Unveiling Innovations in Vector Database Technology
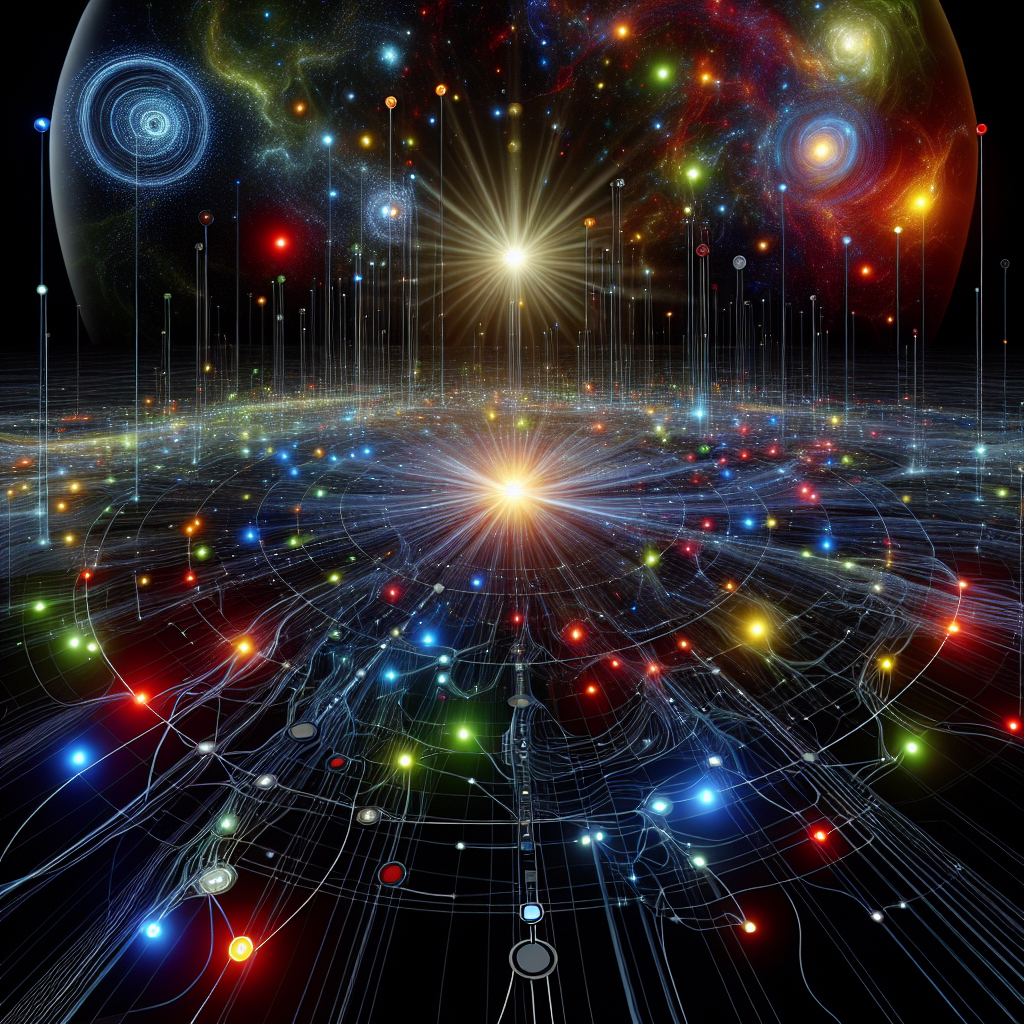
Introduction
The digital landscape is evolving at an unprecedented rate, driven by advancements in artificial intelligence (AI) and machine learning. At the heart of this transformation are vector databases—specialized databases designed for managing complex datasets through high-dimensional vectors. Recent innovations have propelled these technologies into new realms, enhancing processing speeds, optimizing semantic searches, and providing AI-driven data management solutions. This blog post explores the latest advancements in vector database technology, highlighting contributions from entities like Microsoft Azure AI, OpenAI’s research team, and Stanford University.
The Evolution of Vector Databases
Vector databases have significantly evolved since their inception. Initially crafted for simple data retrieval tasks, these systems now support complex operations involving large-scale unstructured data. Recent breakthroughs have enabled faster processing speeds within vector databases, significantly improving query response times for intricate datasets.
Key Advancements
- Faster Processing Speeds: By leveraging optimized algorithms and hardware acceleration, vector databases achieve unprecedented speed in data retrieval.
- Semantic Search Optimization: These databases now offer enhanced capabilities to understand the context behind queries, delivering more accurate search results that align with user intent.
- AI-driven Data Management Solutions: Integrating machine learning algorithms into vector databases has transformed their ability to analyze and interpret unstructured data efficiently. This integration is crucial in industries dealing with massive datasets such as healthcare, finance, and e-commerce.
Enhancing Semantic Search
One of the most transformative aspects of recent advancements in vector database technology is semantic search optimization. Traditional keyword-based searches often fall short when it comes to understanding context or intent behind a user’s query. Vector databases address this gap by representing data in multi-dimensional spaces where relationships between data points are naturally understood.
How Machine Learning Plays a Role
- Improved Query Interpretation: By using machine learning models, vector databases can analyze search queries more accurately and provide results that align with the semantic meaning rather than just keyword matches.
- Contextual Relevance: These systems consider user context such as location, history, and preferences to deliver personalized search experiences.
Recent breakthroughs have enabled faster processing speeds within vector databases, significantly improving query response times for complex datasets. The integration of machine learning algorithms enhances the ability to analyze and interpret large-scale unstructured data efficiently, making semantic search optimization a critical advancement in this field.
Impact on Industries
The latest advancements in vector database technology are revolutionizing industries by providing powerful tools for data analysis and management:
- Healthcare: By quickly analyzing vast amounts of patient data, healthcare providers can offer more personalized treatment plans, improving patient outcomes. Vector databases enable the integration of genomic data with electronic health records, facilitating advanced diagnostics and targeted therapies.
- Finance: Financial institutions benefit from enhanced data security and fraud detection capabilities, leading to more informed decision-making and strategic planning. By processing high-dimensional financial datasets swiftly, vector databases support real-time analytics for risk management and investment strategies.
- E-commerce: Online retailers leverage vector databases to enhance recommendation engines, providing customers with personalized shopping experiences based on their browsing history and preferences. This capability not only boosts customer satisfaction but also increases sales conversion rates.
The integration of machine learning algorithms into vector databases enhances the ability to analyze and interpret large-scale unstructured data efficiently, making these advancements invaluable across various sectors.
Role of Key Contributors
Microsoft Azure AI
Microsoft Azure AI is at the forefront of developing cutting-edge solutions for vector database advancements. Their cloud-based services provide scalable platforms that support fast processing speeds and sophisticated semantic search optimization techniques. With tools like Azure Cognitive Search, businesses can implement intelligent search capabilities that enhance user experience by understanding natural language queries.
OpenAI’s Research Team
OpenAI’s research team has been instrumental in pushing the boundaries of AI-driven data management solutions. Their innovative approaches to machine learning enable more efficient data analysis and interpretation, setting new standards in vector database technology. Recent collaborations with industry leaders have resulted in enhanced models that further improve the accuracy and reliability of semantic searches.
Stanford University
Stanford University contributes through extensive research initiatives that explore optimization techniques for vector databases. These efforts foster innovation and set new benchmarks within the industry. Their work on scalable algorithms and efficient data indexing methods has paved the way for more robust vector database applications, particularly in fields like bioinformatics and social network analysis.
Case Studies and Real-World Applications
To illustrate the practical impact of these advancements, consider a few real-world case studies:
- Smart City Initiatives: Cities are utilizing vector databases to manage and analyze data from IoT sensors. This enables efficient urban planning and resource management by predicting traffic patterns and optimizing public transport routes.
- Content Streaming Platforms: Companies like Netflix use vector databases for content recommendation systems. By analyzing user interactions with high-dimensional data, these platforms can suggest relevant shows and movies, enhancing viewer engagement.
These examples underscore the versatility and power of modern vector database technologies in transforming various sectors by enabling more insightful data-driven decision-making.
Future Trends and Potential
Looking ahead, several trends are likely to shape the future of vector databases:
- Integration with Edge Computing: As edge computing gains traction, vector databases will be crucial for processing data closer to its source. This integration will reduce latency and improve real-time analytics capabilities.
- Advancements in AI Models: Continued improvements in AI models, such as transformer architectures, will further enhance the accuracy of semantic searches within vector databases.
- Increased Emphasis on Data Privacy: With growing concerns over data privacy, future developments may focus on techniques like federated learning to ensure secure and private data processing across distributed systems.
Conclusion
The evolution of vector database technology marks a significant leap in our ability to manage and derive insights from complex datasets. By optimizing for faster processing speeds, enhancing semantic search capabilities, and integrating AI-driven solutions, these databases are set to revolutionize industries ranging from healthcare to finance.