes and ensuring the quality of human input. Addressing these issues is crucial for broader adoption.
What does the future hold for human-in-the-loop data science?
The future promises technological advancements like augmented reality (AR) and virtual reality (VR), automated feedback systems, and wider adoption across industries. These developments will enhance decision-making processes and drive innovation.