Mastering AI Governance with Cognitive Frameworks
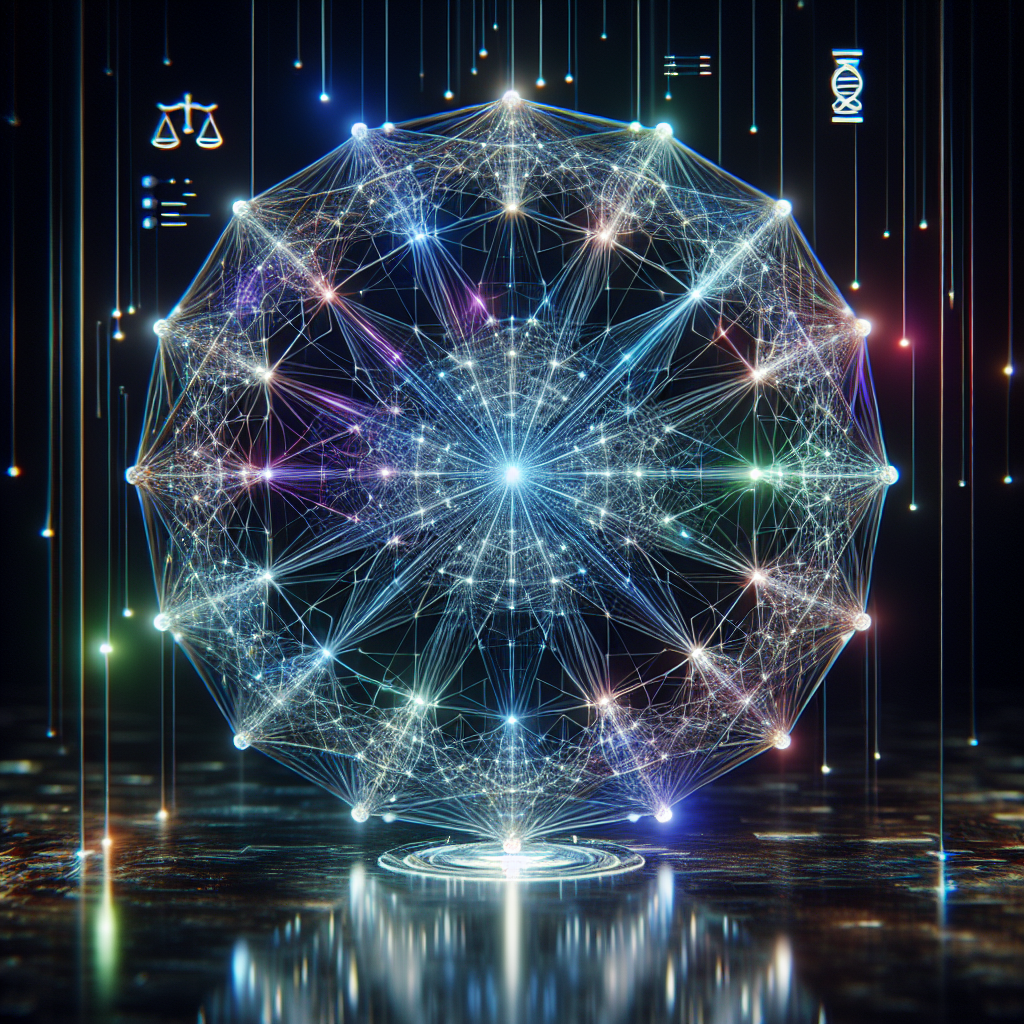
In today’s rapidly evolving landscape, artificial intelligence (AI) is transforming industries by enhancing data analysis, decision-making, and automation capabilities. However, this power comes with the responsibility of managing these technologies ethically and effectively. This blog post delves into how cognitive framework applications can revolutionize AI governance strategies, drawing on insights from leaders like OpenAI, Stanford University, and initiatives by the International AI Ethics Board.
Introduction
Artificial Intelligence is reshaping industries worldwide, offering unparalleled capabilities that drive innovation and efficiency. Yet, with great power comes significant responsibility. Organizations must implement robust AI governance frameworks to mitigate risks, ensure compliance, and uphold ethical standards. This post explores how cognitive frameworks can enhance AI management strategies, fostering sustainable growth and innovation.
The Importance of AI Governance
Defining AI Governance
AI governance involves policies, processes, and guidelines that oversee the development and deployment of AI systems. It ensures these technologies align with organizational values and societal norms. Implementing effective AI governance frameworks enhances organizational accountability, promoting transparency, responsibility, and ethical management—key elements in building trust among stakeholders.
- Enhancing Organizational Accountability: Robust AI governance frameworks help organizations maintain control over their AI initiatives, ensuring compliance with regulatory requirements and ethical standards.
- Ethical AI Management: Governance strategies guide the ethical use of AI by addressing critical issues like bias, privacy, and transparency, fostering responsible innovation. This not only safeguards stakeholders’ interests but also enhances public trust in AI technologies.
Cognitive Frameworks in AI Governance
Understanding Cognitive Biases
Understanding cognitive biases is crucial for effective AI oversight. These biases can significantly influence decision-making processes within AI systems. Recognizing these influences allows for more effective governance:
- Bias Detection: Cognitive frameworks assist in identifying potential biases in AI algorithms by analyzing patterns and outcomes. This includes recognizing data-driven biases that may disproportionately affect certain demographic groups.
- Mitigation Strategies: Once detected, strategies can be implemented to mitigate these biases, ensuring fair and unbiased operations. Techniques such as algorithmic auditing and diverse dataset inclusion are crucial here.
Applying Cognitive Frameworks
Cognitive framework applications provide a structured approach to understanding human interactions with AI systems. They offer insights into human cognition that are invaluable for designing intuitive and ethical AI solutions:
- Human-AI Interaction: Enhancing synergy between humans and AI through cognitive frameworks leads to improved decision-making processes, making technology more accessible and user-friendly.
- AI System Design: These frameworks guide the development of user-friendly, ethical AI applications aligned with human values. For instance, they inform interface design and feedback mechanisms that are critical in enhancing user experience and trust.
- Cognitive Load Management: By understanding how users process information, cognitive frameworks help reduce cognitive load, making complex AI systems easier to understand and use.
Case Studies in Ethical AI Management
OpenAI: Leading by Example
OpenAI is at the forefront of developing AI technologies that benefit humanity while ensuring safety and alignment with human values. Through regular bias audits and active stakeholder engagement, OpenAI exemplifies transparency and accountability in AI development. Their commitment to ethical AI management sets a benchmark for others in the industry.
- Practical Application: OpenAI’s GPT models are continuously improved through feedback loops that incorporate ethical considerations, ensuring alignment with societal norms.
Stanford University: Pioneering Research
Stanford University plays a pivotal role in advancing research on cognitive frameworks and their application in AI governance. The institution’s innovative studies provide valuable insights into understanding and mitigating biases and designing systems that respect user autonomy.
- Research Initiatives: Projects like the Ethics and Governance of Artificial Intelligence (EGAI) program at Stanford explore how to incorporate ethical principles in AI design, contributing significantly to the field.
International AI Ethics Board: Global Standards
The International AI Ethics Board works on establishing global standards for AI governance. Their guidelines emphasize transparency, accountability, and inclusivity in AI systems development, advocating for policies that protect user rights and promote fairness.
- Global Impact: Through initiatives like the AI for Good Summit, they bring together stakeholders from diverse sectors to foster dialogue and collaboration on ethical AI issues.
Challenges and Solutions in AI Governance
While implementing AI governance frameworks enhances organizational accountability, several challenges exist:
- Regulatory Compliance: Keeping up with evolving regulations across different jurisdictions can be complex.
- Solution: Establishing a dedicated compliance team that continuously monitors regulatory changes is essential.
- Data Privacy Concerns: Protecting user data while leveraging it for AI development is critical.
- Solution: Implementing robust data anonymization techniques and ensuring transparency in data usage policies are vital steps.
- Ethical Dilemmas: Balancing innovation with ethical considerations can be challenging.
- Solution: Creating an ethics board within organizations to oversee AI projects ensures that ethical guidelines are integrated into every stage of development.
Future Directions
As AI continues to evolve, future advancements in governance may include:
- AI Explainability: Enhancing the transparency of AI decision-making processes will help users understand how decisions are made.
- Collaborative Governance Models: Encouraging collaboration between governments, private sectors, and academia can lead to more comprehensive governance frameworks.
- Continuous Learning Systems: Developing AI systems that continuously learn from new data and user interactions will improve adaptability and relevance over time.
Conclusion
Mastering AI governance with cognitive frameworks is crucial for fostering ethical AI development. By understanding cognitive biases and applying structured approaches, organizations can ensure their AI systems are transparent, accountable, and aligned with human values. As leaders like OpenAI, Stanford University, and the International AI Ethics Board demonstrate, a commitment to ethical practices in AI can lead to more trustworthy and impactful technologies.