The Future of Data Science: Embracing Generative AI Models
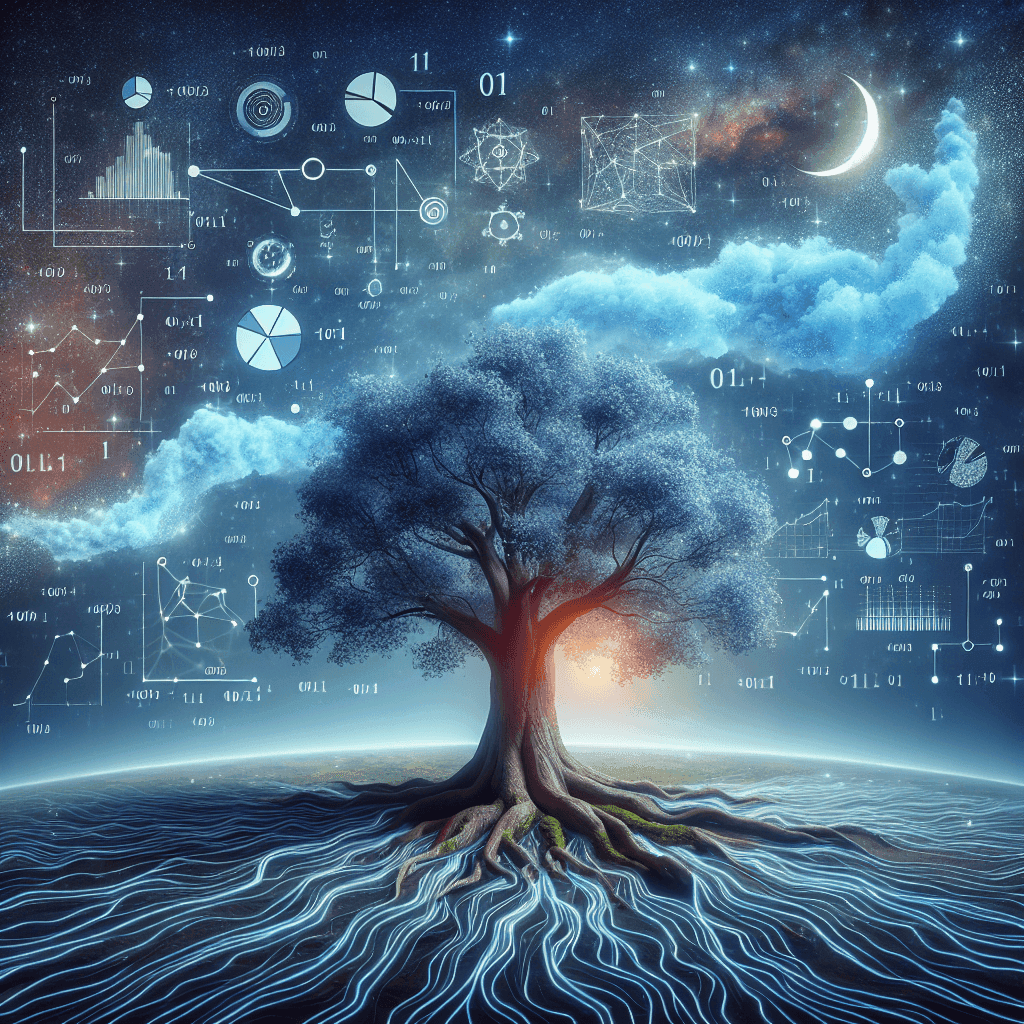
The Future of Data Science: Embracing Generative AI Models
Data science is a rapidly evolving field, with new advancements and technologies emerging every year. One of the most exciting developments in recent years has been the rise of Generative AI models. These models have the potential to revolutionize various aspects of data science, from predictive modeling to data generation. In this blog post, we will explore the future of data science and how Generative AI models are set to shape its course.
The Current State of Data Science
Before we dive into the future of data science, let’s take a look at where we stand today. Data science has become an essential tool for businesses, organizations, and individuals alike. It enables us to extract insights from large datasets, make predictions, and inform decision-making. However, traditional data science methods have limitations. They often rely on manual processing, require significant expertise, and can be time-consuming.
The Limitations of Traditional Data Science Methods
- Scalability: Traditional data science methods are often limited by their ability to scale. As datasets grow in size, it becomes increasingly difficult to process them manually.
- Expertise: Data science requires specialized skills and expertise. This can be a barrier for individuals who want to apply data science techniques but lack the necessary experience.
- Time-consuming: Traditional data science methods often involve manual processing, which can be time-consuming.
The Rise of Generative AI Models
Generative AI models are changing the game for data science. These models enable us to generate new data, predict outcomes, and identify patterns in complex datasets. Some of the key benefits of Generative AI models include:
- Scalability: Generative AI models can process large datasets quickly and efficiently.
- Speed: Generative AI models can automate many tasks, freeing up time for more strategic work.
- Insight generation: Generative AI models can generate new insights and identify patterns that traditional data science methods may miss.
Applications of Generative AI Models in Data Science
- Predictive modeling: Generative AI models can be used to build predictive models that can forecast future outcomes. These models are particularly useful for predicting continuous values, such as stock prices or temperatures.
- Data generation: Generative AI models can generate new data, which can be used for training machine learning models or for testing hypotheses. This is especially useful when working with limited or noisy datasets.
- Anomaly detection: Generative AI models can identify anomalies in complex datasets. These models are particularly useful for identifying outliers and unusual patterns.
The Future of Data Science with Generative AI Models
The future of data science is looking bright with the emergence of Generative AI models. These models have the potential to revolutionize various aspects of data science, from predictive modeling to data generation. As these technologies continue to evolve, we can expect to see new applications and innovations emerge.
Use Cases for Generative AI Models
- Generative Adversarial Networks (GANs): GANs are a type of Generative AI model that can generate highly realistic images and videos.
- Variational Autoencoders (VAEs): VAEs are another type of Generative AI model that can compress and decompress data, making it useful for tasks such as dimensionality reduction and feature learning.
- Generative Reinforcement Learning: This involves using Generative AI models to learn complex behaviors in environments, making it useful for applications such as robotics and game playing.
Challenges and Opportunities
- Data quality: Generative AI models require high-quality data to function effectively. Poorly quality data can lead to biased or inaccurate outcomes.
- Explainability: Generative AI models can be difficult to interpret, making it challenging to understand how they arrived at their conclusions. This can make it challenging to trust the results of these models.
- Ethics: Generative AI models raise ethical concerns, such as the potential for biased outcomes or the misuse of data.
Strategies for Overcoming Challenges
- Data curation: To improve the quality of data used in Generative AI models, organizations can invest time and resources into data curation.
- Model interpretability techniques: Researchers are actively working on developing new model interpretability techniques to help explain the conclusions reached by Generative AI models.
- Regulatory frameworks: As Generative AI models become more widespread, regulatory frameworks will need to be put in place to ensure that these technologies are used responsibly.
The Benefits of Generative AI Models
- Increased efficiency: Generative AI models can automate many tasks, freeing up time for more strategic work.
- Improved accuracy: Generative AI models can improve the accuracy of predictions and outcomes by identifying patterns and relationships in complex datasets.
- New insights: Generative AI models can generate new insights and identify patterns that traditional data science methods may miss.
How to Get Started with Generative AI Models
- Choose a programming language: To work with Generative AI models, you will need to choose a programming language such as Python or R.
- Select a library or framework: There are many libraries and frameworks available for working with Generative AI models, including TensorFlow, PyTorch, and Keras.
- Practice and experiment: The best way to learn about Generative AI models is through practice and experimentation. Start by building small projects and gradually work your way up to more complex applications.
Future Trends in Data Science
- Edge AI: As data becomes increasingly ubiquitous, the need for Edge AI will grow. This involves using Generative AI models at the edge of the network, reducing latency and improving performance.
- Explainability: As Generative AI models become more widespread, there will be a growing need for model interpretability techniques to help explain their conclusions.
- Autonomous data science: Autonomous data science involves using Generative AI models to automatically analyze and improve datasets, making it an exciting area of research and development.
By understanding the current state of data science, the limitations of traditional methods, and the benefits of Generative AI models, we can begin to envision a future where these technologies are used to drive business innovation and success.