Optimizing Agent Learning for Business Applications
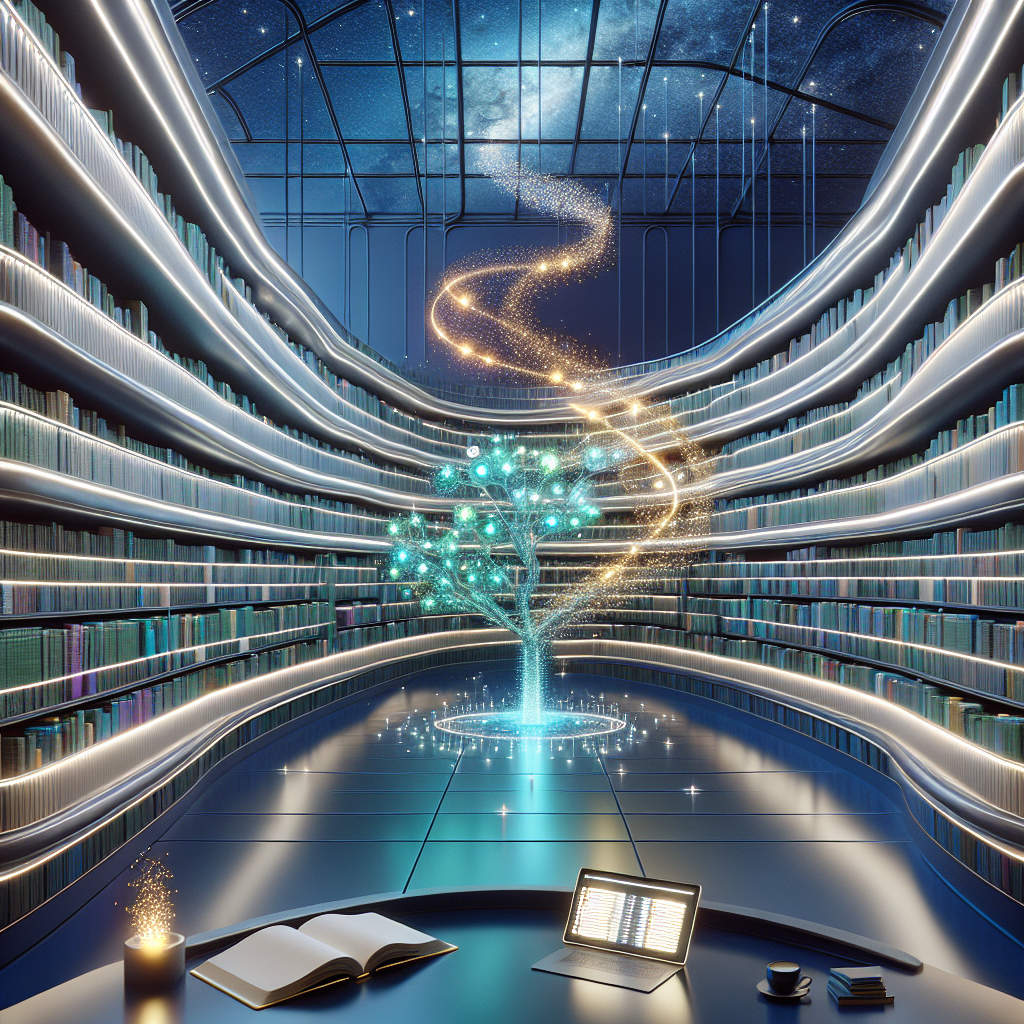
Hey there! If you’re a business professional eager to unlock the full potential of AI-driven solutions, let’s talk about optimizing agent learning. Whether you’re navigating the complexities of finance, healthcare, or any industry where efficiency is crucial, integrating artificial intelligence can revolutionize your organization’s operations. In this guide, we’ll explore why and how enhancing agent learning is vital for business applications, offering actionable insights to elevate your AI capabilities.
Introduction: Why Optimize Agent Learning?
Let’s kick things off with the big picture. Picture an office where decisions are made with pinpoint accuracy, processes run smoother than ever before, and customer satisfaction skyrockets because you’re always a step ahead. Sounds like a dream? It’s actually achievable by optimizing agent learning in your business operations.
In today’s fast-paced market landscape, companies that quickly adapt to changes hold the competitive edge. Implementing machine learning strategies is not just beneficial; it’s essential for staying ahead of the curve. Automated agents can take over tasks from customer service to data analysis, freeing up your team to focus on strategic initiatives. But here’s the catch: to fully reap the benefits of these AI-driven solutions, you need to fine-tune how these agents learn and make decisions.
The Power of Enhanced Efficiency
Optimizing agent learning not only improves efficiency but also boosts innovation within your organization. By automating routine tasks, your team can dedicate more time to creative problem-solving and strategic planning. This shift from operational tasks to value-added activities is a game-changer for any business aiming to enhance productivity.
Real-World Impact
Consider how some of the world’s leading companies have harnessed optimized agent learning. Netflix uses AI-driven algorithms to personalize recommendations, ensuring users discover content they’ll love. In healthcare, AI models predict patient outcomes and assist in early diagnosis, significantly improving treatment plans. These examples highlight the transformative power of well-optimized agent learning.
Prerequisites for Optimizing Agent Learning
Before diving into the nitty-gritty, let’s ensure we’ve got everything in place:
- A Basic Understanding of Machine Learning: Get familiar with how algorithms learn from data. This foundational knowledge will help you make informed decisions about which AI tools and models to implement.
- Access to AI Tools: Explore platforms like OpenAI or IBM Watson that can be game-changers for your business. These platforms offer robust frameworks that cater to diverse industry needs.
- Data Collection Infrastructure: Make sure you can gather and manage large datasets, as they’re crucial for training your agents.
Building the Right Foundation
Before jumping into optimization strategies, it’s essential to have a solid foundation in place. Ensuring data integrity and accessibility is critical since high-quality data forms the backbone of any successful AI initiative. Additionally, understanding basic machine learning concepts allows you to better collaborate with technical teams or external consultants.
Step-by-Step Instructions
1. Define Your Goals Clearly
Start by asking yourself: What specific challenges am I looking to overcome? Is it enhancing customer service response times, automating data entry tasks, or predicting market trends? Having clear objectives will steer the optimization process and help measure success.
Setting SMART Goals
To ensure your goals are actionable, consider setting SMART (Specific, Measurable, Achievable, Relevant, Time-bound) objectives. This approach not only clarifies what you aim to achieve but also provides a framework for evaluating progress and outcomes.
2. Gather and Preprocess Data
Data is the lifeblood of machine learning. Collect relevant datasets—these could be historical transaction records, social media interactions, or customer feedback. Clean your data to remove inconsistencies and ensure it’s in a format that can be fed into your AI models.
Case Study: A Retail Giant’s Approach
A major retail company faced challenges with inventory management due to inconsistent data from various sources. By implementing a comprehensive data preprocessing strategy, they harmonized their datasets, leading to more accurate demand forecasting and reduced stockouts by 30%.
3. Choose the Right Model and Framework
Not all machine learning models are created equal. Depending on your goals, you might opt for supervised learning (where you train the model with labeled data) or unsupervised learning (for finding hidden patterns). Tools like OpenAI’s GPT-3 or IBM Watson offer versatile frameworks to kickstart this process.
Matching Models to Business Needs
When selecting a model, consider factors such as complexity, scalability, and interpretability. For instance, if you need quick insights from large datasets, ensemble methods might be ideal due to their robustness and accuracy.
4. Train and Test Your Agents
Once you’ve selected your models, it’s time for training. Feed your preprocessed data into the system and let it learn. After training, test the model on a separate dataset to evaluate its accuracy and effectiveness. Iterate as needed to refine performance.
Leveraging Real-Time Feedback
Consider using real-time feedback loops during testing phases. This approach allows you to make immediate adjustments based on how well your agents perform in live environments, enhancing their learning process.
5. Implement Feedback Loops
Continuous improvement is key. Set up mechanisms where your agents receive feedback based on their actions. This helps them adjust and improve over time. Incorporating human-in-the-loop systems can also enhance decision-making accuracy.
Industry Example: Financial Services
In the financial sector, companies implement feedback loops to refine fraud detection algorithms continually. By analyzing flagged transactions with expert oversight, these AI models adapt to new fraud patterns more effectively.
6. Monitor Performance Regularly
After deployment, keep an eye on how well the AI solutions are performing. Use analytics tools to track metrics like task completion rates or customer satisfaction scores. This data will be invaluable for ongoing optimization.
Predictive Maintenance Insights
For industries reliant on machinery, predictive maintenance models that continuously monitor equipment performance can prevent costly downtimes and extend asset lifespan through proactive interventions.
Common Mistakes to Avoid
- Underestimating Data Quality: Poor data can lead to inaccurate results. Prioritize data integrity from the outset.
- Ignoring Model Bias: Ensure your models don’t perpetuate existing biases in the data, as this could harm decision-making processes.
- Lack of Continuous Monitoring: AI solutions require regular updates and adjustments to remain effective.
- Overlooking Security Concerns: Protect sensitive data by implementing robust security measures.
Advanced Tips for Experts
- Experiment with Transfer Learning: This involves using pre-trained models to save time and resources while achieving high accuracy, especially in domains where labeled data is scarce.
- Explore Reinforcement Learning: For tasks that involve sequential decision-making, reinforcement learning can be particularly effective. This approach allows agents to learn optimal actions through trial-and-error interactions with their environment.
- Stay Updated on AI Trends: The field evolves rapidly; keeping abreast of the latest research and developments can provide a competitive edge.
Future Predictions
As AI technology continues to advance, we anticipate more sophisticated agent learning models that are capable of handling increasingly complex tasks with minimal human intervention. This evolution will likely lead to further automation in areas such as customer service, supply chain management, and personalized marketing strategies.
Frequently Asked Questions
What is agent learning in AI?
Agent learning refers to the process where AI systems learn from interactions with their environment to make decisions or predictions. It’s an integral part of building smart, autonomous agents for business applications.
How do OpenAI and IBM Watson differ in optimizing agent learning?
OpenAI focuses on advanced language models like GPT-3, ideal for natural language processing tasks. IBM Watson offers a broader suite of AI tools, including cognitive computing capabilities suited for various industries.
Is data security a concern with AI-driven solutions?
Absolutely. As you integrate AI into your business, ensure robust encryption and access controls to protect sensitive information from breaches.
Ready to Transform Your Business with AI?
We understand that integrating AI-driven solutions can seem daunting at first. But with the right approach, it can be a game-changer for your business operations. We’ve helped numerous companies across different industries—be it enhancing customer experiences or optimizing supply chain processes—implement robust AI agent learning systems.
Interested in exploring how these strategies can revolutionize your business? Reach out to us through our contact form on this page for a no-obligation consultation. We’re here to guide you every step of the way, ensuring that you harness the full potential of AI solutions tailored to your specific needs.
If you have any questions or need further assistance, don’t hesitate to get in touch—we’d be more than happy to help!