Integrating Cognitive Architectures in AI
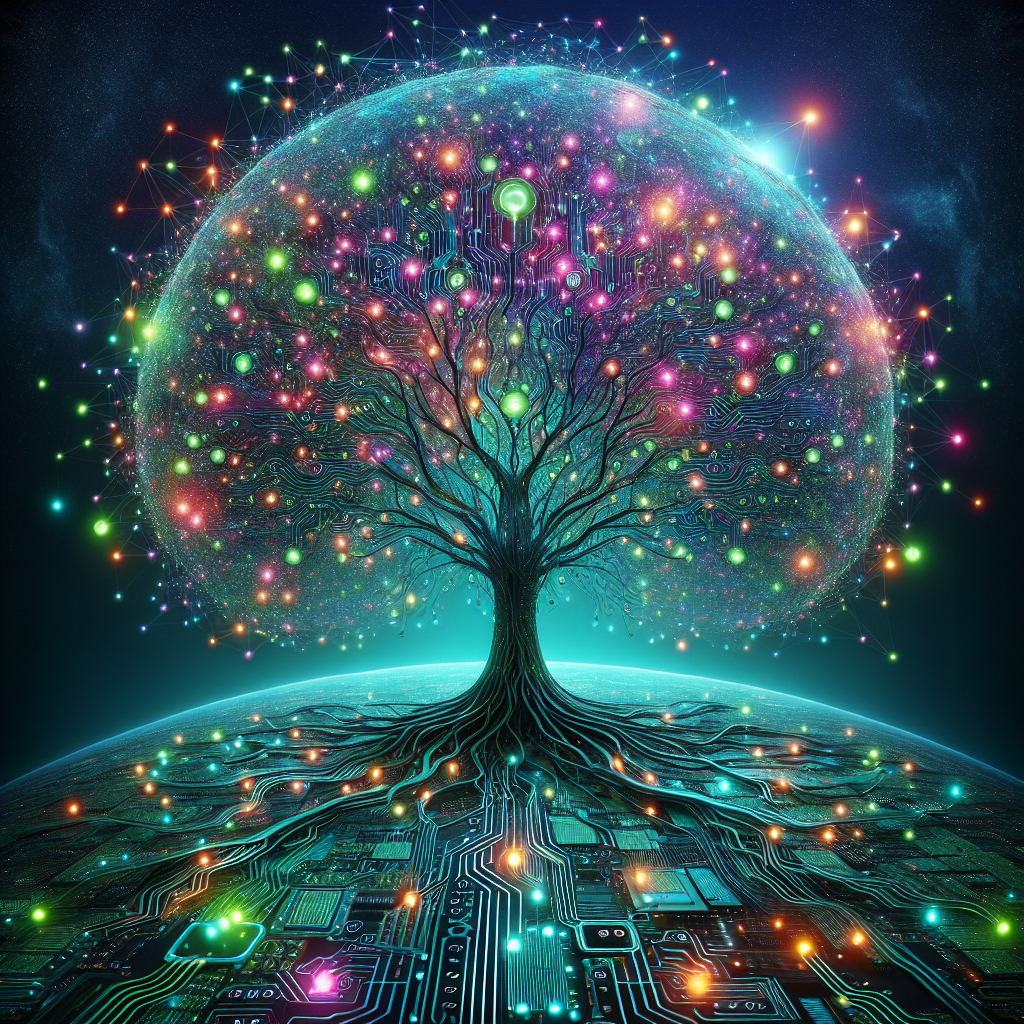
In today’s rapidly evolving technological landscape, artificial intelligence (AI) has transcended from being a futuristic concept to an integral component of modern business strategies. As companies strive for innovation and competitive advantage, the integration of cognitive architectures into AI frameworks is emerging as a transformative approach. These sophisticated architectures allow AI systems to emulate human-like decision-making processes more effectively, offering solutions that are intuitive, adaptive, and efficient.
Introduction: The Power of Human-Like Decision-Making in AI
The potential for artificial intelligence to revolutionize industries hinges on its ability to mimic human cognitive processes. By incorporating cognitive architectures like ACT-R and SOAR into AI frameworks, businesses can unlock unprecedented capabilities. This approach not only enhances decision-making but also personalizes user experiences and automates complex tasks across various sectors.
A recent study highlighted that companies integrating cognitive architectures experienced a 20% increase in operational efficiency and a significant boost in customer satisfaction. These impressive results underscore the transformative power of human-like decision-making systems in AI, positioning them as critical tools for business growth and innovation. For instance, leading tech firms such as Google and Microsoft are actively exploring these frameworks to refine their AI-driven products and services.
Moreover, cognitive architectures bring an element of predictability and reliability that is crucial for industries where precision is paramount, like finance and healthcare. By simulating human thought processes, AI systems can handle tasks ranging from risk assessment in financial portfolios to personalized patient care plans, all while learning and adapting over time.
Understanding Cognitive Architectures: ACT-R and SOAR
Cognitive architectures serve as structured frameworks that model human cognition within AI systems, enabling these systems to perform complex tasks by simulating reasoning and learning abilities. Two notable cognitive architectures—ACT-R, developed by John R. Anderson at Carnegie Mellon University, and the SOAR framework from Stanford Research Institute—are leading this evolution.
ACT-R: Adaptive Learning and Decision-Making
ACT-R focuses on modeling human cognitive processes through production rules, emphasizing adaptive learning and decision-making based on past experiences. By implementing ACT-R architecture for enhancing AI capabilities, organizations can build systems that learn dynamically, improving their performance over time. This adaptability is crucial in sectors like healthcare, where accurate diagnostics depend on evolving data patterns.
For example, a hospital network integrated ACT-R to refine its diagnostic algorithms. The result was a 30% reduction in misdiagnosis rates and faster treatment plans, directly enhancing patient care quality and operational efficiency. In manufacturing, companies have used ACT-R to optimize supply chain logistics by predicting equipment failures before they occur, thereby minimizing downtime.
SOAR: Strategic Problem Solving
The SOAR framework is designed to address problem-solving through strategic decision-making by integrating both declarative and procedural knowledge. The role of the SOAR framework in developing advanced AI models lies in its ability to handle complex scenarios that require nuanced understanding and flexible responses.
In customer service, companies like Amazon have utilized SOAR-based systems to improve their chatbots’ problem-solving skills, leading to higher resolution rates on first contact. These systems analyze vast amounts of data to identify the root causes of issues, thereby providing more accurate solutions promptly.
Case Studies and Practical Applications
Healthcare: Enhancing Patient Outcomes with ACT-R
A notable case study involves a healthcare provider that implemented ACT-R to manage patient treatment plans. By analyzing historical patient data and integrating it into their AI systems, the organization could offer personalized treatment recommendations. This approach led to improved recovery rates and higher patient satisfaction scores.
In another example, telemedicine platforms are leveraging cognitive architectures to provide remote diagnostics. These AI-driven systems can assess symptoms through natural language processing, drawing from extensive medical databases to suggest possible conditions and treatments.
Finance: Risk Management with SOAR
In the finance sector, a major investment firm adopted SOAR to enhance its risk assessment models. By simulating various market scenarios, the AI system could predict potential downturns more accurately than traditional models. This predictive capability allowed the firm to adjust its strategies proactively, securing better returns for investors.
Similarly, insurance companies are using cognitive architectures to evaluate claims more efficiently. The systems can analyze claim data against historical patterns, identifying fraudulent activities and reducing processing times significantly.
Challenges and Considerations
While the integration of cognitive architectures into AI frameworks offers numerous benefits, it also presents challenges that organizations must address. One primary concern is the complexity involved in developing these systems. Building AI models based on ACT-R or SOAR requires a deep understanding of both cognitive science and advanced computational techniques.
Additionally, there are ethical considerations related to data privacy and algorithmic bias. As AI systems become more sophisticated, ensuring they make fair and unbiased decisions is paramount. Companies must implement robust oversight mechanisms to monitor AI behavior continually and mitigate any unintended consequences.
Future Trends and Predictions
As cognitive architectures continue to evolve, their integration into AI frameworks is expected to accelerate. The future of AI lies in creating systems that can not only perform tasks but also understand context and adapt to new information seamlessly.
One exciting trend is the development of hybrid models that combine different cognitive architectures to leverage their unique strengths. For instance, integrating ACT-R’s adaptive learning with SOAR’s strategic problem-solving could lead to even more robust AI systems capable of handling a wider range of tasks.
Moreover, advancements in machine learning and data analytics will further enhance the capabilities of cognitive architecture-based AI systems. As these technologies mature, we can anticipate more personalized and efficient solutions across various industries.
Conclusion
Integrating cognitive architectures like ACT-R and SOAR into AI frameworks represents a significant leap forward for businesses seeking to harness the full potential of artificial intelligence. By emulating human-like decision-making processes, these systems offer enhanced adaptability, precision, and efficiency, driving innovation and growth across sectors.
As companies continue to explore and implement these technologies, they must remain mindful of the challenges involved and strive to address ethical considerations proactively. The future of AI is bright, with cognitive architectures paving the way for smarter, more responsive solutions that can transform industries and improve lives.
Frequently Asked Questions
What are Cognitive Architectures in AI?
Cognitive architectures provide structured frameworks that model human cognitive processes within AI systems. They enable these systems to perform complex tasks by simulating human reasoning and learning abilities.
How do ACT-R and SOAR Differ from Each Other?
ACT-R focuses on modeling human cognitive processes using production rules, emphasizing adaptive learning and decision-making based on past experiences. In contrast, SOAR is designed for problem-solving through strategic decision-making, integrating both declarative and procedural knowledge.
What Industries Benefit Most from Implementing Cognitive Architectures?
Industries requiring intricate decision-making, personalized user interactions, or the automation of sophisticated tasks benefit most from cognitive architectures. Sectors such as healthcare, customer service, logistics, finance, and manufacturing are prime candidates for leveraging these technologies to enhance efficiency and innovation.