Machine Learning for Predictive Maintenance in Government Infrastructure
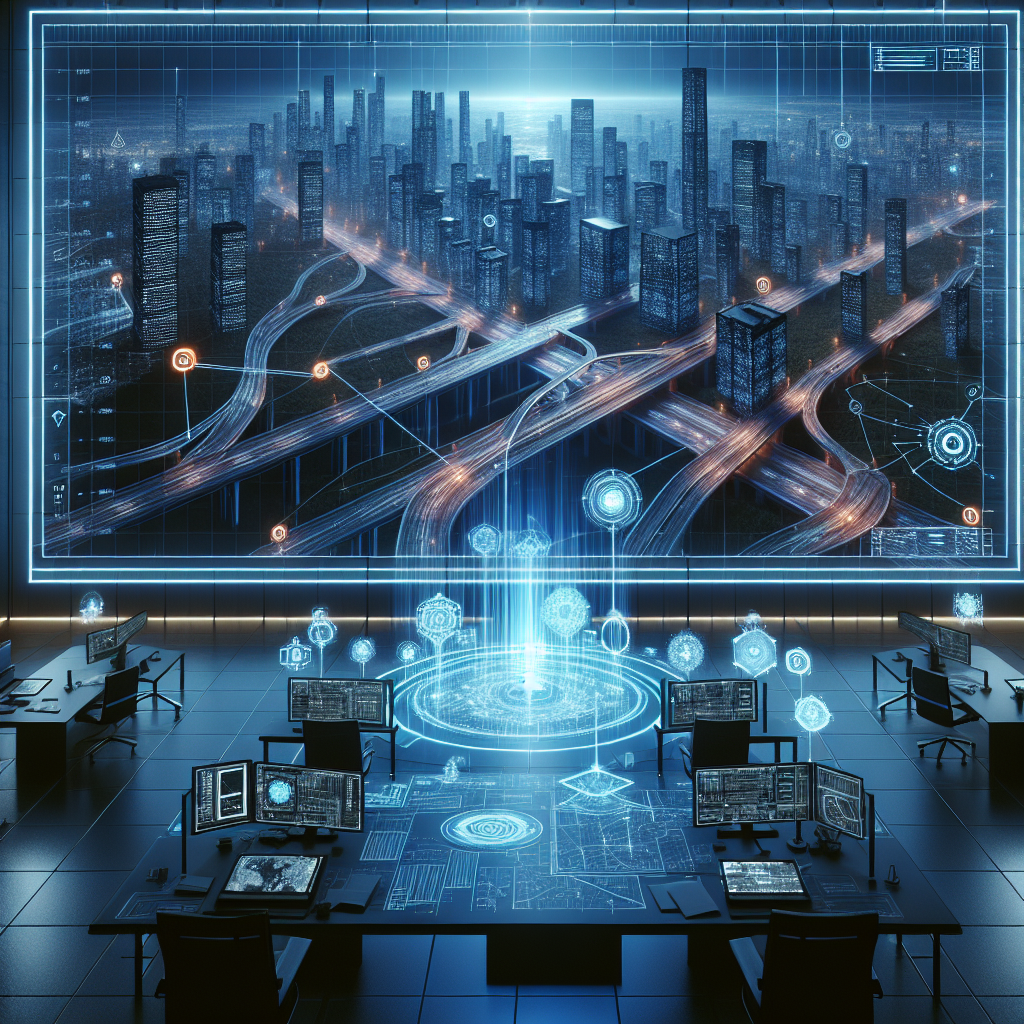
Machine Learning for Predictive Maintenance in Government Infrastructure
In today’s rapidly evolving technological landscape, government infrastructure is experiencing a transformative shift with the integration of machine learning into predictive maintenance strategies. This innovative approach offers immense potential to revolutionize how public assets are managed and preserved. In this blog post, we delve into the significance of leveraging machine learning for predictive maintenance within government infrastructure. We will explore its benefits, challenges, and practical applications, providing valuable insights into why it’s a game-changer in public asset management.
Introduction
Government infrastructure encompasses an extensive array of essential public facilities such as roads, bridges, water systems, energy grids, and public buildings. These assets are fundamental to societal functioning but face deterioration due to factors like age, usage, and environmental conditions. Traditionally, maintenance strategies have relied on fixed schedules or reactive measures—tactics that often lead to unexpected failures and increased costs.
However, applying machine learning to predict and prevent infrastructure maintenance issues is transforming this landscape. By adopting predictive maintenance, government agencies can ensure the longevity and reliability of their assets while optimizing operational efficiency and reducing expenses. This post will illuminate how machine learning-driven predictive maintenance benefits government infrastructure management and examine real-world applications and case studies.
The Evolution of Maintenance Strategies
Traditional Approaches: Scheduled and Reactive Maintenance
Scheduled Maintenance:
- Based on predetermined intervals, often leading to unnecessary repairs or overlooked issues.
- Can result in inefficiencies and higher costs due to maintenance being performed whether needed or not.
Reactive Maintenance:
- Involves addressing problems as they arise, often leading to costly downtime and emergency repairs.
- Results in unpredictable budgeting and potential safety hazards.
The Advantages of Predictive Maintenance
Predictive maintenance is a proactive strategy that utilizes data analysis tools and machine learning algorithms to predict equipment failures before they occur. This approach optimizes the timing of maintenance tasks, ensuring interventions are made precisely when needed, thus enhancing efficiency and reducing costs significantly.
Key Benefits:
- Cost Efficiency: By preventing unexpected breakdowns, predictive maintenance reduces repair expenses and minimizes downtime.
- Extended Asset Lifespan: Early detection and intervention help in prolonging the operational life of infrastructure components.
- Safety Enhancements: Proactive issue identification contributes to safer public spaces by mitigating potential hazards.
Implementing Machine Learning for Predictive Maintenance
Data Collection: The Foundation of Predictive Insights
For predictive maintenance to be effective, accurate data collection is crucial. Sensors and IoT devices embedded in government infrastructure gather vast amounts of real-time data on asset conditions and environmental factors.
Data Sources Include:
- Structural Health Monitoring: Assessing the integrity of buildings and bridges through sensors.
- Environmental Data: Collecting information about weather patterns, temperature, humidity, etc., which can affect infrastructure durability.
- Usage Patterns: Understanding how often and in what manner transportation networks are used to better predict wear and tear.
Machine Learning Algorithms: The Core of Prediction
Machine learning algorithms analyze the collected data to identify patterns that precede failures. These algorithms continuously improve as they process more data, enhancing their predictive accuracy over time.
- Anomaly Detection: Identifying deviations from normal operating conditions.
- Predictive Analytics: Forecasting future states based on historical and real-time data.
Key Advantages in Transportation Networks
Enhancing Reliability
Minimized Downtime:
- Predictive maintenance schedules interventions before failures occur, ensuring transportation systems remain operational without unexpected interruptions.
- This reliability is crucial for public transport systems, reducing delays and improving commuter satisfaction.
Optimized Resource Allocation:
- Resources such as personnel and materials are deployed more efficiently, focusing on areas with the highest risk of failure.
- This targeted approach prevents wastage and ensures that critical components receive timely attention.
Improving Safety
Early Detection of Safety Hazards:
- Machine learning models can identify potential safety issues before they become hazardous, such as structural weaknesses in bridges or rail tracks.
- Early interventions prevent accidents, ensuring the safety of passengers and operators.
Consistent Monitoring:
- Continuous monitoring allows for real-time detection of anomalies that could lead to failures, providing a proactive approach to maintenance.
- This constant vigilance ensures that even minor issues are addressed before they escalate into major problems.
Case Study: The Benefits in Urban Transit Systems
The European Union’s project on smart cities showcases the application of machine learning in urban transit systems. By implementing predictive maintenance strategies, several cities reported a 30% reduction in service disruptions and a significant improvement in passenger safety due to early identification of potential failures.
- Example: In Berlin, predictive analytics have been used to monitor tram lines, resulting in fewer unexpected breakdowns and improved scheduling accuracy.
Challenges in Implementing Machine Learning for Predictive Maintenance
Despite its clear advantages, implementing machine learning-driven predictive maintenance comes with challenges that need addressing:
Data Quality and Availability:
- High-quality data is essential for accurate predictions.
- Ensuring comprehensive and reliable data collection can be challenging.
Investment in Technology:
- Significant upfront costs are required to implement necessary infrastructure and software solutions.
- Balancing budget constraints with the need for advanced technology remains a hurdle.
Skillset Development:
- A skilled workforce is crucial to manage and interpret machine learning systems effectively.
- Ongoing training and education programs are needed to bridge skill gaps.
Change Management:
- Resistance to change within organizations can impede adoption.
- Strategies for smooth transitions must be developed and communicated clearly.
Conclusion
Machine learning-driven predictive maintenance represents a transformative opportunity for government infrastructure management, especially in transportation networks. By embracing this innovative approach, agencies can significantly enhance asset longevity, operational efficiency, and safety standards while reducing costs. As technology continues to advance, the potential for machine learning in infrastructure management will only grow, providing even greater opportunities for optimization and sustainability.
In conclusion, governments worldwide should prioritize investing in predictive maintenance technologies and address associated challenges proactively. By doing so, they can ensure a more resilient and efficient infrastructure landscape that benefits communities for generations to come. This strategic approach not only bolsters the reliability of urban transit systems but also underscores a commitment to public safety and environmental stewardship. As cities continue to grow and evolve, leveraging machine learning in predictive maintenance will be pivotal in meeting the demands of modern transportation networks.