Exploring BERT’s Impact on Natural Language Processing
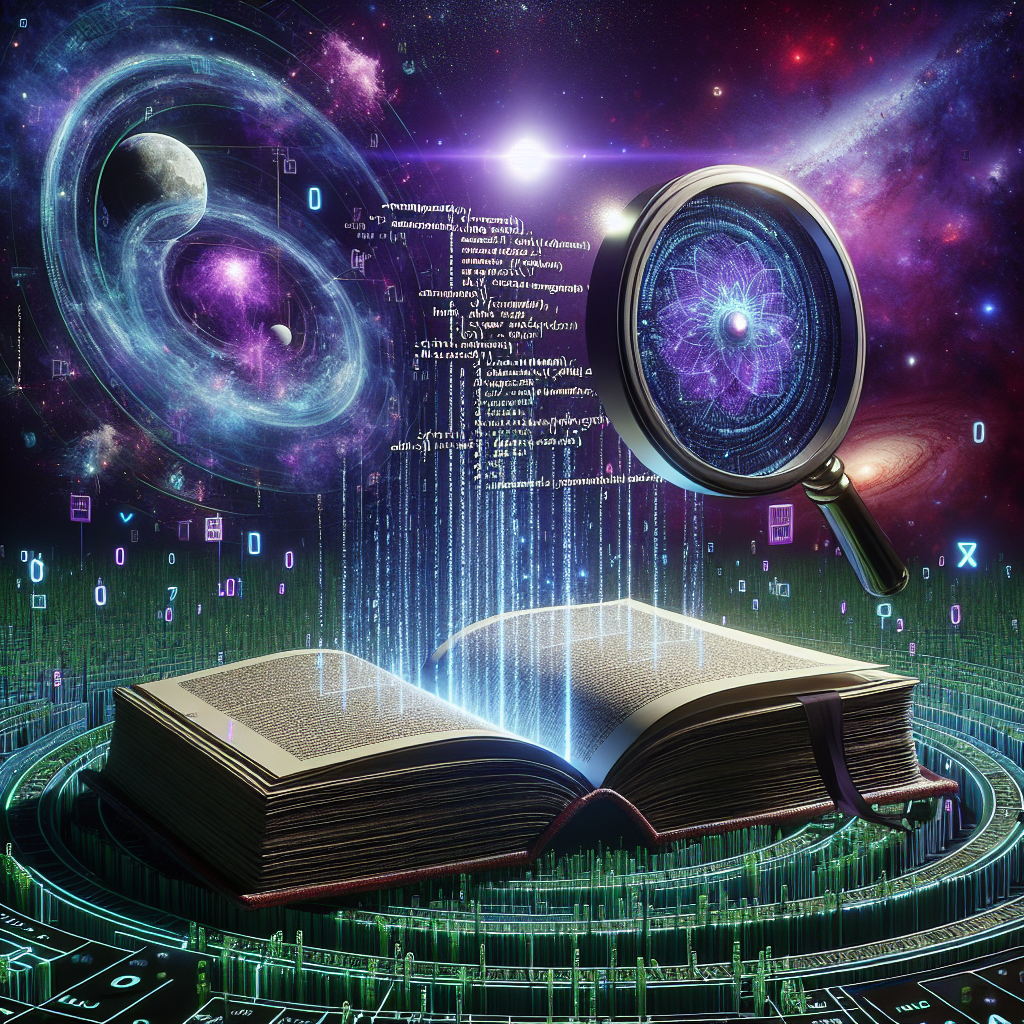
Exploring BERT’s Impact on Natural Language Processing
Introduction
Natural Language Processing (NLP) has undergone a revolutionary transformation with the advent of pre-trained transformer models like BERT. Developed by Google AI Research, BERT—Bidirectional Encoder Representations from Transformers—has significantly altered how machines understand and process human language. By harnessing deep learning techniques, BERT boosts context-awareness in language models, facilitating more precise semantic analysis and comprehension.
In this comprehensive exploration, we delve into the multifaceted impact of BERT on NLP. We will examine its pivotal role in advancing text understanding and its applications across various domains. Join us as we uncover how BERT has revolutionized semantic analysis, improved contextual interpretation, and paved the way for innovative deep learning NLP applications.
The Genesis of BERT
Understanding Pre-trained Transformer Models
The emergence of BERT as a groundbreaking model is deeply rooted in the evolution of transformer models, which have redefined natural language processing. Unlike traditional sequential models that process words one at a time, transformers can handle multiple words simultaneously. This ability allows them to grasp context more effectively. Introduced by Google AI Research and inspired by earlier work from institutions like Stanford University and OpenAI, BERT builds on this transformative foundation.
Bidirectional Training
One of BERT’s distinctive features is its bidirectional training approach. Traditional language models processed text in a single direction—either left-to-right or right-to-left—but not both simultaneously. BERT overcomes this limitation by considering the context from both directions at once, providing a deeper understanding of word meanings based on surrounding text.
How BERT Enhances Context-awareness
Deep Learning and Language Understanding
BERT employs advanced deep learning techniques to enhance its ability to understand language nuances. By training on vast amounts of data using unsupervised methods, BERT can capture intricate patterns in human language. This process involves predicting masked words within sentences, enabling the model to learn contextual relationships between words more effectively.
Contextual Word Embeddings
Unlike earlier models that generated static word embeddings, BERT produces dynamic representations that change depending on context. For instance, the word “bank” will have different meanings based on whether it appears in a financial or river-related sentence. This capability is crucial for improving text understanding across various applications and highlights the role of BERT in advancing semantic analysis and comprehension.
The Role of BERT in Advancing Semantic Analysis
Enhanced Comprehension through Attention Mechanisms
BERT’s architecture utilizes attention mechanisms that allow it to focus on relevant parts of the input data, thereby enhancing its semantic analysis capabilities. These mechanisms enable BERT to weigh different words’ importance dynamically, leading to more accurate language comprehension.
Applications in Sentiment Analysis and Question Answering
The advancements brought by BERT have been particularly impactful in areas like sentiment analysis and question answering. By improving text understanding, BERT helps machines accurately gauge the sentiment behind customer reviews or swiftly provide precise answers to complex queries, demonstrating its profound impact on natural language processing advancements.
Real-World Applications of BERT
Enhancing User Experience in Digital Platforms
BERT is utilized across various industries for tasks such as sentiment analysis, question answering, machine translation, fraud detection, and healthcare analytics. By enhancing text understanding and decision-making processes, BERT significantly improves user experiences on digital platforms. For instance, it can power intelligent chatbots that provide instant support to users, making interactions more seamless and efficient.
Driving Innovation in Healthcare Analytics
In the healthcare sector, BERT aids in analyzing patient records and medical literature, enabling faster and more accurate diagnosis and treatment recommendations. This application of BERT not only enhances patient care but also streamlines operational workflows within healthcare institutions.
Case Study: Google Search
One prominent example is how BERT has been integrated into Google’s search algorithms to better understand user queries. By interpreting the context of search terms, BERT enables more relevant and precise search results, significantly enhancing users’ online research experiences.
Challenges and Limitations of BERT
While BERT represents a significant leap forward in NLP, it is not without its challenges. The model requires substantial computational resources for training and deployment, which can be a barrier for some organizations. Additionally, while BERT’s understanding of language has improved, it still struggles with certain nuances, such as sarcasm or context-dependent meanings that require world knowledge.
Future Directions in NLP
Looking ahead, the future of NLP promises even greater advancements. Researchers are exploring ways to make models like BERT more efficient and accessible. One area of focus is on developing smaller models that retain BERT’s capabilities but with reduced computational demands. Additionally, integrating multimodal data—combining text with images or audio—could further enhance context-awareness in language processing.
Conclusion
BERT has undeniably transformed the landscape of natural language processing by significantly improving how machines interpret human language. Its ability to provide context-rich interpretations and its versatility across different applications have made it a cornerstone in NLP advancements. As technology continues to evolve, BERT’s influence will likely expand, opening new possibilities for machine understanding and interaction with human language.
Join us as we continue exploring these exciting developments and their implications for industries worldwide. Stay informed about the ongoing evolution of NLP technologies and discover how they are reshaping our interaction with digital systems.