Unleashing Creative Potential with Generative AI-Powered Data Visualization
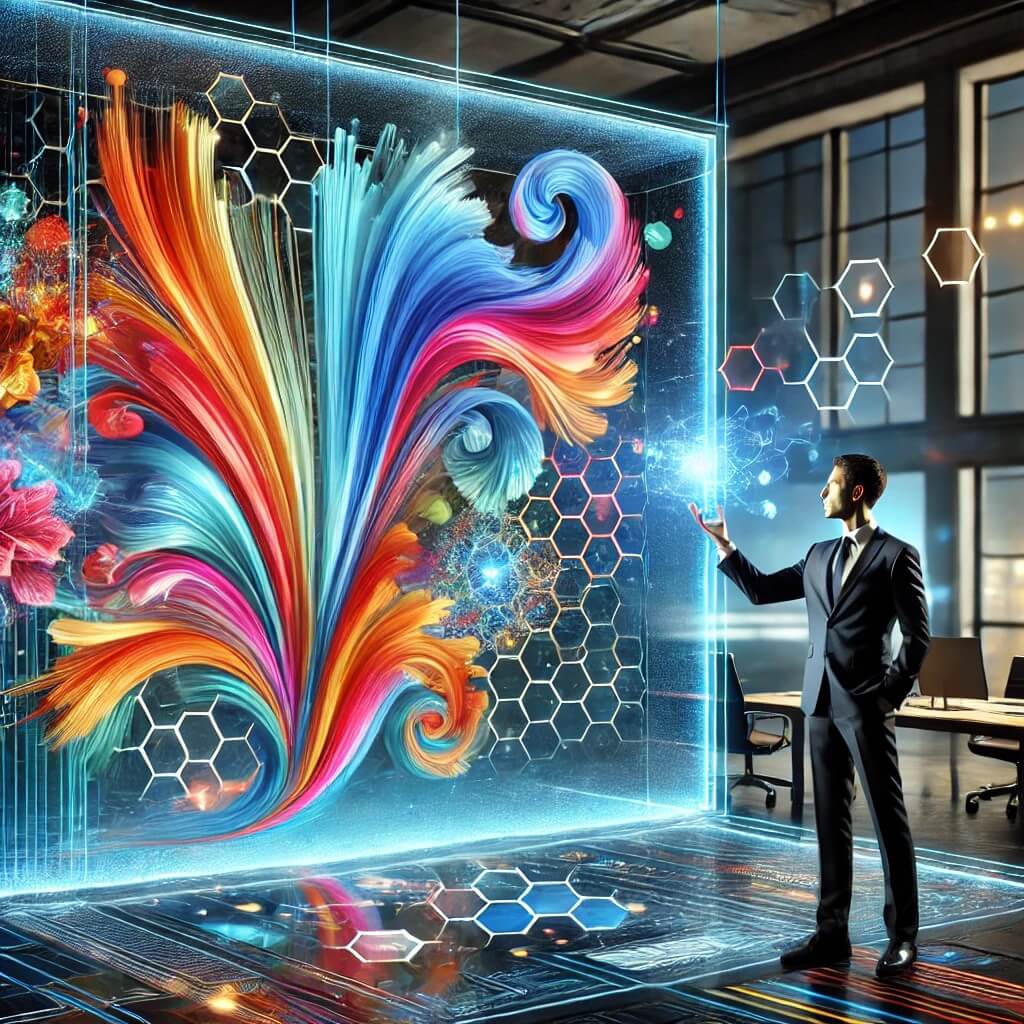
Introduction
The world of data visualization has come a long way since its early days as a simple means of communicating complex information. Today, data visualization is an essential tool for organizations across various industries, helping them to make sense of vast amounts of data and drive business growth. However, despite the advancements in data visualization techniques, many organizations still struggle to effectively communicate their insights to stakeholders. This is where generative AI-powered data visualization comes into play – a game-changing technology that has the potential to unlock new possibilities for creative potential.
The Importance of Data Visualization
Data visualization is more than just a pretty picture; it’s a powerful tool that helps organizations to:
- Communicate complex information in an easily digestible format
- Identify trends and patterns in data that might go unnoticed otherwise
- Make informed decisions based on data-driven insights
- Drive business growth by optimizing processes and improving outcomes
However, traditional data visualization techniques often fall short of meeting these needs. Manual design can be time-consuming and prone to bias, while scalability is often a challenge when working with large datasets.
The Rise of Generative AI-Powered Data Visualization
Gen AI-powered data visualization represents a significant departure from traditional methods. By leveraging the power of machine learning algorithms, organizations can create new, unique visualizations from existing data. This technology has the potential to revolutionize the way we communicate complex information and drive business growth.
In the following sections, we’ll delve deeper into the world of gen AI-powered data visualization, exploring its benefits, limitations, and real-world applications. We’ll also provide a step-by-step guide on how to implement this emerging technology in your organization, unlocking new possibilities for creative potential.
The Limitations of Traditional Data Visualization
While traditional data visualization techniques have been widely adopted across various industries, they often fall short in meeting the complex needs of modern organizations. Here are some limitations of traditional data visualization:
1. Manual Design and Bias
Traditional data visualization relies heavily on manual design, which can be time-consuming and prone to bias. When designers create visualizations based on their personal experiences or assumptions, it can lead to inaccurate or incomplete representations of the data. This can result in poor decision-making and wasted resources.
Example: A marketing team creates a dashboard to track customer engagement metrics. However, due to manual design and bias, they focus on vanity metrics like social media followers rather than actual customer behavior. As a result, their decisions lead to inefficient resource allocation and missed opportunities.
2. Lack of Scalability
Traditional data visualization often struggles with large datasets, which can be overwhelming for designers to process and visualize effectively. This leads to compromised visualizations that don’t accurately represent the data, resulting in poor decision-making.
Example: A financial institution tries to track millions of transactions using traditional data visualization techniques. However, due to the sheer volume of data, they end up simplifying their visualizations too much, losing critical insights and missing potential risks.
3. Limited Interactivity
Traditional data visualization often lacks interactivity, making it difficult for users to explore and analyze the data in-depth. This can result in a shallow understanding of the data and missed opportunities for deeper analysis.
Example: A healthcare organization creates a dashboard to track patient outcomes. However, due to limited interactivity, users can only view static visualizations, missing critical insights into the impact of different treatments on patient outcomes.
4. Difficulty Communicating Complex Information
Traditional data visualization often struggles with complex information, making it challenging for organizations to effectively communicate their insights to stakeholders. This can result in missed opportunities and poor decision-making.
Example: A scientific research team creates a report to share with policymakers. However, due to the complexity of the data and traditional visualization techniques, they struggle to communicate their findings effectively, leading to misunderstandings and misinterpretations.
These limitations highlight the need for innovative solutions that can overcome the challenges of traditional data visualization. Gen AI data visualization represents a significant departure from these traditional methods, offering new possibilities for creative potential and effective communication of complex information.
What is Generative AI-Powered Data Visualization?
Gen AI data visualization represents a significant departure from traditional data visualization techniques. It leverages the power of machine learning algorithms to create new, unique visualizations from existing data. This technology has the potential to revolutionize the way we communicate complex information and drive business growth.
What is Generative AI?
Generative AI refers to a type of machine learning algorithm that can generate new, synthetic data or content based on patterns and structures learned from existing data. In the context of data visualization, generative AI can be used to create new visualizations, such as plots, charts, and graphs, that are tailored to specific data analysis tasks.
How Does Generative AI-Powered Data Visualization Work?
Gen AI data visualization works by using machine learning algorithms to analyze existing data and identify patterns and relationships. These patterns are then used to create new visualizations that can be customized for specific data analysis tasks.
Here’s a high-level overview of the process:
- Data Collection: Gather relevant data from various sources.
- Data Preprocessing: Clean, transform, and format the data for analysis.
- Model Training: Train a machine learning model on the preprocessed data to identify patterns and relationships.
- Visualization Generation: Use the trained model to generate new visualizations that are tailored to specific data analysis tasks.
- Post-processing: Refine and customize the generated visualizations as needed.
Benefits of Generative AI-Powered Data Visualization
Generative AI-powered data visualization offers several benefits, including:
- Increased creativity and innovation: By leveraging machine learning algorithms, organizations can create new, unique visualizations that can help to drive business growth.
- Improved scalability and efficiency: Generative AI-powered data visualization can handle large datasets with ease, reducing the time and effort required for data analysis.
- Enhanced user engagement and insights: Customizable visualizations can be tailored to specific user needs, improving user engagement and providing deeper insights into complex data.
Real-World Applications
Generative AI-powered data visualization has a wide range of real-world applications, including:
- Marketing and advertising: Use generative AI-powered data visualization to create customized visualizations for marketing campaigns.
- Healthcare and medical research: Apply generative AI-powered data visualization to analyze complex medical data and identify new trends and patterns.
- Financial analysis and prediction: Use generative AI-powered data visualization to create predictive models that can forecast financial trends and outcomes.
Benefits of Generative AI-Powered Data Visualization
Generative AI-powered data visualization offers a wide range of benefits that can help organizations to improve their decision-making processes, drive business growth, and enhance user engagement. Here are some of the key benefits of this technology:
1. Increased Creativity and Innovation
Gen AI data visualization allows organizations to create new, unique visualizations that can help to drive business growth. By leveraging machine learning algorithms, organizations can generate new ideas and insights that might not have been possible with traditional data visualization techniques.
Example: A marketing team uses gen AI data visualization to create customized visualizations for their marketing campaigns. The generated visualizations reveal new trends and patterns in customer behavior, leading to more effective targeting and increased sales.
2. Improved Scalability and Efficiency
Gen AI data visualization can handle large datasets with ease, reducing the time and effort required for data analysis. This means that organizations can process and visualize vast amounts of data quickly and efficiently, making it easier to identify trends and patterns.
Example: A financial institution uses generative AI-powered data visualization to analyze millions of transactions. The technology allows them to generate visualizations in real-time, enabling them to detect potential risks and take corrective action before they become major issues.
3. Enhanced User Engagement and Insights
Generative AI-powered data visualization can be customized for specific user needs, improving user engagement and providing deeper insights into complex data. This means that organizations can create visualizations that are tailored to the needs of their users, making it easier to communicate complex information and drive business growth.
Example: A healthcare organization uses generative AI-powered data visualization to analyze patient outcomes. The generated visualizations reveal new trends and patterns in patient behavior, enabling them to develop more effective treatments and improve patient care.
4. Better Decision-Making
Generative AI-powered data visualization can help organizations to make better decisions by providing deeper insights into complex data. By leveraging machine learning algorithms, organizations can generate visualizations that highlight key trends and patterns, making it easier to identify opportunities and mitigate risks.
Example: A scientific research team uses generative AI-powered data visualization to analyze complex medical data. The generated visualizations reveal new trends and patterns in patient behavior, enabling them to develop more effective treatments and improve patient care.
5. Cost Savings
Generative AI-powered data visualization can help organizations to save costs by reducing the need for manual data analysis and visualization. By leveraging machine learning algorithms, organizations can generate visualizations quickly and efficiently, making it easier to identify trends and patterns without the need for extensive manual analysis.
Example: A financial institution uses generative AI-powered data visualization to analyze millions of transactions. The technology allows them to generate visualizations in real-time, reducing the need for manual analysis and saving costs on personnel and infrastructure.
Real-World Applications and Use Cases
Generative AI-powered data visualization has a wide range of real-world applications across various industries, including marketing, healthcare, finance, education, and more. Here are some examples of how this technology can be used in practice:
1. Marketing and Advertising
- Targeted advertising: Use generative AI-powered data visualization to create customized visualizations for targeted advertising campaigns.
- Marketing analytics: Leverage machine learning algorithms to analyze customer behavior and preferences, providing insights on effective marketing strategies.
- Product recommendation engines: Use generative AI-powered data visualization to develop product recommendation engines that suggest relevant products based on user behavior.
Example: A e-commerce company uses generative AI-powered data visualization to create customized visualizations for targeted advertising campaigns. The generated visualizations reveal new trends and patterns in customer behavior, leading to more effective targeting and increased sales.
2. Healthcare and Medical Research
- Patient outcomes analysis: Use generative AI-powered data visualization to analyze patient outcomes and identify new trends and patterns in medical research.
- Medical imaging analysis: Leverage machine learning algorithms to analyze medical images and provide insights on disease diagnosis and treatment.
- Clinical trial design: Use generative AI-powered data visualization to develop clinical trial designs that optimize patient recruitment and study outcomes.
Example: A healthcare organization uses generative AI-powered data visualization to analyze patient outcomes. The generated visualizations reveal new trends and patterns in patient behavior, enabling them to develop more effective treatments and improve patient care.
3. Finance and Banking
- Risk analysis: Use generative AI-powered data visualization to analyze financial risk and identify potential threats to business stability.
- Portfolio optimization: Leverage machine learning algorithms to optimize investment portfolios and provide insights on asset allocation.
- Financial forecasting: Use generative AI-powered data visualization to develop financial forecasts that predict future trends and outcomes.
Example: A financial institution uses generative AI-powered data visualization to analyze millions of transactions. The technology allows them to generate visualizations in real-time, enabling them to detect potential risks and take corrective action before they become major issues.
4. Education
- Student performance analysis: Use generative AI-powered data visualization to analyze student performance and identify trends and patterns in educational outcomes.
- Curriculum design: Leverage machine learning algorithms to develop curriculum designs that optimize student engagement and learning outcomes.
- Teacher evaluation: Use generative AI-powered data visualization to evaluate teacher effectiveness and provide insights on professional development.
Implementation Roadmap for Generative AI-Powered Data Visualization
Implementing AI-powered data visualization in an organization requires a strategic approach to ensure successful adoption and integration with existing infrastructure and workflows. Here’s a step-by-step guide on how to implement this technology:
1. Define Business Goals and Objectives
- Identify the business needs that can be addressed through AI-powered data visualization.
- Determine the specific use cases and applications for this technology.
- Develop clear objectives and metrics for success.
Example: A financial institution aims to improve risk analysis and portfolio optimization using AI-powered data visualization. They define specific goals, such as reducing risk by 10% and increasing returns on investment (ROI) by 5%.
2. Assess Current Data Infrastructure
- Evaluate the existing data infrastructure, including data quality, availability, and formats.
- Identify potential gaps or limitations that may impact implementation.
- Determine the necessary upgrades or modifications to support generative AI-powered data visualization.
Example: A healthcare organization assesses their current data infrastructure and finds that they need to upgrade their data management system to accommodate the demands of generative AI-powered data visualization.
3. Choose the Right Tools and Technologies
- Select suitable tools and technologies for generating visualizations, such as machine learning algorithms and data visualization software.
- Consider factors like scalability, flexibility, and user-friendliness.
- Ensure compatibility with existing infrastructure and workflows.
Example: A marketing team chooses a specific AI-powered data visualization tool that can integrate with their existing customer relationship management (CRM) system.
4. Integrate with Existing Infrastructure and Workflows
- Design an integration plan to incorporate generative AI-powered data visualization into existing processes.
- Develop strategies for data sharing, collaboration, and communication between teams.
- Ensure seamless workflow and minimal disruption to business operations.
Example: A financial institution integrates AI-powered data visualization with their existing risk management system, enabling them to generate visualizations in real-time and make informed decisions.
5. Train and Upskill Personnel
- Develop training programs for staff to learn about generative AI-powered data visualization.
- Provide education on using the technology effectively and interpreting results.
- Ensure that personnel are equipped with the necessary skills to use this technology successfully.
Example: An educational institution provides training sessions for teachers on using gen AI-powered data visualization to analyze student performance and develop more effective teaching strategies.
6. Monitor Progress and Evaluate Success
- Establish metrics to track progress and evaluate success.
- Regularly review and assess the effectiveness of generative AI-powered data visualization in achieving business goals.
- Make adjustments as needed to optimize implementation and maximize benefits.
Conclusion
Gen AI-powered data visualization has revolutionized the way organizations analyze and interpret complex data, providing insights that were previously unimaginable. By leveraging machine learning algorithms and data visualization techniques, businesses can unlock new opportunities for growth, improve decision-making, and drive innovation.
In this article, we have explored the benefits of generative AI visualization, including increased creativity and innovation, improved scalability and efficiency, enhanced user engagement and insights, better decision-making, and cost savings. We have also discussed real-world applications and use cases across various industries, such as marketing, healthcare, finance, education, and more.
Implementing gen AI-powered data visualization requires a strategic approach to ensure successful adoption and integration with existing infrastructure and workflows. By defining business goals and objectives, assessing current data infrastructure, choosing the right tools and technologies, integrating with existing infrastructure and workflows, training and upskilling personnel, and monitoring progress and evaluating success, organizations can maximize the benefits of this technology.
As gen AI-powered data visualization continues to evolve and improve, its potential applications will only continue to grow. With its ability to unlock new insights and drive business growth, this technology is sure to have a significant impact on various industries in the years to come.
Recommendations
- Organizations should consider implementing generative AI-powered data visualization to unlock new opportunities for growth and improvement.
- Businesses should invest in training and upskilling personnel to ensure they are equipped with the necessary skills to use this technology effectively.
- Companies should assess their current data infrastructure and make necessary upgrades or modifications to support generative AI-powered data visualization.
- Organizations should integrate generative AI-powered data visualization with existing processes and workflows to maximize its benefits.
By following these recommendations, organizations can stay ahead of the curve and reap the rewards of generative AI-powered data visualization.