Building Resilient Neural Architecture Models
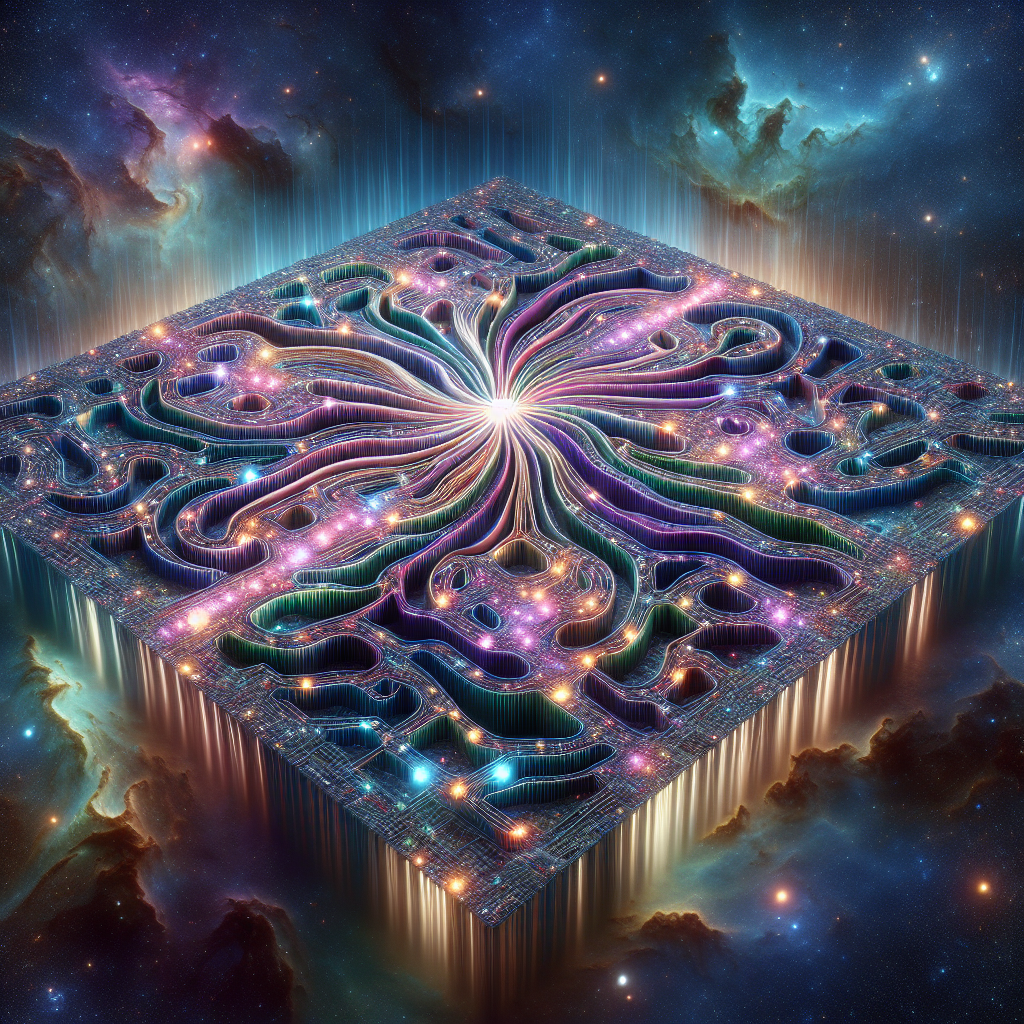
In today’s fast-paced world of artificial intelligence (AI), the demand for resilient neural networks and robust machine learning architectures has skyrocketed. As organizations across various industries strive to harness AI’s potential, they also face challenges in adapting these systems to diverse datasets and dynamic environments. This comprehensive guide explores the concept of resilient neural networks, delves into automated model design, and highlights strategies for enhancing adaptability—all crucial elements for building robust machine learning architectures.
Introduction
The rise of AI has brought about significant advancements across fields like healthcare, finance, and more. However, developing models that remain effective across different datasets and conditions remains a persistent challenge. Resilient neural networks address this issue by focusing on automated model design and optimization techniques to build systems that are not only powerful but also adaptable and robust.
Leading research organizations such as Google Brain, DeepMind, and the Massachusetts Institute of Technology (MIT) have made significant strides in this area, offering valuable insights and methodologies. In this blog post, we’ll explore these strategies and how they can be applied to create resilient neural architecture models.
Understanding Resilient Neural Networks
What Are Resilient Neural Networks?
Resilient neural networks are designed to maintain high performance despite variations in datasets or adversarial conditions. They prioritize adaptability, flexibility, and robustness, making them essential for AI systems that need to perform consistently across diverse environments.
Key Features of Resilient Neural Networks
- Adaptability: Ability to adjust to new data patterns without requiring extensive retraining.
- Flexibility: Capability to handle a variety of tasks or datasets with minimal modification.
- Robustness: Resistance to noise, errors, and adversarial attacks that could degrade performance.
Strategies for Enhancing the Adaptability of Neural Network Models
To ensure neural network models are adaptable to diverse datasets, several strategies can be employed:
Dataset Augmentation
Dataset augmentation artificially increases the size and diversity of training data by applying techniques like rotation, scaling, or adding noise. This exposure helps models generalize better and enhances their adaptability.
Transfer Learning
Transfer learning leverages pre-trained models on large datasets to fine-tune them for specific tasks with smaller datasets. This technique improves model generalization and reduces the need for extensive labeled data, enhancing adaptability in diverse contexts.
Meta-Learning
Meta-learning, or “learning to learn,” enables neural networks to quickly adapt to new tasks by learning from a variety of previous experiences. It allows models to build generalized knowledge that can be applied across different domains, improving their adaptability and robustness.
Techniques for Automating Optimization and Training Processes
Techniques for automating optimization and training processes are pivotal in developing artificial intelligence systems efficiently:
Neural Architecture Search (NAS)
Neural Architecture Search (NAS) is an automated approach to designing neural network architectures. By exploring a vast configuration space, NAS reduces manual effort and enhances performance by discovering optimal architecture designs.
Example: EfficientNet
EfficientNet is a result of applying NAS to optimize model scaling. It has achieved state-of-the-art accuracy with significantly fewer computational resources compared to traditional models, showcasing the power of automated design in achieving efficient and robust architectures.
Hyperparameter Optimization
Hyperparameter optimization involves fine-tuning the parameters that govern model training. Automated techniques like Bayesian optimization or grid search ensure that the best possible configurations are discovered efficiently, leading to more effective neural networks.
Case Study: Google’s AutoML
Google’s AutoML uses hyperparameter optimization as part of its suite to help users develop custom machine learning models with minimal expertise required. It demonstrates how automation can democratize AI development and enhance model performance across various applications.
Role of Reinforcement Learning in Model Development
Reinforcement learning (RL) techniques play a crucial role in neural network training, particularly in areas where decision-making is involved:
Architecture Search through RL
Reinforcement learning algorithms are employed to optimize neural architectures by treating the design process as a sequential decision-making problem. This approach allows for the exploration of vast architectural spaces, identifying configurations that yield optimal performance.
Application: AlphaGo and Neural Network Architectures
AlphaGo’s success in mastering complex games like Go was partly due to innovative RL techniques applied not only during gameplay but also in refining its neural network architecture. Similar methods can be adapted for optimizing AI models across various domains.
Policy Gradient Methods
Policy gradient methods within reinforcement learning are used to directly optimize the policy that determines model behavior, facilitating better adaptation to new environments and tasks. This approach is particularly useful when traditional gradient-based optimization falls short due to non-differentiable elements in the process.
Real-World Applications of Resilient Neural Networks
Resilient neural networks have transformative applications across multiple sectors:
Healthcare
In healthcare, resilient neural networks enable personalized medicine by adapting models to individual patient data. They are used for predictive diagnostics, improving treatment plans, and monitoring chronic diseases more effectively.
Example: IBM Watson Health
IBM’s Watson Health employs AI-driven analytics that adapt to new medical research findings and patient information, providing clinicians with insights that enhance decision-making processes in real-time.
Autonomous Vehicles
Autonomous vehicles rely on resilient neural networks to navigate complex environments. These networks must continuously adapt to varying road conditions, weather changes, and unexpected obstacles, ensuring safety and reliability.
Case Study: Tesla Autopilot
Tesla’s Autopilot system utilizes advanced neural networks that learn from vast amounts of driving data, improving its ability to handle diverse driving scenarios safely and efficiently.
Financial Services
In financial services, resilient neural networks enhance fraud detection systems by adapting to new patterns of fraudulent behavior. They also support algorithmic trading strategies that require rapid adaptation to market conditions.
Application: JPMorgan’s COIN
JPMorgan’s Contract Intelligence (COIN) platform uses machine learning algorithms to interpret commercial loan agreements quickly and accurately, reducing the time and errors associated with manual processing.
The Future of Resilient Neural Networks
As AI continues to evolve, resilient neural networks will play an increasingly critical role in driving innovation across industries. Ongoing research focuses on improving their adaptability, scalability, and efficiency:
Quantum Computing Integration
Future advancements may involve integrating quantum computing techniques with neural network architectures, potentially revolutionizing processing power and model capabilities.
Explainable AI (XAI)
The development of explainable AI approaches aims to make resilient neural networks more transparent, fostering trust and allowing for better understanding and refinement of their decision-making processes.
Conclusion
Building resilient neural architecture models is essential in today’s rapidly advancing technological landscape. By leveraging strategies such as dataset augmentation, transfer learning, meta-learning, and automated optimization techniques like NAS and hyperparameter tuning, we can develop AI systems that are adaptable, efficient, and robust. Reinforcement learning further enhances these capabilities by optimizing model architectures and policies.
As resilient neural networks continue to find applications in healthcare, autonomous vehicles, financial services, and beyond, their transformative impact on industries is undeniable. The future of AI will be shaped by ongoing research into making these models more adaptable, scalable, and efficient, ensuring they meet the ever-growing demands of diverse datasets and dynamic environments.
Ready to Transform Your Business with AI?
Are you looking to build resilient neural networks that adapt to diverse datasets and dynamic environments? Our AI Agentic software development and AI Cloud Agents services are designed to help you achieve this goal. By leveraging automated model design and robust machine learning architectures, we’ve empowered companies across various industries to implement cutting-edge solutions.
We understand the challenges of adapting AI models to real-world applications, which is why our team specializes in providing tailored strategies that enhance adaptability and resilience. Whether through dataset augmentation, transfer learning, or advanced optimization techniques like Neural Architecture Search, we can help you build powerful AI systems delivering consistent performance across diverse scenarios.
Contact us today for a consultation to explore how we can support your AI initiatives. Visit our website [insert link] to learn more about our services and see how we’ve helped other organizations succeed with their AI projects. Let’s work together to transform your business with innovative, resilient AI solutions.