The Impact of AI on Environmental Data Analysis
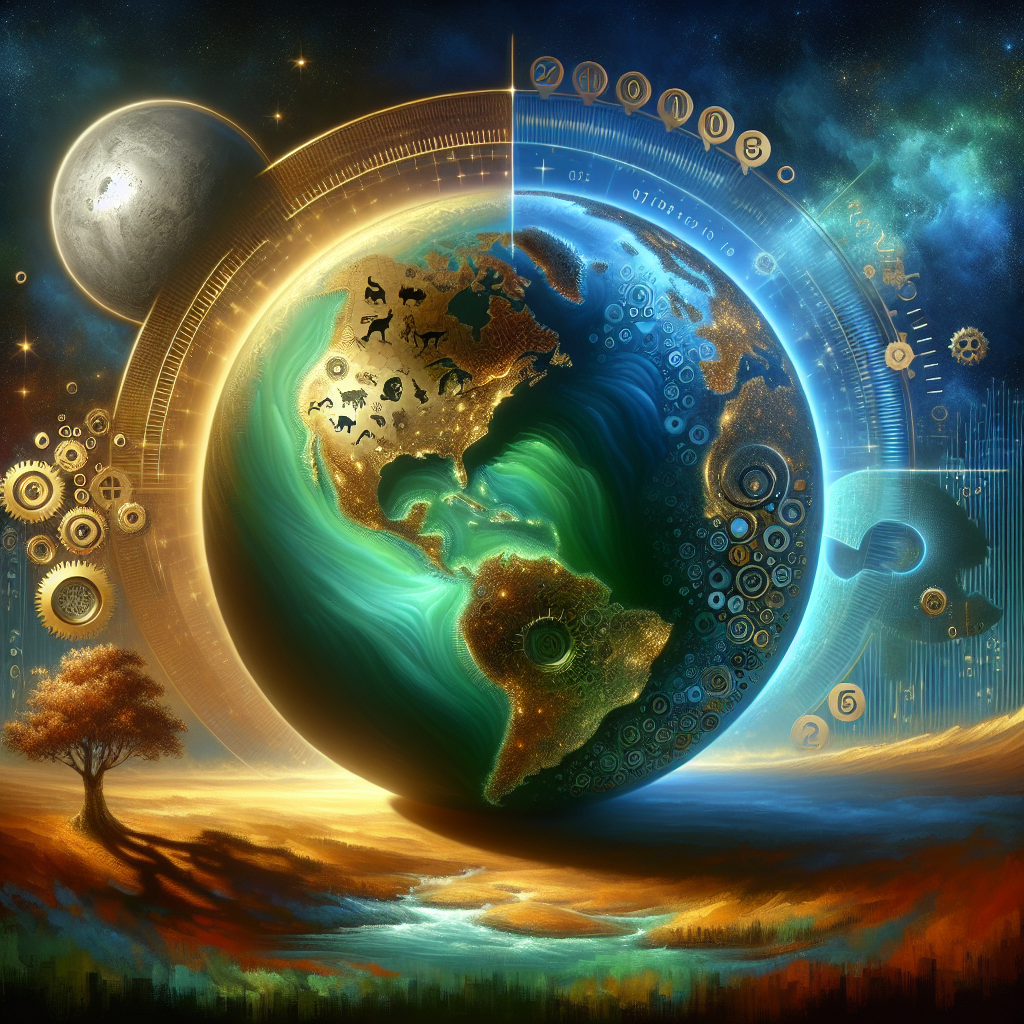
The Impact of AI on Environmental Data Analysis
Introduction
In today’s world, where addressing environmental challenges is more critical than ever, leveraging cutting-edge technologies like Artificial Intelligence (AI) has become essential. AI in environmental data analysis is revolutionizing how we approach sustainability and tackle pressing issues such as climate change. This blog post explores the profound impact AI is having on environmental data analysis, offering sustainability insights and enhancing our capabilities for climate change modeling.
The Role of AI in Environmental Data Analysis
Enhancing Data Processing Capabilities
The enormous volume of environmental data generated daily—from satellite imagery provided by agencies like NASA Earth Observatory to sensor readings—presents a significant challenge. Here, AI excels at processing massive datasets efficiently, extracting meaningful patterns that would be impossible for humans to discern manually. Machine learning algorithms can analyze and interpret vast amounts of information in real time, providing actionable insights swiftly.
Accuracy and Predictive Analytics
AI enhances the accuracy of environmental models by continuously refining predictions based on new data inputs. Through techniques like deep learning, AI systems learn from historical data, improving their predictive capabilities over time. This is especially vital for predicting weather patterns and assessing climate change impacts, where precision can significantly influence decision-making processes. Predictive analytics using AI can aid in the development of sustainable practices and policies.
Automation in Data Collection
Automation powered by AI technologies has revolutionized environmental data collection methods. Drones equipped with AI sensors can monitor deforestation or track wildlife movements, while automated weather stations gather atmospheric data continuously. This not only saves time and resources but also increases the frequency and reliability of data collection.
Sustainability Insights through AI
Resource Management
AI provides sustainability insights by optimizing resource management practices across various sectors. For instance, smart agriculture systems use AI to analyze soil health and weather patterns, enabling farmers to maximize crop yields while minimizing water and fertilizer usage. Similarly, AI-driven energy grids can dynamically balance supply and demand, reducing waste and enhancing efficiency.
Pollution Control
AI plays a critical role in monitoring and controlling pollution levels. Advanced algorithms process data from air quality sensors to predict pollution spikes and suggest mitigation strategies. Furthermore, AI systems optimize industrial processes to reduce emissions, contributing significantly to cleaner air and water—a mission supported by organizations like Greenpeace.
Wildlife Conservation
Conservation efforts benefit immensely from AI’s ability to analyze large datasets related to wildlife populations and habitats. AI-powered models can predict the impact of environmental changes on species distribution, aiding conservationists in developing targeted strategies to protect endangered animals, as highlighted by initiatives documented by the National Geographic Society.
Climate Change Modeling with AI
Enhanced Predictive Models
AI enhances climate change modeling by improving the accuracy and granularity of predictive models. These models consider variables from global data sources like NASA Earth Observatory, helping policymakers understand potential future scenarios better. By processing large datasets to identify trends and solutions for environmental challenges, AI supports robust climate change mitigation strategies.
Scenario Analysis
AI’s ability to perform scenario analysis is invaluable in climate change modeling. This capability allows researchers to explore various “what-if” situations, providing insights into the potential outcomes of different policy decisions and their impacts on the environment.
Real-Time Monitoring
AI facilitates real-time monitoring of environmental parameters, crucial for timely responses to climate-related events. By analyzing patterns that precede natural disasters, AI can help predict such occurrences, enabling authorities to allocate resources effectively and potentially save lives.
Case Studies: AI in Action
AI and Deforestation
In the Amazon rainforest, AI technologies are being used to combat illegal logging activities. Satellite imagery combined with machine learning models identifies areas where deforestation might be occurring. Organizations like Greenpeace have partnered with technology firms to deploy these systems, enabling quicker responses to protect endangered ecosystems.
Predictive Modeling for Hurricanes
AI is transforming how meteorologists predict hurricanes by analyzing vast datasets from ocean buoys, satellites, and weather stations. AI algorithms detect subtle changes in weather patterns that might indicate the formation of a hurricane, allowing for earlier warnings and better-prepared communities. The National Geographic Society has highlighted such initiatives as crucial advancements in disaster management.
Urban Heat Islands
Cities often experience elevated temperatures due to human activities—a phenomenon known as urban heat islands. AI models analyze data from various sources, including satellite imagery and on-ground sensors, to identify areas most affected by this issue. By predicting temperature changes, city planners can implement greening strategies that mitigate heat effects and improve urban living conditions.
Challenges in Using AI for Environmental Data Analysis
Data Quality and Availability
Despite its potential, AI’s effectiveness is contingent upon the quality and availability of data. Incomplete or biased datasets can lead to inaccurate models and predictions. Ensuring comprehensive and unbiased data collection remains a significant challenge.
Ethical Considerations
The deployment of AI in environmental monitoring raises ethical questions, particularly regarding privacy and surveillance. For instance, while drones equipped with AI sensors provide valuable data on deforestation, they could also intrude into private lands, raising concerns about consent and regulation.
Technical Complexity
Developing and maintaining sophisticated AI models require substantial technical expertise and resources. Smaller organizations or developing countries may struggle to access the necessary technology, leading to a digital divide in environmental monitoring capabilities.
Future Prospects of AI in Environmental Data Analysis
Enhanced Collaboration
The future will likely see increased collaboration between governments, NGOs, tech companies, and academia to harness AI’s full potential for environmental protection. Initiatives like open-source platforms can democratize access to AI tools, enabling more comprehensive global efforts to tackle environmental challenges.
Advancements in AI Technologies
As AI technologies continue to evolve, we can expect even more sophisticated models capable of providing deeper insights into environmental phenomena. For instance, advancements in quantum computing could drastically enhance AI’s data processing capabilities, leading to faster and more accurate predictions.
Integration with IoT Devices
The integration of AI with Internet of Things (IoT) devices will further revolutionize environmental monitoring. Networks of interconnected sensors can provide real-time data on various parameters, from air quality to soil moisture levels, offering a more holistic view of our planet’s health.
Conclusion
AI is transforming environmental data analysis by enhancing data processing capabilities, improving predictive accuracy, and enabling automation in data collection. It provides valuable sustainability insights across resource management, pollution control, and wildlife conservation, while also revolutionizing climate change modeling through enhanced predictive models, scenario analysis, and real-time monitoring. However, challenges such as data quality, ethical considerations, and technical complexity must be addressed to fully leverage AI’s potential.
The future of environmental data analysis lies in enhanced collaboration, advancements in AI technologies, and integration with IoT devices. By embracing these opportunities while addressing the associated challenges, we can pave the way for a more sustainable future, ensuring that our planet remains healthy for generations to come.