Model Alignment in Generative AI Projects
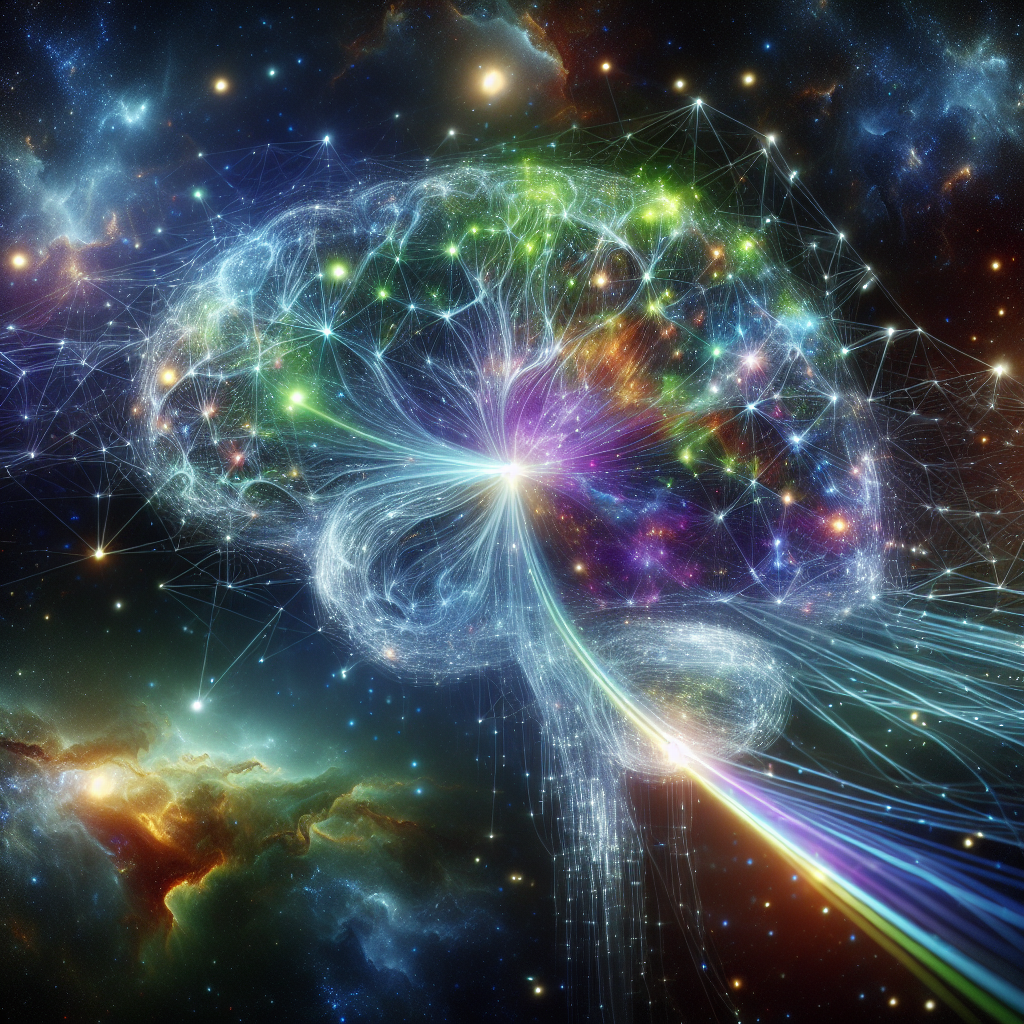
In recent years, generative AI has captivated both industry leaders and the public with its transformative potential across various sectors. However, as these technologies advance, ensuring they align with human values and organizational goals becomes paramount for successful deployment. This blog post explores essential strategies, ethical considerations, and best practices in generative AI model alignment.
Introduction
Generative AI models have become a cornerstone of innovation, enabling applications from creative content generation to data-driven decision-making processes. Despite their potential, these models can pose significant risks if misaligned with human values or organizational objectives. Model alignment ensures that AI systems behave in ways beneficial and consistent with intended purposes. This article delves into techniques for achieving model alignment, discusses its ethical implications, and highlights the importance of ensuring that generative AI models align with human values and organizational goals.
The Importance of Generative AI Model Alignment
Generative AI models, such as those developed by OpenAI, have shown remarkable capabilities in producing realistic text, images, and even music. However, their success hinges on how well they align with human values and organizational goals. Misalignment can lead to unintended consequences, from biased outputs to security vulnerabilities.
Why Model Alignment Matters
- Ethical Considerations: Ensuring AI models adhere to ethical standards is crucial for maintaining public trust and avoiding harm.
- Organizational Goals: Aligning AI with business objectives ensures that projects deliver value and support strategic initiatives.
- Regulatory Compliance: Adhering to frameworks, like those proposed by the European Union, helps avoid legal pitfalls.
Real-world Examples
Consider the case of generative AI in content creation. Platforms using models like GPT-3 have faced challenges with generating biased or inappropriate content if not properly aligned with ethical standards. By implementing robust alignment strategies, these platforms can enhance user trust and compliance with regulations, such as those governing hate speech.
Another example is the use of generative AI in healthcare for creating patient-specific treatment plans. Without proper alignment, AI systems might recommend ineffective treatments due to biased training data. Ensuring model alignment with clinical guidelines and ethical standards can prevent such risks, improving patient outcomes and trust in medical technology.
Techniques for Achieving Model Alignment
Achieving generative AI model alignment involves various strategies, from training data selection to continuous monitoring. Here are some effective techniques:
1. Define Clear Objectives
Establish clear objectives that align with both human values and organizational goals. This initial step sets a foundation for the AI project’s development.
Case Study: Google’s AI Principles
Google has outlined principles guiding its AI research and application, emphasizing alignment with user needs and societal benefits. By setting explicit objectives, Google ensures that its generative models serve diverse populations without exacerbating inequalities.
2. Diverse Training Data
Utilize diverse datasets to minimize bias and ensure the model can generalize across different scenarios. Institutions like Stanford University emphasize the importance of data diversity in reducing systemic biases.
Example: Facial Recognition Bias Mitigation
In facial recognition systems, leveraging diverse training datasets has been crucial in minimizing racial and gender biases. By including a wide range of demographic groups in training data, models become more equitable and aligned with ethical standards.
3. Regular Evaluation
Implement techniques for evaluating the performance of AI models against specified objectives. This involves setting up benchmarks and continuously monitoring outcomes to ensure alignment is maintained throughout the model’s lifecycle. Techniques for evaluating the performance of AI models can significantly improve overall project outcomes.
Tools and Frameworks
Frameworks like IBM’s AI Fairness 360 provide tools to assess and mitigate bias in machine learning models. Regular evaluation using such frameworks ensures ongoing alignment with ethical standards and organizational goals.
4. Human-in-the-Loop Systems
Incorporate human oversight into AI systems to provide context-sensitive evaluations and adjustments. This approach helps catch issues that automated processes might miss.
Example: Content Moderation
Platforms like Facebook use human-in-the-loop systems for content moderation, where humans review AI-generated decisions to ensure they align with community standards and ethical guidelines.
Ethical Considerations in AI Project Development
Ethics play a critical role in generative AI development. Addressing ethical considerations can prevent potential pitfalls and ensure responsible innovation.
- Transparency: Ensure transparency in how models are developed and deployed.
- Accountability: Establish clear accountability mechanisms for decisions made by AI systems.
- Fairness: Implement measures to detect and mitigate biases within AI models.
The Role of Transparency
Transparent practices allow stakeholders to understand how generative AI models function, fostering trust. For instance, OpenAI’s move towards more transparent model architectures helps demystify AI decision-making processes for users and regulators alike.
Building Accountability
Creating mechanisms for accountability in AI systems ensures that developers and organizations can be held responsible for the outcomes of their technologies. This might include maintaining detailed logs of decision-making processes or implementing audit trails for AI-generated content.
AI Project Development Strategies
Strategic planning is essential for successful generative AI projects. Here are some strategies to consider:
1. Cross-disciplinary Collaboration
Involve experts from diverse fields—such as ethicists, legal professionals, and domain specialists—to ensure comprehensive alignment of AI models with ethical and regulatory standards.
Collaborative Frameworks
Creating interdisciplinary teams can lead to more robust AI systems that account for a wide range of perspectives and potential impacts.
2. Continuous Feedback Loops
Implement mechanisms for continuous feedback from users and stakeholders. This helps refine AI systems in response to real-world usage and emerging ethical concerns.
Example: User-Centric Design
Incorporating user feedback into the development cycle, as seen in many tech companies’ agile methodologies, ensures that generative AI models remain aligned with evolving user needs and societal values.
3. Scenario Planning
Conduct scenario planning to anticipate potential challenges and outcomes of deploying generative AI systems. This proactive approach helps mitigate risks and aligns projects with long-term organizational goals.
Risk Management in AI Deployment
By analyzing various deployment scenarios, organizations can better prepare for unexpected consequences, ensuring that their AI systems are both effective and aligned with ethical standards.
Conclusion
Model alignment is essential for the successful deployment of generative AI. By focusing on clear objectives, diverse training data, regular evaluation, and human oversight, developers can ensure these models align with human values and organizational goals. Ethical considerations such as transparency, accountability, and fairness must be integral to AI project development strategies. As generative AI continues to evolve, ongoing attention to alignment will be crucial in harnessing its potential for positive societal impact.