Using AI in Financial Market Trends
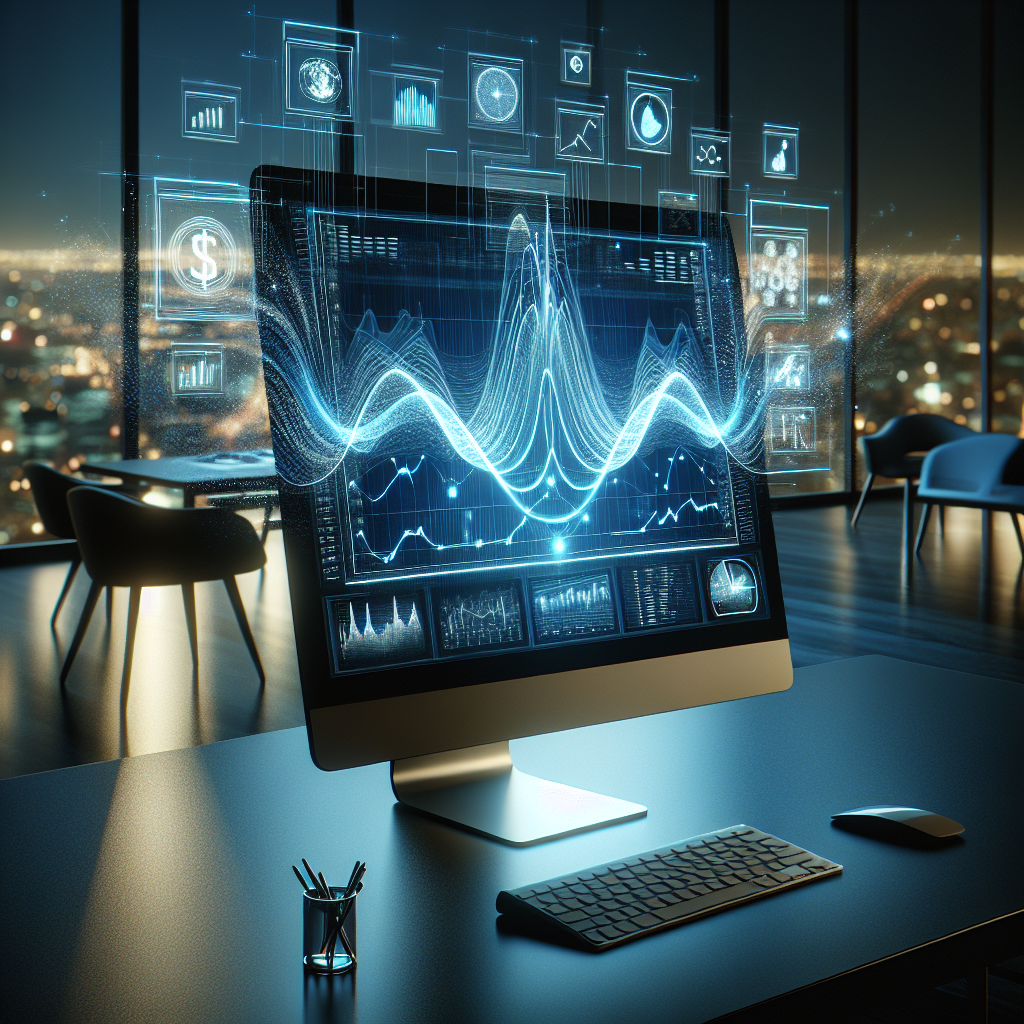
The Role of AI in Financial Market Trends: Driving Insights and Decision-Making
The world of finance is evolving rapidly, driven by advancements in technology that enhance our ability to analyze data, make predictions, and manage risk. Artificial intelligence (AI) plays a pivotal role in this transformation, offering powerful tools for understanding financial market trends. From predictive modeling for stock performance to sentiment analysis on financial news, AI is reshaping how we approach investment decisions.
In this blog post, we’ll explore the various ways AI in finance is being utilized, the benefits it brings, and the ethical considerations that come with its adoption. We’ll also touch on key tools like Bloomberg Terminal, FactSet, and Refinitiv, which are essential for financial professionals navigating the data-driven landscape.
The Power of Predictive Modeling for Stock Performance
One of the most promising applications of AI in finance is predictive modeling for stock performance. By leveraging machine learning algorithms, these models can analyze historical and real-time data to forecast future market movements with greater accuracy than traditional methods.
How Predictive Models Work
Predictive models use a combination of statistical techniques and advanced algorithms to identify patterns in financial data. They consider various factors such as economic indicators, company performance metrics, and market sentiment to generate forecasts. For example, an AI model might analyze quarterly earnings reports, management statements, and macroeconomic trends to predict how a stock will perform over the next quarter.
Predictive modeling also incorporates unsupervised learning techniques like clustering and anomaly detection to identify unexpected patterns or outliers in the data that could indicate potential market shifts. This multifaceted approach ensures that predictive models are not only accurate but also resilient to changing market conditions.
Benefits of Predictive Modeling
- Improved Accuracy: By analyzing vast amounts of data quickly and accurately, predictive models can identify trends that humans might miss. Traditional methods often rely on limited datasets and subjective analysis, whereas AI can sift through terabytes of information to uncover hidden patterns.
- Timely Insights: AI can process real-time data, providing timely insights into market conditions and potential opportunities. This capability is particularly valuable during volatile market periods when quick decisions can make a significant impact on investment performance.
- Risk Assessment: Predictive models can help financial institutions identify risk factors that might not be apparent through traditional methods. By forecasting potential downturns or unexpected events, AI can enable proactive risk management strategies.
Tools for Predictive Modeling
Several tools and platforms offer advanced predictive modeling capabilities for stock performance analysis:
- FactSet: Known for its comprehensive financial data coverage, FactSet provides robust predictive analytics solutions that help investors make informed decisions.
- Refinitiv Data Platform (formerly Thomson Reuters Financial & Risk): Offers advanced analytics and machine learning tools to predict market trends and inform investment strategies.
Sentiment Analysis on Financial News
Sentiment analysis is another powerful application of AI in finance, particularly for assessing the impact of news events on financial markets. By analyzing text data from news articles, social media, and other sources, sentiment analysis can gauge investor sentiment towards specific stocks or sectors.
How Sentiment Analysis Works
Sentiment analysis uses natural language processing (NLP) techniques to interpret human language. Advanced algorithms can analyze the tone and context of written content to determine whether it is positive, negative, or neutral. This information is then used to assess how market participants might react to specific news events.
For instance, an AI model could analyze a press release from a major corporation and predict its impact on stock prices based on historical data patterns associated with similar announcements in the past. Sentiment analysis can also incorporate sentiment indicators such as emojis, hashtags, and slang terms used in social media posts.
Benefits of Sentiment Analysis
- Early Indicators: Sentiment analysis can provide early signals about market trends before traditional financial metrics become available. This capability is particularly valuable for traders who need to make quick decisions based on breaking news.
- Comprehensive Coverage: By analyzing a wide range of sources, sentiment analysis provides a more comprehensive view of investor sentiment than traditional methods that rely solely on structured financial data.
- Real-Time Insights: Sentiment analysis can be performed in real-time, allowing financial professionals to respond quickly to changing market conditions.
Tools for Sentiment Analysis
Several tools and platforms offer advanced sentiment analysis capabilities:
- Bloomberg Terminal: Incorporates news analytics features that use AI to provide insights into market trends based on news sentiment.
- Refinitiv Data Platform (formerly Thomson Reuters Financial & Risk): Offers advanced NLP capabilities to analyze news articles and social media posts for sentiment analysis.
Enhancing Risk Management with AI
Risk management is a critical aspect of financial operations, and AI in finance offers powerful tools to enhance this process. By using machine learning algorithms, financial institutions can identify potential risks early and take proactive measures to mitigate them.
How AI Enhances Risk Management
AI can analyze large datasets to identify risk factors that might not be apparent through traditional methods. For example, an AI model might detect unusual patterns in credit card transactions that could indicate fraudulent behavior. Similarly, it can evaluate market conditions to assess potential investment risks.
Advanced AI techniques such as reinforcement learning can also be used to optimize risk management strategies by simulating different scenarios and identifying the most effective responses. This approach enables financial institutions to develop more resilient risk management frameworks that can adapt to changing market conditions.
Benefits of AI in Risk Management
- Early Detection: AI can detect risk factors early, allowing financial institutions to take corrective actions promptly. Traditional methods often rely on lagging indicators that may not provide sufficient time for effective risk mitigation.
- Improved Decision-Making: By providing insights into complex datasets, AI helps financial professionals make more informed decisions. This capability is particularly valuable during times of market volatility when quick and accurate decision-making can have a significant impact on investment performance.
- Cost Reduction: AI-driven risk management tools can reduce the need for manual monitoring and analysis, lowering operational costs. Automated systems can perform continuous risk assessments without the need for human intervention, freeing up financial professionals to focus on higher-value activities.
Tools for Risk Management
Several tools and platforms offer advanced risk analytics solutions:
- FactSet: Provides robust risk analytics capabilities that help financial institutions manage risk effectively. These platforms provide real-time risk assessments and support scenario planning to ensure robust risk management strategies.
- Refinitiv Data Platform (formerly Thomson Reuters Financial & Risk): Offers advanced risk assessment tools that use AI to identify potential risks and optimize risk management strategies.
Ethical Considerations in AI Adoption
As with any technology, the adoption of AI in finance raises several ethical considerations that need to be addressed. Here are some key issues:
- Bias in Algorithms: AI models can perpetuate biases present in training data if not properly designed and monitored. For example, an AI model trained on historical financial data may inadvertently incorporate gender or racial biases that could affect investment decisions.
- Transparency: The decision-making processes of AI systems can be complex and opaque, making it challenging to understand how decisions are made. This lack of transparency raises concerns about accountability and the ability to explain AI-driven decisions to stakeholders.
- Data Privacy: AI models require access to large amounts of data, including sensitive financial information. Ensuring data privacy and protecting against unauthorized access is a critical ethical consideration in the adoption of AI in finance.
Addressing Ethical Considerations
To address these ethical concerns, several strategies can be employed:
- Bias Mitigation: Implementing bias mitigation techniques during the development and deployment of AI models can help reduce the risk of perpetuating biases. This includes using diverse training datasets and regularly auditing AI systems for fairness.
- Explainability: Developing explainable AI (XAI) systems that provide transparent and understandable explanations for AI-driven decisions can improve accountability and trust in AI technologies. XAI techniques include feature importance analysis, counterfactual explanations, and model interpretability tools.
- Data Governance: Establishing robust data governance frameworks to ensure the secure handling of sensitive financial information is critical for protecting data privacy. This includes implementing data access controls, encryption, and regular audits of data management practices.
Future Trends in AI for Finance
The use of AI in finance is rapidly evolving, and several emerging trends are likely to shape its future:
- Integration with Blockchain: The integration of AI with blockchain technology can enable more secure and efficient financial transactions. AI algorithms can be used to analyze blockchain data for fraud detection, risk assessment, and portfolio optimization.
- Enhanced Personalization: Advances in AI can enable more personalized financial services tailored to individual investor preferences and needs. AI-driven recommendation systems can provide customized investment advice based on an investor’s unique circumstances.
- Regulatory Compliance: As AI is increasingly adopted in finance, regulatory compliance becomes a critical consideration. Emerging trends include the development of AI models that comply with regulatory requirements and the use of AI to automate regulatory reporting and compliance checks.
Challenges and Opportunities
While these emerging trends present significant opportunities for innovation in finance, they also pose challenges:
- Regulatory Uncertainty: The rapid pace of technological change can create uncertainty around regulatory frameworks governing AI in finance. Financial institutions need to stay informed about evolving regulations and ensure that their AI systems comply with relevant laws.
- Data Quality: The effectiveness of AI models depends on the quality and availability of data. Ensuring access to high-quality financial data is critical for developing accurate and reliable AI-driven insights.
- Technical Expertise: Implementing AI in finance requires specialized technical expertise. Financial institutions need to invest in training programs and hire skilled professionals to develop and maintain their AI systems.
Conclusion
The use of AI in finance has the potential to transform the industry by enabling more efficient, accurate, and personalized financial services. By leveraging advanced analytics, sentiment analysis, risk management, and other AI techniques, financial institutions can gain a competitive edge and better serve their clients.
However, the adoption of AI also raises ethical considerations that need to be carefully addressed. Ensuring fairness, transparency, and data privacy is critical for building trust in AI technologies and ensuring responsible innovation.
As the field of AI continues to evolve, financial institutions must stay ahead of technological advancements while navigating regulatory challenges and addressing ethical concerns. By doing so, they can unlock the full potential of AI to drive growth, improve investor outcomes, and shape the future of finance.