Leveraging RAG for Advanced NLP Solutions
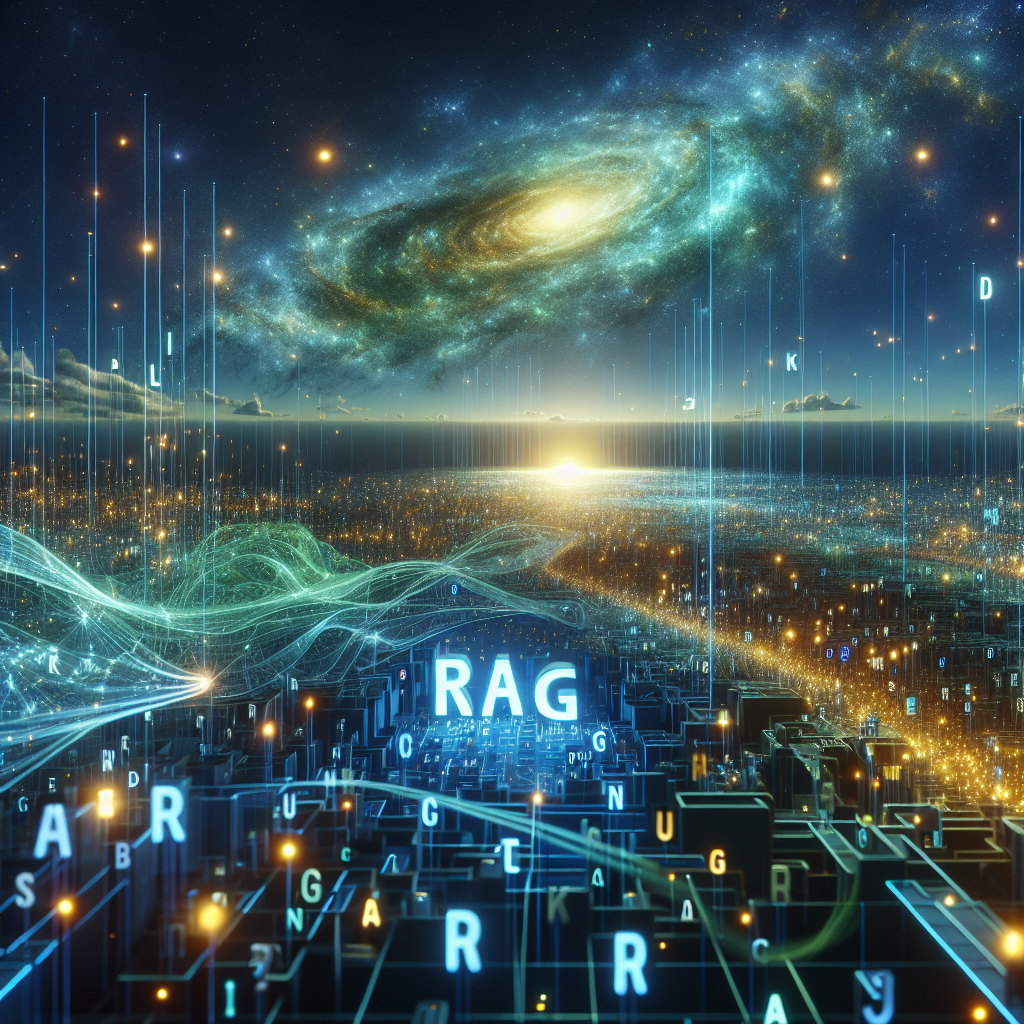
In the rapidly evolving landscape of Natural Language Processing (NLP), Retrieval-Augmented Generation (RAG) has emerged as a groundbreaking advancement, capturing the attention of researchers and developers alike. This innovative approach to language model performance is transforming how AI systems understand, generate, and interact with human language. Contributions from leading institutions like OpenAI, Google Research, and Stanford University have propelled RAG-based AI models to the forefront, enhancing semantic understanding in NLP applications.
In this comprehensive guide, we will explore what Retrieval-Augmented Generation entails, its impact on Natural Language Processing advancements, and how RAG-based AI models can elevate your business. We’ll delve into the mechanics of RAG, examine case studies from leading research entities, highlight practical applications across various industries, and provide insights for integrating these technologies effectively.
Understanding Retrieval-Augmented Generation
What is Retrieval-Augmented Generation?
Retrieval-Augmented Generation (RAG) combines retrieval mechanisms with generative language models to enhance AI systems’ performance in understanding and generating human language. By leveraging external knowledge sources, RAG provides more contextually relevant responses, thus improving the accuracy and adaptability of NLP applications.
How Does RAG Improve Language Model Performance?
The integration of real-time data from external databases allows RAG to access a broader range of information. This dynamic retrieval capability results in more accurate and contextually appropriate responses, significantly enhancing how retrieval-augmented generation improves language model performance. Unlike traditional models that rely on fixed datasets, RAG’s ability to dynamically pull relevant data ensures continuous improvement and adaptation.
The Technical Mechanism Behind RAG
At its core, RAG operates by using two main components: a retriever and a generator. The retriever scans through vast databases or knowledge bases to find information pertinent to the input query. This retrieved context is then fed into the generative model, which crafts responses that are both coherent and contextually aligned with the retrieved data.
This dual-component system allows RAG to mitigate some of the common pitfalls in traditional NLP models, such as generating irrelevant or outdated information. By constantly updating its knowledge base through retrieval processes, RAG maintains a high level of accuracy and relevance.
The Impact of RAG on Enhancing Semantic Understanding
RAG’s ability to incorporate diverse datasets leads to a deeper semantic understanding within NLP applications. By utilizing external data sources, RAG-based AI models can offer more nuanced and precise interpretations of human language, addressing the limitations often found in traditional NLP systems that rely solely on pre-existing knowledge.
Real-World Examples
- Customer Support Automation: In customer service scenarios, RAG enables chatbots to provide solutions based on up-to-date information from company FAQs or support documentation, leading to more efficient and satisfactory user interactions.
- Content Creation and Summarization: For content platforms, RAG can be used to generate summaries of long articles or reports by retrieving key points and generating coherent, concise content.
- Medical Research Assistance: In healthcare, RAG models assist researchers by providing the latest findings from medical journals and studies, ensuring that insights are based on current knowledge.
Leading Research Entities in RAG Development
OpenAI’s Contributions
OpenAI has been instrumental in advancing RAG technology, developing innovative models that push the boundaries of what AI can achieve. Their research highlights how retrieval-augmented generation enhances semantic understanding and overall model performance. Notably, OpenAI’s work on context-awareness has demonstrated significant improvements in conversational agents.
Google Research Innovations
Google Research continues to lead in the field with cutting-edge experiments that showcase the transformative potential of RAG. By integrating these advancements into their products, they have demonstrated significant improvements in NLP applications across various domains. Their work often focuses on scalability and efficiency, ensuring RAG models can handle large-scale data retrieval.
Stanford University’s Role
Stanford University has been a pioneer in exploring the theoretical underpinnings of RAG. Researchers at Stanford focus on optimizing retrieval algorithms to improve speed and accuracy, making RAG more practical for real-world applications. Their studies often involve interdisciplinary approaches, combining insights from computer science, linguistics, and cognitive psychology.
Practical Applications Across Industries
Healthcare
In healthcare, RAG models are revolutionizing how medical information is accessed and utilized. By integrating the latest research findings into diagnostic tools, RAG helps clinicians make informed decisions quickly. For instance, RAG can assist in diagnosing rare diseases by retrieving relevant case studies or treatment protocols.
Finance
The finance sector benefits from RAG through enhanced data analysis capabilities. Financial analysts use RAG to retrieve and analyze market trends, enabling them to provide more accurate forecasts and investment strategies. Additionally, customer service bots powered by RAG can offer personalized financial advice based on real-time data retrieval.
Education
In education, RAG is being used to create intelligent tutoring systems that adapt to individual learning styles. By retrieving educational resources tailored to a student’s needs, these systems provide personalized support, enhancing the learning experience and improving outcomes.
Future Directions for RAG in NLP
As we look to the future, several exciting developments are on the horizon for RAG technology:
- Integration with Other AI Technologies: Combining RAG with other AI advancements, such as reinforcement learning or computer vision, could lead to even more sophisticated applications.
- Improved Personalization: Future iterations of RAG models may offer enhanced personalization capabilities, tailoring responses not only based on context but also individual user preferences and histories.
- Ethical Considerations and Bias Mitigation: As RAG becomes more widespread, addressing ethical concerns and mitigating biases in retrieved data will be crucial to ensure fair and unbiased AI applications.
Conclusion
Retrieval-Augmented Generation represents a significant leap forward in the field of Natural Language Processing. By integrating dynamic retrieval mechanisms with generative models, RAG enhances semantic understanding and improves language model performance across various applications. As leading research entities continue to innovate, the potential for RAG in industries such as healthcare, finance, and education is vast.
Are you ready to leverage the power of Retrieval-Augmented Generation to elevate your business’s NLP capabilities? Our team specializes in AI-driven solutions that integrate cutting-edge technologies like RAG. We have successfully implemented these innovations across various industries, helping businesses enhance customer interactions, streamline content generation, and accelerate research efforts.
By partnering with us, you can unlock new dimensions of efficiency and effectiveness for your organization. Explore our customized AI solutions tailored to meet your unique needs. Visit our website or contact our experts today to learn more about how we can help transform your business with the latest advancements in NLP technology.
We are committed to delivering top-tier AI services that drive success and innovation. Let us empower your business with the transformative potential of Retrieval-Augmented Generation.