Implementing Generative AI for Advanced Manufacturing Processes
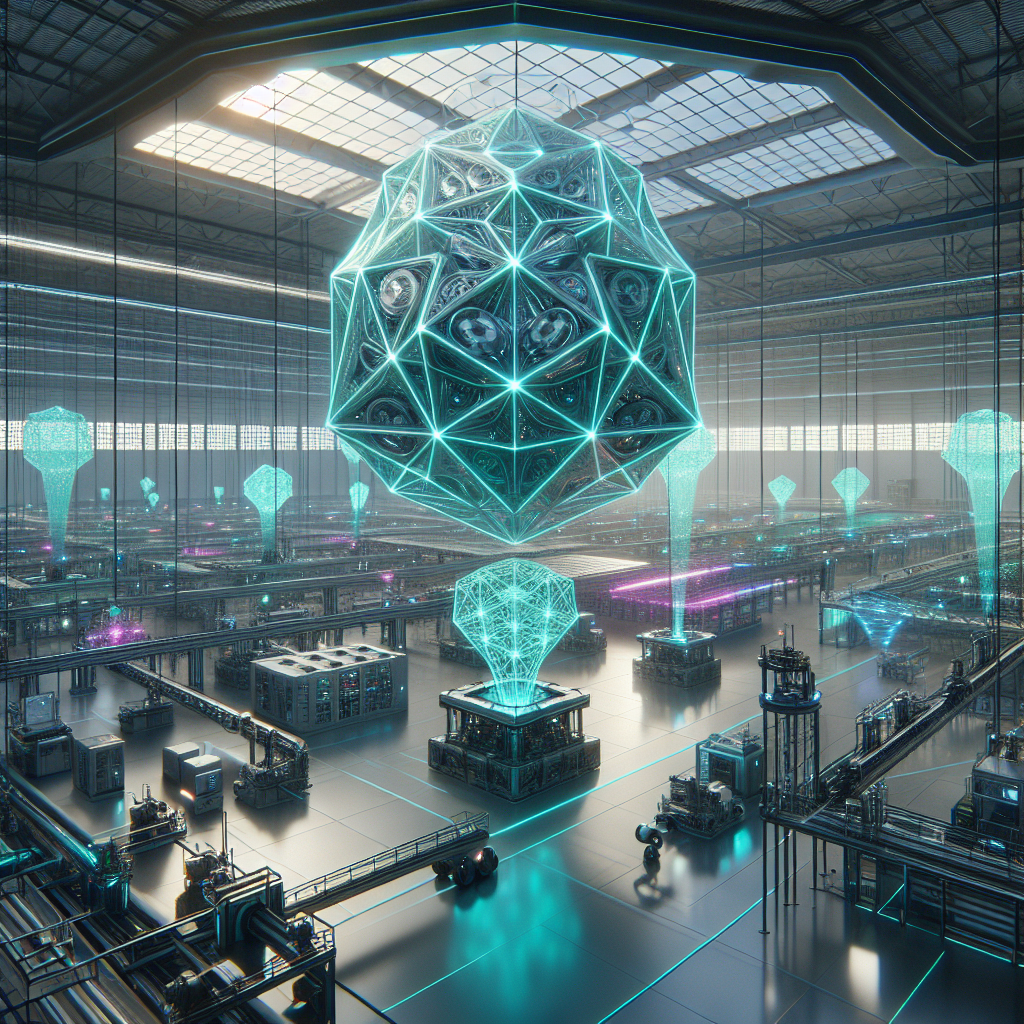
Implementing Generative AI for Advanced Manufacturing Processes
The manufacturing industry is undergoing a transformative phase, propelled by advanced technologies like generative AI, industrial automation, and smart factories. As companies strive to enhance efficiency, reduce costs, and improve product quality, implementing generative AI in manufacturing processes has emerged as a strategic imperative.
In this comprehensive guide, we explore how generative AI can revolutionize manufacturing operations, delve into its integration with industrial automation, and discuss the role of smart factories in harnessing these technologies. By understanding the nuances of generative AI manufacturing, businesses can unlock new levels of innovation and competitiveness.
Introduction
The advent of generative AI has brought forth unprecedented opportunities for industries worldwide, particularly in advanced manufacturing. Unlike traditional AI models that analyze existing data to make predictions or decisions, generative AI algorithms create new designs by identifying patterns within datasets. This capability allows for innovative solutions across various sectors, enhancing productivity and efficiency.
Integrating generative AI with industrial automation leads to smarter production lines, optimizing processes from the ground up. Companies like Siemens, General Electric, and Ford Motor Company are already exploring these advancements, showcasing the potential of generative AI manufacturing. Smart factories further complement this integration by creating intelligent environments that adapt to new technological inputs.
Innovations in additive manufacturing with generative design techniques have enabled manufacturers to create complex structures that were previously unattainable, leading to more efficient and sustainable production methods. This guide will delve into the transformative impact of generative AI on manufacturing processes, highlighting real-world applications and future possibilities.
The Power of Generative AI Manufacturing
Generative AI manufacturing offers a plethora of benefits, driving industries toward unprecedented efficiency and innovation. By utilizing advanced machine learning algorithms, these systems can create optimized designs that improve both cost-effectiveness and performance.
Design Optimization
One of the standout capabilities of generative AI is its ability to optimize designs in ways traditional methods cannot match. In sectors like automotive and aerospace, companies such as Ford Motor Company are leveraging generative design techniques to develop components that meet strict safety standards while minimizing weight and material usage. This not only enhances fuel efficiency but also reduces manufacturing costs.
Process Innovation
Generative AI is revolutionizing how production lines operate by integrating seamlessly with industrial automation systems. The use of robotics and AI in optimizing production lines allows for greater precision and flexibility, leading to significant time savings and reduced waste. Siemens has been at the forefront of this integration, demonstrating how advanced analytics can streamline operations and enhance output quality.
The Role of Smart Factories
Smart factories represent the convergence of IoT technology, data analytics, and generative AI within a manufacturing context. These environments utilize real-time data to optimize production processes dynamically, ensuring minimal downtime and maximum efficiency. By incorporating predictive maintenance, smart factories preemptively address equipment failures before they occur, significantly reducing operational disruptions.
Enhanced Decision-Making
With the integration of AI-driven insights, decision-makers in smart factories can make informed choices that drive productivity and innovation. Real-time data analytics allow for quick adjustments to production parameters, adapting to changes in demand or supply chain conditions with minimal lag time.
Advancements in Additive Manufacturing
Additive manufacturing, or 3D printing, has experienced a significant boost through the application of generative design techniques. These advancements enable manufacturers to produce parts that are lighter and stronger than those made using traditional methods. Generative AI algorithms can explore countless iterations quickly, identifying optimal designs for specific applications without the constraints typically faced by human designers.
Customization and Complexity
The ability to customize products at scale is one of the standout benefits offered by advanced additive manufacturing techniques. Complex geometries that are difficult or impossible to achieve with conventional manufacturing methods can be produced efficiently using generative AI, opening new avenues for innovation in sectors like aerospace and medical devices.
Industry Applications and Case Studies
Automotive Manufacturing
In automotive manufacturing, companies such as Ford Motor Company have successfully employed generative AI to redesign components, achieving significant weight reductions without compromising safety or performance. This not only reduces fuel consumption but also enhances the overall driving experience by improving vehicle dynamics.
Aerospace Engineering
The aerospace industry is another sector benefiting from generative AI in additive manufacturing. By optimizing the design of aircraft components for weight and strength, companies can produce lighter airframes that consume less fuel, reducing operational costs and environmental impact.
Electronics Production
In electronics production, generative AI aids in designing more efficient circuit boards. These optimized designs help minimize space usage while maximizing functionality, contributing to the development of smaller yet more powerful electronic devices.
Overcoming Challenges in Generative AI Implementation
While the potential benefits are substantial, several challenges need addressing for widespread adoption of generative AI in manufacturing:
Data Quality and Availability
The effectiveness of generative AI is heavily dependent on the quality and quantity of data available. Companies must invest in robust data collection and management systems to ensure that their AI models have access to comprehensive datasets.
Integration with Existing Systems
Seamlessly integrating generative AI technologies with current industrial processes can be complex. Manufacturers need to adopt flexible IT infrastructure and encourage cross-departmental collaboration to overcome these hurdles effectively.
The Future of Generative AI in Manufacturing
The future of manufacturing lies in the continued evolution and integration of generative AI technologies. As algorithms become more sophisticated, their ability to drive innovation will only increase. Companies that embrace this technology early on are likely to gain a competitive edge by improving efficiency, reducing costs, and delivering superior products.
Sustainability and Ethical Considerations
As generative AI continues to transform manufacturing processes, sustainability and ethical considerations must also be prioritized. By optimizing resource use and minimizing waste, generative AI can contribute significantly to more sustainable production practices.
Conclusion
The integration of generative AI into advanced manufacturing processes marks a significant leap forward for industries worldwide. By combining generative AI with industrial automation and smart factory technologies, manufacturers can achieve unprecedented levels of efficiency, innovation, and quality. As companies like Siemens, General Electric, and Ford Motor Company continue to explore these cutting-edge solutions, they not only stay competitive but also lead the charge in shaping the future of manufacturing. The journey towards a fully automated, intelligent manufacturing ecosystem is just beginning, with generative AI at its core.
Frequently Asked Questions
1. What exactly is generative AI?
Generative AI refers to machine learning algorithms designed to create new data or content by identifying patterns in existing datasets. Unlike traditional AI, which focuses on analysis and prediction, generative AI can generate novel solutions or designs autonomously.
2. How does generative AI improve manufacturing processes?
By optimizing designs and integrating with industrial automation systems, generative AI enhances productivity, reduces waste, and enables the creation of complex structures that were previously unattainable.
3. What role do smart factories play in this integration?
Smart factories are equipped with IoT devices and advanced analytics to monitor and adjust production processes continuously. This creates a dynamic environment where resources are optimized, leading to greater efficiency and adaptability.
4. How does additive manufacturing benefit from generative design techniques?
Additive manufacturing benefits from these techniques by allowing for efficient material use, customization, and the creation of complex designs that improve product performance while reducing costs.
5. Can you provide examples of industries using generative AI?
Industries such as automotive, aerospace, and electronics are actively leveraging generative AI to enhance design optimization, production processes, and overall efficiency. Companies like Ford Motor Company, General Electric, and Siemens are at the forefront of these advancements, driving innovation across their respective fields.
6. What are some challenges associated with implementing generative AI in manufacturing?
Challenges include ensuring data quality and availability, integrating new technologies with existing systems, and addressing potential workforce disruptions as roles evolve to meet the demands of an increasingly automated environment. Overcoming these obstacles requires strategic planning, investment, and a commitment to ongoing learning and adaptation.