The Power of Predictive Analytics
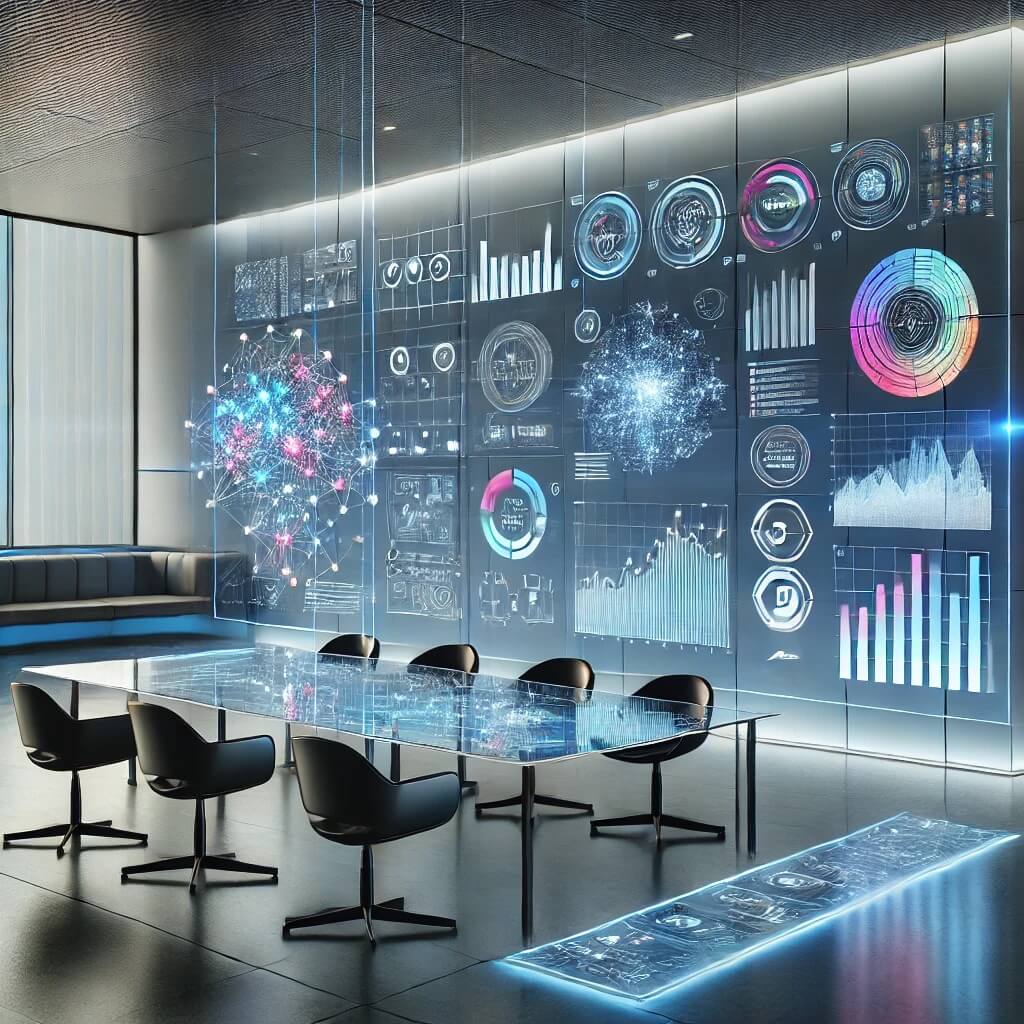
I. Introduction
Predictive analytics has revolutionized the way businesses make decisions, drive growth, and stay competitive in today’s fast-paced market. By harnessing the power of data-driven insights, organizations can anticipate customer needs, optimize operations, and identify new opportunities for revenue growth. However, traditional predictive analytics approaches often rely on historical data and linear models, which can be limited in their ability to capture complex patterns and relationships.
In recent years, the emergence of generative AI has opened up new possibilities for predictive analytics. Generative AI enables the creation of synthetic data that mimics real-world patterns and structures, allowing businesses to simulate various scenarios, test hypotheses, and make more informed decisions. By combining the strengths of traditional forecasting analytics with the innovative capabilities of generative AI, organizations can unlock significant business value, improve decision-making, and drive long-term success.
In this article, we will delve into the world of trend analytics and explore how generative AI can unlock business value by providing actionable insights, improving decision-making, and driving growth. We will discuss the role of generative AI in predictive analytics, highlight its benefits and case studies, and provide guidance on overcoming common challenges and limitations.
Key Highlights
- Predictive data analytics has revolutionized business decision-making and driven growth.
- Traditional approaches to predictive data analytics are limited by historical data and linear models.
- Generative AI offers new possibilities for predictive data analytics, enabling the creation of synthetic data that mimics real-world patterns and structures.
- Combining traditional predictive data analytics with generative AI can unlock significant business value and drive long-term success.
II. What is Predictive Analytics?
Outcome analytics is a statistical technique that uses historical data, machine learning algorithms, and other techniques to predict future events or outcomes. It involves analyzing large datasets to identify patterns, trends, and relationships that can inform business decisions. The goal of outcome analytics is to provide actionable insights that enable organizations to anticipate customer needs, optimize operations, and drive growth.
Key Concepts in Outcome Analytics
- Data Preparation and Visualization: This involves cleaning, transforming, and visualizing data to prepare it for analysis.
- Model Development and Selection: This involves selecting the most suitable machine learning algorithm or statistical model to analyze the data and make predictions.
- Deployment and Maintenance: This involves implementing the predictive model in a production environment and monitoring its performance over time.
Types of Predictive Analytics
- Descriptive Analytics: This type of analytics provides historical insights into past events, behaviors, and trends.
- Diagnostic Analytics: This type of analytics helps identify the root causes of problems or issues.
- Predictive Analytics: This type of analytics uses machine learning algorithms to forecast future events or outcomes.
- Prescriptive Analytics: This type of analytics provides recommendations on what actions to take based on predicted outcomes.
Benefits of Predictive Analytics
- Improved Decision-Making: Data analytics enables organizations to make informed decisions by providing accurate forecasts and predictions.
- Increased Efficiency: Data analytics can help optimize business processes, reduce waste, and improve resource allocation.
- Enhanced Customer Experience: Data analytics can help organizations anticipate customer needs and provide personalized experiences.
III. The Role of Generative AI in Analytics
Generative AI has emerged as a powerful tool for insight analytics, enabling the creation of synthetic data that mimics real-world patterns and structures. This technology allows businesses to simulate various scenarios, test hypotheses, and make more informed decisions.
How Generative AI Enhances Predictive Analytics
- Improved Model Accuracy: Gen AI can generate synthetic data that is similar in distribution and structure to the original data, allowing for more accurate modeling.
- Increased Sample Sizes and Diversity: Gen AI can create new data samples that are similar to existing ones but with increased diversity, enabling more robust models.
- Enhanced Feature Engineering and Selection: Gen AI can help identify relevant features and select the most important variables for analysis.
Applications of Generative AI in Predictive Analytics
- Demand Forecasting: Gen AI can be used to predict demand based on historical sales data, taking into account factors such as seasonality, trends, and external influences.
- Risk Analysis: Gen AI can help identify potential risks by simulating various scenarios and predicting outcomes.
- Customer Segmentation: Gen AI can create synthetic customer profiles that mimic real-world behaviors, enabling more accurate segmentation and targeting.
Benefits of Combining Predictive Analytics with Generative AI
- More Accurate Forecasts: The combination of traditional predictive analytics with generative AI enables the creation of more accurate forecasts.
- Increased Confidence in Decision-Making: The use of generative AI can increase confidence in decision-making by providing a range of possible outcomes and scenarios.
- Improved Resource Allocation: The combination of predictive analytics with generative AI can help optimize resource allocation and reduce waste.
III. The Role of Generative AI in Anticipatory Analytics
Generative AI has emerged as a powerful tool for anticipatory analytics, enabling the creation of synthetic data that mimics real-world patterns and structures. This technology allows businesses to simulate various scenarios, test hypotheses, and make more informed decisions.
How Generative AI Enhances Predictive Analytics
- Improved Model Accuracy: Generative AI can generate synthetic data that is similar in distribution and structure to the original data, allowing for more accurate modeling.
- Increased Sample Sizes and Diversity: Generative AI can create new data samples that are similar to existing ones but with increased diversity, enabling more robust models.
- Enhanced Feature Engineering and Selection: Generative AI can help identify relevant features and select the most important variables for analysis.
Applications of Generative AI in Predictive Analytics
- Demand Forecasting: Generative AI can be used to predict demand based on historical sales data, taking into account factors such as seasonality, trends, and external influences.
- Risk Analysis: Generative AI can help identify potential risks by simulating various scenarios and predicting outcomes.
- Customer Segmentation: Generative AI can create synthetic customer profiles that mimic real-world behaviors, enabling more accurate segmentation and targeting.
Benefits of Combining Predictive Analytics with Generative AI
- More Accurate Forecasts: The combination of traditional anticipatory analytics with generative AI enables the creation of more accurate forecasts.
- Increased Confidence in Decision-Making: The use of generative AI can increase confidence in decision-making by providing a range of possible outcomes and scenarios.
- Improved Resource Allocation: The combination of anticipatory analytics with generative AI can help optimize resource allocation and reduce waste.
IV. Benefits of Using Generative AI in Outcome Analytics
Using generative AI in future analytics can bring numerous benefits to businesses, enabling them to make more informed decisions and drive growth. Some of the key advantages include:
Improved Business Outcomes
- Increased Revenue: By accurately predicting demand and optimizing resource allocation, businesses can increase revenue and profitability.
- Reduced Costs: Generative AI can help identify areas where costs can be reduced, such as through improved supply chain management or optimized operations.
- Enhanced Customer Satisfaction: Predictive analytics with generative AI can enable businesses to better understand customer needs and preferences, leading to increased satisfaction.
Enhanced Decision-Making
- Actionable Insights: Generative AI provides actionable insights that inform business decisions, enabling organizations to anticipate customer needs and stay ahead of the competition.
- Increased Confidence in Decision-Making: The use of generative AI can increase confidence in decision-making by providing a range of possible outcomes and scenarios.
- Improved Resource Allocation: Predictive analytics with generative AI can help optimize resource allocation and reduce waste.
Competitive Advantage
- Differentiation: By leveraging predictive analytics with generative AI, businesses can differentiate themselves from competitors and establish a unique market position.
- Innovation: Generative AI enables the creation of new products, services, and experiences that meet evolving customer needs and preferences.
- Increased Agility: Predictive analytics with generative AI can help organizations respond quickly to changing market conditions and stay ahead of the competition.
V. Case Studies: Real-World Applications of Generative AI in Future Trend Analytics
In this section, we will explore real-world case studies that demonstrate the application of generative AI in predictive analytics. These examples illustrate how businesses have leveraged generative AI to drive business value and achieve success.
Company XYZ: A Telecommunications Case Study
Background: Telco Company XYZ is a telecommunications company with a large customer base. They wanted to predict which customers were most likely to upgrade their plans or add new services.
Solution: The company’s data science team used generative AI to analyze customer data, including usage patterns, billing information, and demographic details. They trained a predictive model that could accurately forecast the likelihood of customers upgrading or adding new services based on these factors.
Results: By using generative AI, Company XYZ was able to identify high-value customers who were most likely to upgrade their plans or add new services. This enabled them to target these customers with personalized promotions and offers, resulting in a significant increase in revenue growth and customer satisfaction.
Key Takeaways
- Generative AI can be used to predict complex behaviors and outcomes in various industries.
- By leveraging generative AI, businesses can identify high-value customers and tailor their marketing efforts to meet their needs.
- The use of generative AI can lead to increased revenue growth, improved customer satisfaction, and a competitive edge.
VI. Overcoming Challenges and Limitations
While generative AI has the potential to revolutionize predictive analytics, there are several challenges and limitations that need to be addressed.
Common Challenges
- Data Quality Issues: The quality of data used in generative AI models is crucial for accuracy and reliability.
- Model Interpretability: Generative AI models can be complex and difficult to interpret, making it challenging to understand the underlying decision-making process.
- Regulatory Compliance: There are regulations and laws that govern the use of generative AI in various industries, such as finance, healthcare, and education.
Mitigation Strategies
- Data Preparation and Cleaning: Ensuring that data is accurate, complete, and up-to-date can help to mitigate data quality issues.
- Model Selection and Validation: Carefully selecting and validating models can help to ensure that they are reliable and accurate.
- Collaboration with Stakeholders: Working closely with stakeholders, such as business leaders, data scientists, and subject matter experts, can help to address regulatory compliance and model interpretability issues.
Addressing Data Quality Issues
- Data Governance: Establishing a data governance framework can help to ensure that data is accurate, complete, and up-to-date.
- Data Validation and Verification: Validating and verifying data can help to identify errors and inconsistencies.
- Data Standardization: Standardizing data formats and structures can help to improve data quality.
Addressing Model Interpretability
- Model Explainability Techniques: Using techniques such as feature importance, partial dependence plots, and SHAP values can help to explain model decisions.
- Business Domain Knowledge: Understanding the business domain and context can help to interpret model results and make informed decisions.
- Collaboration with Subject Matter Experts: Working closely with subject matter experts can help to ensure that models are aligned with business goals and objectives.
Addressing Regulatory Compliance
- Regulatory Frameworks: Familiarizing yourself with regulatory frameworks, such as GDPR, HIPAA, and COPPA, can help to ensure compliance.
- Risk Assessment: Conducting a risk assessment can help to identify potential risks and develop strategies to mitigate them.
- Collaboration with Compliance Experts: Working closely with compliance experts can help to ensure that models are compliant with regulatory requirements.
VII. Conclusion to Predictive Analytics
In this article, we have explored the power of predictive analytics and how it can be enhanced by using generative AI. We have discussed the benefits of combining traditional predictive analytics with generative AI, including improved accuracy, increased confidence in decision-making, and better resource allocation.
We have also examined some real-world case studies that demonstrate the application of generative AI in predictive analytics, including a telecommunications company that used generative AI to predict customer behavior and optimize marketing campaigns.
Furthermore, we have discussed some common challenges and limitations associated with generative AI, such as data quality issues, model interpretability, and regulatory compliance. We have also provided some mitigation strategies for addressing these challenges, including ensuring data quality, selecting and validating models, and collaborating with stakeholders.
In conclusion, the combination of traditional predictive analytics and generative AI has the potential to revolutionize business decision-making and drive growth. By leveraging this technology, businesses can unlock new insights, make more informed decisions, and stay ahead of the competition.
Key Takeaways
- Predictive analytics can be enhanced by using generative AI.
- The combination of traditional predictive analytics and generative AI has the potential to revolutionize business decision-making and drive growth.
- Common challenges associated with generative AI include data quality issues, model interpretability, and regulatory compliance.
- Mitigation strategies for addressing these challenges include ensuring data quality, selecting and validating models, and collaborating with stakeholders.
Future Directions
The future of predictive analytics is bright, and the use of generative AI is likely to play an increasingly important role in this field. As this technology continues to evolve, we can expect to see even more sophisticated applications of predictive analytics in a wide range of industries.
Some potential areas for future research include:
- Developing more advanced techniques for handling complex data and improving model interpretability.
- Investigating the use of generative AI in other domains, such as natural language processing and computer vision.
- Exploring new ways to integrate predictive analytics with other technologies, such as robotics and Internet of Things (IoT).
By continuing to innovate and push the boundaries of what is possible with predictive analytics and generative AI, we can unlock even more value for businesses and society as a whole.