Scalable AI Solutions for Data Science Teams
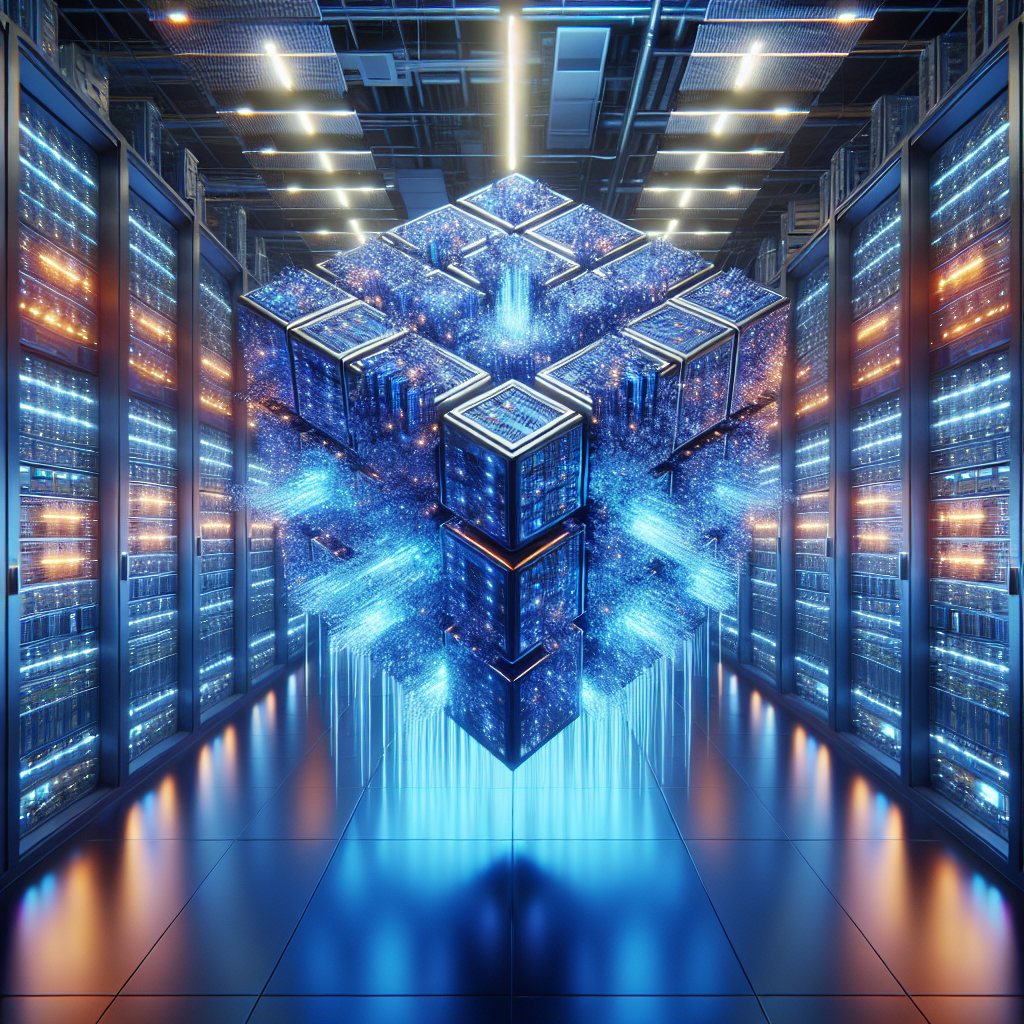
Scalable AI Solutions for Data Science Teams: Unlocking Efficiency and Innovation
As the field of data science continues to evolve, Data Science Teams are facing increasing pressure to deliver insights and models that drive business value. To meet this demand, teams are turning to Scalable AI Solutions that can handle large volumes of data, complex algorithms, and rapid deployment. In this blog post, we will delve into the world of scalable AI solutions and their impact on data science teams, exploring the importance of scalability in AI solutions and how they can transform the way teams work.
Introduction to Scalable AI Solutions
Scalable AI solutions are designed to help data science teams overcome the challenges of working with large datasets, complex models, and tight deadlines. These solutions provide a range of benefits, including faster processing times, improved model accuracy, and increased collaboration. By leveraging scalable AI solutions, data science teams can focus on higher-level tasks, such as strategy and innovation, rather than getting bogged down in technical details. This is particularly important when exploring scalable AI solutions for data science teams, as it enables them to unlock efficiency and drive business growth.
Benefits of Scalable AI Solutions for Data Science Teams
The benefits of scalable AI solutions for data science teams are numerous. Some of the key advantages include:
- Improved scalability: Scalable AI solutions can handle large volumes of data and complex algorithms with ease, making them ideal for big data projects that utilize frameworks like Hadoop.
- Increased efficiency: By automating routine tasks and providing real-time insights, scalable AI solutions can help data science teams work more efficiently and effectively, allowing them to focus on understanding the importance of scalability in AI solutions.
- Enhanced collaboration: Scalable AI solutions often provide tools and features that facilitate collaboration and communication among team members, stakeholders, and external partners, which is critical when implementing scalable AI solutions.
- Faster time-to-market: With scalable AI solutions, data science teams can deploy models and insights faster, giving their organizations a competitive edge in the market and enabling them to respond quickly to changing market conditions.
Key Components of Scalable AI Solutions
So, what makes up a scalable AI solution? Some of the key components include:
- Cloud-based infrastructure: Cloud-based infrastructure provides the scalability and flexibility that data science teams need to handle large datasets and complex models, making it an essential component of scalable AI solutions.
- Distributed computing: Distributed computing allows data science teams to process large datasets in parallel, reducing processing times and improving model accuracy, which is critical for big data projects.
- Automated machine learning: Automated machine learning (AutoML) tools provide a range of benefits, including faster model development, improved model accuracy, and reduced manual effort, making them an essential component of scalable AI solutions.
- Real-time analytics: Real-time analytics enable data science teams to analyze and respond to changing market conditions in real-time, making it possible to stay ahead of the competition.
Implementing Scalable AI Solutions
Implementing scalable AI solutions requires careful planning and execution. Here are some steps that data science teams can follow:
- Define project goals and objectives: Clearly define the goals and objectives of the project, including the types of insights and models that need to be developed.
- Choose the right tools and technologies: Choose the right tools and technologies for the project, including cloud-based infrastructure, distributed computing, and automated machine learning.
- Develop a robust data strategy: Develop a robust data strategy that includes data collection, storage, processing, and analysis.
- Build a skilled team: Build a skilled team with expertise in data science, machine learning, and software development.
Overcoming Challenges and Limitations
While scalable AI solutions offer many benefits, there are also challenges and limitations to be aware of. Some of the common challenges and limitations include:
- Data quality issues: Poor data quality can negatively impact model accuracy and reliability.
- Model interpretability: Complex models can be difficult to interpret, making it challenging to understand their decisions and actions.
- Security and governance: Scalable AI solutions require robust security and governance controls to protect sensitive data.
Future Directions
The field of scalable AI solutions is rapidly evolving, with new technologies and techniques emerging all the time. Some potential future directions include:
- Increased use of edge computing: Edge computing enables data science teams to analyze and respond to changing market conditions in real-time, reducing latency and improving model accuracy.
- Greater emphasis on explainability: As models become more complex, there is a growing need for techniques that can explain their decisions and actions, making them more transparent and trustworthy.
- More focus on human-AI collaboration: Scalable AI solutions will increasingly involve collaboration between humans and machines, enabling data science teams to work more efficiently and effectively.
Case Studies
Several organizations have successfully implemented scalable AI solutions to drive business growth and innovation. Here are a few examples:
- Netflix: Netflix uses scalable AI solutions to personalize recommendations for its users, improving engagement and reducing churn.
- Amazon: Amazon uses scalable AI solutions to optimize supply chain operations, improving efficiency and reducing costs.
- Google: Google uses scalable AI solutions to improve search results, making it easier for users to find relevant information.
Conclusion
Scalable AI solutions offer a range of benefits for data science teams, from improved scalability and increased efficiency to enhanced collaboration and faster time-to-market. By understanding the importance of scalability in AI solutions and exploring scalable AI solutions for data science teams, organizations can unlock efficiency and drive business growth. While there are challenges and limitations to be aware of, the potential benefits of scalable AI solutions make them an essential component of any data science strategy.
Frequently Asked Questions
Q1: What are the key components of a scalable AI solution?
A1: The key components of a scalable AI solution include cloud-based infrastructure, distributed computing, automated machine learning, and real-time analytics.
Q2: How can I get started with implementing scalable AI solutions for my data science team?
A2: To get started, identify your team’s needs and goals, and then develop a roadmap that outlines the key milestones, timelines, and resource allocations for the project.
Q3: What are some common challenges and limitations of scalable AI solutions?
A3: Common challenges and limitations include data quality issues, model interpretability problems, and security and governance concerns.
Q4: How can I ensure that my scalable AI solution is secure and compliant with regulations?
A4: To ensure security and compliance, design your solution with security and governance in mind, and implement robust controls and monitoring mechanisms to protect sensitive data.
Q5: What are some potential future directions for scalable AI solutions?
A5: Potential future directions include increased use of edge computing, greater emphasis on explainability, and more focus on human-AI collaboration.
By following these guidelines and best practices, data science teams can unlock the full potential of scalable AI solutions and drive business growth and innovation. Whether you’re just starting out or looking to improve existing processes, this guide provides a comprehensive overview of the key components, benefits, and challenges of scalable AI solutions.