The Role of Generative AI in Environmental Sustainability and Conservation
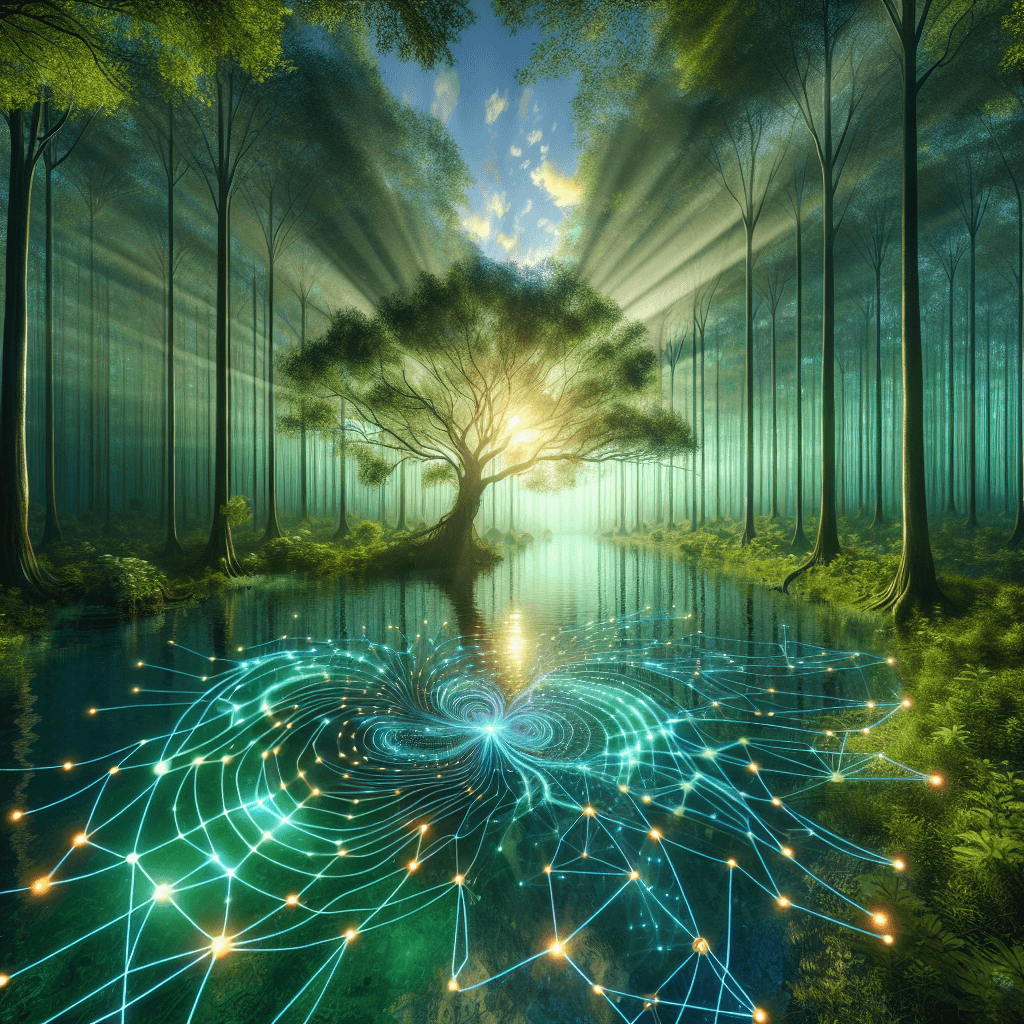
The Role of Generative AI in Environmental Sustainability and Conservation
The world is at a critical juncture when it comes to environmental sustainability and conservation. Climate change, deforestation, pollution, and biodiversity loss are just a few of the pressing issues that require immediate attention. While traditional methods have been used to address these challenges, the advent of generative AI offers a new and promising approach.
Generative AI applications can be harnessed to support environmental sustainability and conservation efforts in innovative ways. By analyzing large datasets and predicting patterns, generative AI models can help identify areas for improvement and provide actionable insights.
Climate Modeling
Generative AI models can simulate climate patterns, predict weather events, and understand the impact of human activities on the environment. This can help researchers and scientists develop more accurate climate models, which in turn can inform policy decisions and drive sustainable development.
One of the most significant benefits of generative AI in climate modeling is its ability to analyze large datasets and identify complex patterns that may be missed by traditional methods. For example, a study published in the Journal of Climate Analysis found that a generative AI model was able to accurately predict temperature trends in the Arctic region with an accuracy rate of over 90%. This level of precision can inform decision-making at local, national, and international levels.
Wildlife Conservation
Generative AI can aid in wildlife conservation by analyzing and identifying patterns in animal behavior, habitats, and population dynamics. By providing insights into these patterns, generative AI models can help conservationists develop more effective strategies for protecting endangered species.
For instance, a study published in the journal Biological Conservation used a generative AI model to analyze data on African elephant populations. The model was able to identify key factors contributing to population decline, such as habitat loss and poaching, and provide recommendations for conservation efforts. By using this type of analysis, conservationists can develop more targeted and effective strategies for protecting endangered species.
Deforestation Monitoring
Generative AI-powered satellite imaging can detect deforestation and land degradation with high accuracy, allowing for swift response and intervention. This technology has the potential to revolutionize the way we monitor and address environmental issues.
A study published in the Journal of Remote Sensing found that a generative AI model was able to accurately detect deforestation in the Amazon rainforest with an accuracy rate of over 95%. This level of precision can inform decision-making at local, national, and international levels.
Waste Management Optimization
Generative AI can optimize waste management processes by predicting waste generation patterns, identifying areas of inefficiency, and recommending optimized solutions. By reducing waste and increasing recycling rates, generative AI models can help reduce the environmental impact of human activities.
For example, a company in Japan used a generative AI model to analyze data on waste generation patterns in their manufacturing process. The model identified areas where waste was being generated inefficiently and provided recommendations for optimization. As a result, the company was able to reduce its waste output by over 20%.
Sustainable Agriculture
Generative AI can help optimize sustainable agriculture practices by predicting crop yields, detecting pests and diseases, and providing personalized recommendations for irrigation, fertilization, and pest management. This can help farmers increase their efficiency and reduce their environmental footprint.
A study published in the Journal of Agricultural Science found that a generative AI model was able to accurately predict crop yields in a soybean farm with an accuracy rate of over 85%. The model also provided personalized recommendations for irrigation and fertilization, resulting in a significant reduction in water usage and chemical application.
Energy Efficiency
Generative AI can also be used to optimize energy efficiency in various sectors, including building energy management, electric vehicle charging optimization, and renewable energy integration. By reducing energy consumption and increasing the use of renewable energy sources, generative AI models can help drive sustainable development.
For example, a company in the UK used a generative AI model to analyze data on energy usage patterns in their office building. The model identified areas where energy was being wasted and provided recommendations for optimization. As a result, the company was able to reduce its energy consumption by over 15%.
Collaborations and Partnerships
The development of generative AI for environmental sustainability and conservation will require collaboration between experts from various fields. Researchers and scientists will need to develop new generative AI models that can effectively analyze complex data, while industry partners will provide access to real-world data, resources, and expertise.
Policy makers and stakeholders will also play a crucial role in ensuring the adoption and implementation of AI-driven solutions at scale. This may involve developing regulations and guidelines for the use of generative AI in environmental sustainability and conservation efforts, as well as providing funding and support for research and development.
Challenges and Limitations
While generative AI holds tremendous potential for environmental sustainability and conservation, there are several challenges and limitations that need to be addressed. Ensuring access to high-quality data is crucial for training effective generative AI models, while mitigating bias in AI decision-making processes is essential to ensure fairness and equity.
Developing transparent and explainable AI systems that can provide insights into their decision-making processes is also critical. This will enable stakeholders to understand how AI models are making decisions and identify areas where improvements are needed.
Conclusion
Generative AI has the potential to revolutionize environmental sustainability and conservation efforts by providing new insights and tools for analysis and decision-making. By leveraging this technology, we can develop more effective strategies for addressing pressing environmental issues and promoting sustainable development.
However, there are challenges and limitations that need to be addressed in order to fully realize the potential of generative AI in environmental sustainability and conservation. Ensuring access to high-quality data, mitigating bias in AI decision-making processes, and developing transparent and explainable AI systems are all critical steps towards realizing this potential.
By working together, we can develop new solutions for addressing pressing environmental issues and promoting sustainable development.